Model Stealing Defense with Hybrid Fuzzy Models: Work-in-Progress
2020 International Conference on Hardware/Software Codesign and System Synthesis (CODES+ISSS)(2020)
摘要
With increasing applications of Deep Neural Networks (DNNs) to edge computing systems, security issues have received more attentions. Particularly, model stealing attack is one of the biggest challenge to the privacy of models. To defend against model stealing attack, we propose a novel protection architecture with fuzzy models. Each fuzzy model is designed to generate wrong predictions corresponding to a particular category. In addition' we design a special voting strategy to eliminate the systemic errors, which can destroy the dark knowledge in predictions at the same time. Preliminary experiments show that our method substantially decreases the clone model's accuracy (up to 20%) without loss of inference accuracy for benign users.
更多查看译文
关键词
model stealing defense,hybrid fuzzy models,deep neural networks,security issues,model stealing attack,fuzzy model,systemic errors,clone model,DNN
AI 理解论文
溯源树
样例
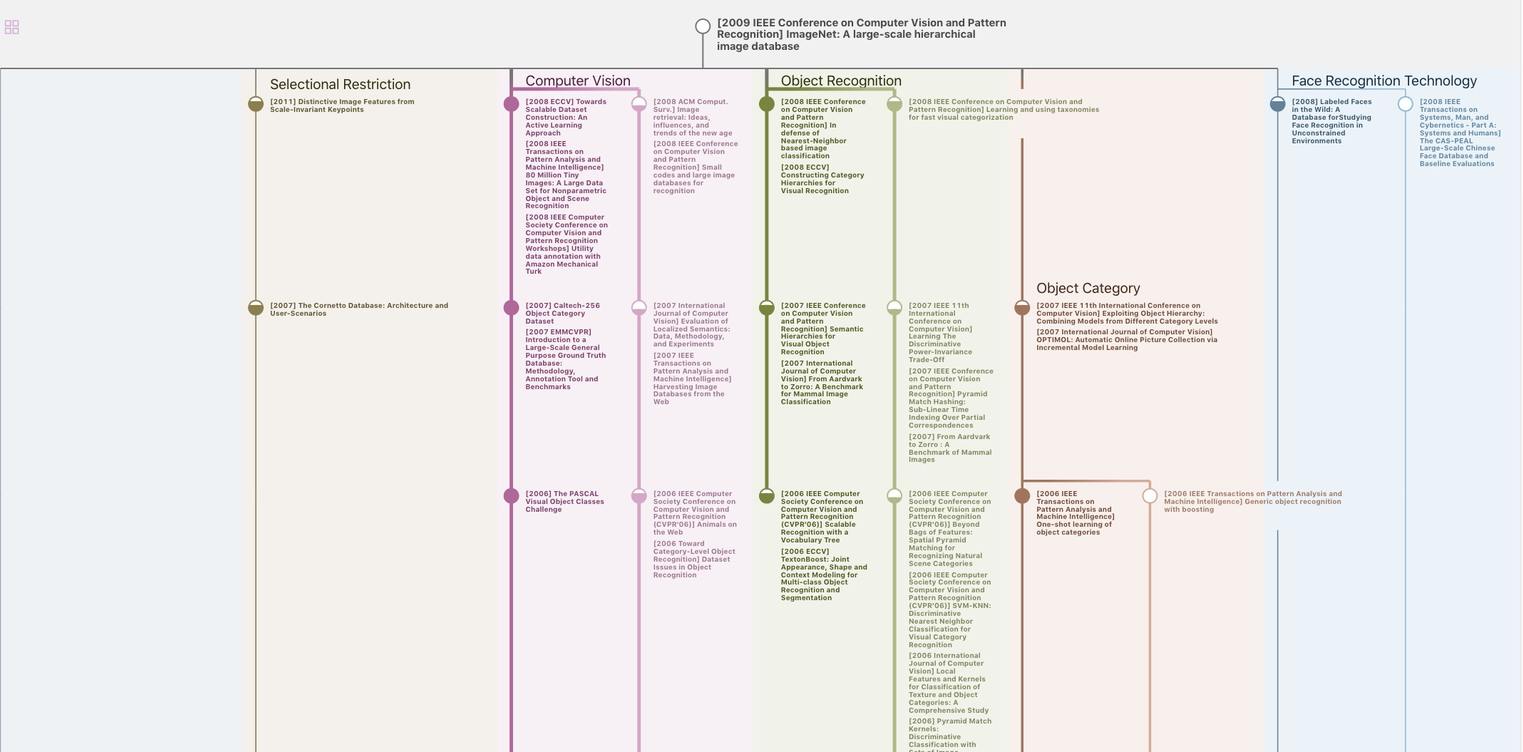
生成溯源树,研究论文发展脉络
Chat Paper
正在生成论文摘要