Collaborative Robot-Assisted Endovascular Catheterization with Generative Adversarial Imitation Learning.
ICRA(2020)
摘要
Master-slave systems for endovascular catheterization have brought major clinical benefits including reduced radiation doses to the operators, improved precision and stability of the instruments, as well as reduced procedural duration. Emerging deep reinforcement learning (RL) technologies could potentially automate more complex endovascular tasks with enhanced success rates, more consistent motion and reduced fatigue and cognitive workload of the operators. However, the complexity of the pulsatile flows within the vasculature and non-linear behavior of the instruments hinder the use of model-based approaches for RL. This paper describes model-free generative adversarial imitation learning to automate a standard arterial catherization task. The automation policies have been trained in a pre-clinical setting. Detailed validation results show high success rates after skill transfer to a different vascular anatomical model. The quality of the catheter motions also shows less mean and maximum contact forces compared to manual-based approaches.
更多查看译文
关键词
reduced procedural duration,deep reinforcement learning technologies,complex endovascular tasks,reduced fatigue,cognitive workload,model-based approaches,model-free generative adversarial imitation learning,standard arterial catherization task,automation policies,catheter motions,collaborative robot-assisted endovascular catheterization,master-slave systems,clinical benefits,radiation doses,vascular anatomical model
AI 理解论文
溯源树
样例
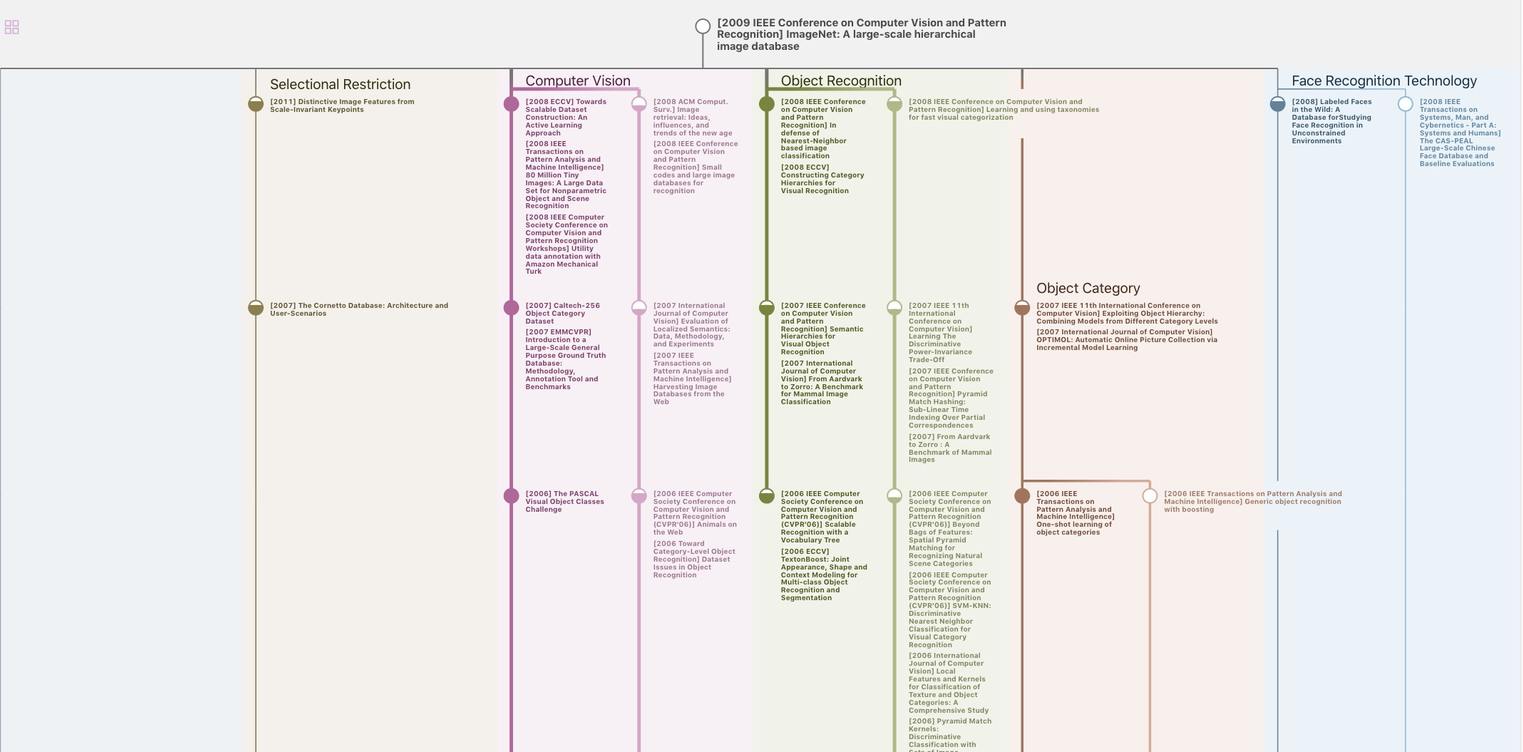
生成溯源树,研究论文发展脉络
Chat Paper
正在生成论文摘要