Probabilistic TSDF Fusion Using Bayesian Deep Learning for Dense 3D Reconstruction with a Single RGB Camera.
ICRA(2020)
摘要
In this paper, we address a 3D reconstruction problem using depth prediction from a single RGB image. With the recent advances in deep learning, depth prediction shows high performance. However, due to the discrepancy between training environment and test environment, 3D reconstruction can be vulnerable to the uncertainty of depth prediction. To consider the uncertainty of depth prediction for robust 3D reconstruction, we adopt Bayesian deep learning framework. Conventional Bayesian deep learning requires a large amount of time and GPU memory to perform Monte Carlo sampling. To address this problem, we propose a lightweight Bayesian neural network consisting of U-net structure and summation-based skip connections, which is performed in real-time. Estimated uncertainty is utilized in probabilistic TSDF fusion for dense 3D reconstruction by maximizing the posterior of TSDF value per voxel. As a result, global TSDF robust to erroneous depth values can be obtained and then dense 3D reconstruction from the global TSDF is achievable more accurately. To evaluate the performance of depth prediction and 3D reconstruction using our method, we utilized two official datasets and demonstrated the outperformance of the proposed method over other conventional methods.
更多查看译文
关键词
probabilistic tsdf fusion,bayesian deep learning,3d reconstruction,single rgb camera
AI 理解论文
溯源树
样例
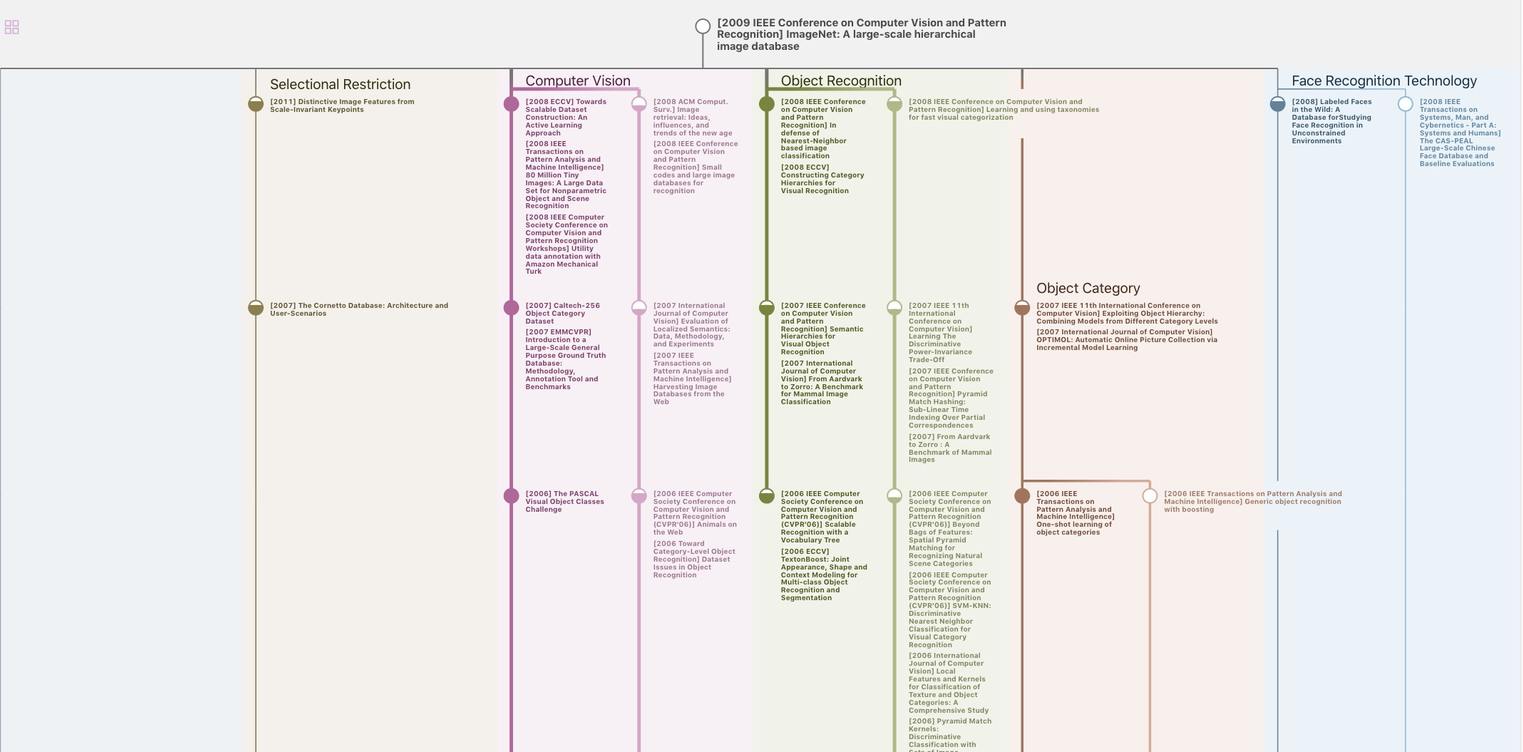
生成溯源树,研究论文发展脉络
Chat Paper
正在生成论文摘要