Scad-Penalized Complex Gaussian Graphical Model Selection
2020 IEEE 30th International Workshop on Machine Learning for Signal Processing (MLSP)(2020)
摘要
We consider the problem of estimating the conditional independence graph (CIG) of a sparse, high-dimensional proper complex-valued Gaussian graphical model (CGGM). For CGGMs, the problem reduces to estimation of the inverse covariance matrix with more unknowns than the sample size. We consider a smoothly clipped absolute deviation (SCAD) penalty instead of the £1-penalty to regularize the problem, and analyze a SCAD-penalized log-likelihood based objective function to establish consistency and sparsistency of a local estimator of inverse covariance in a neighborhood of the true value. A numerical example is presented to illustrate the advantage of SCAD-penalty over the usual £1-penalty.
更多查看译文
关键词
Complex Gaussian graphical models,undirected graph,SCAD penalty,consistency,sparsistency
AI 理解论文
溯源树
样例
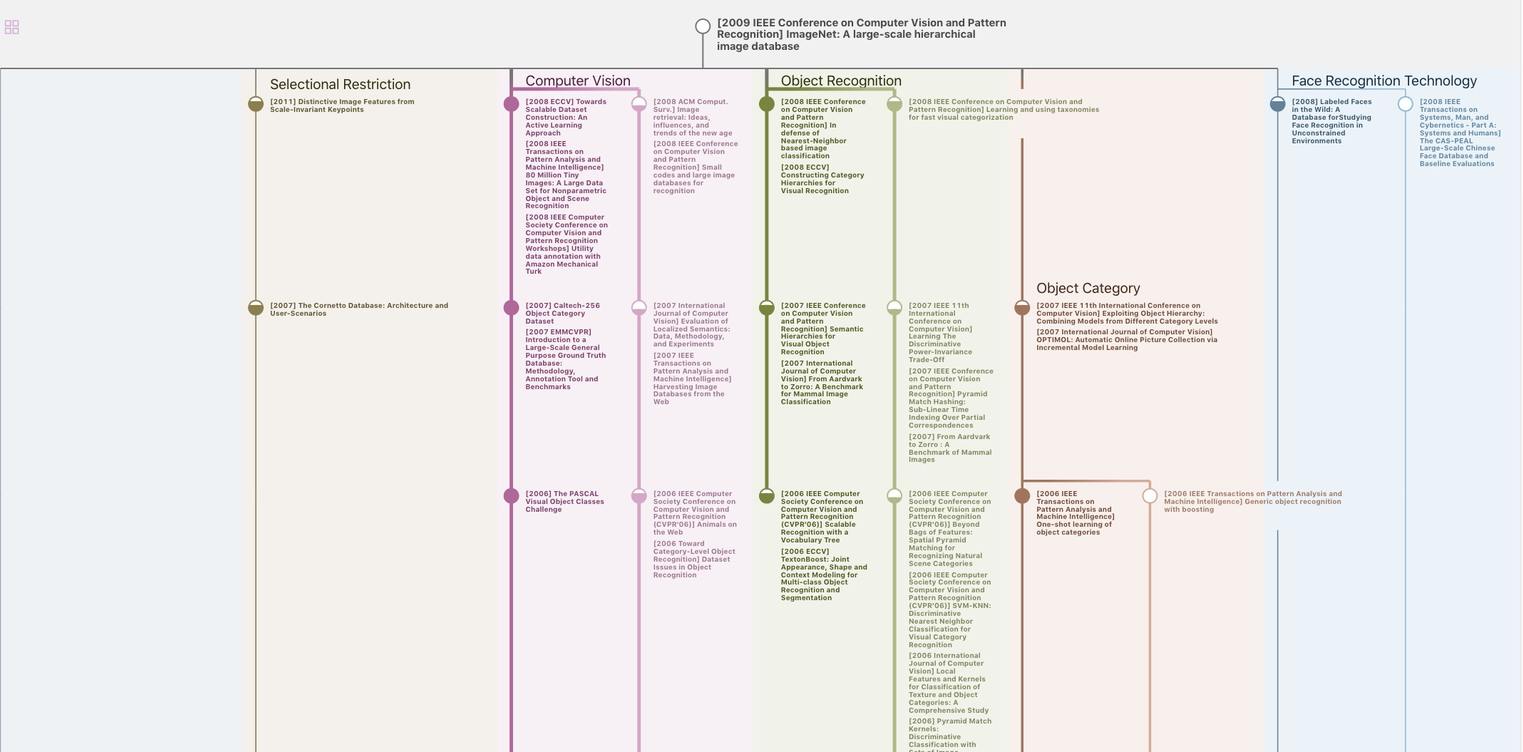
生成溯源树,研究论文发展脉络
Chat Paper
正在生成论文摘要