Sepsis Deterioration Prediction Using Channelled Long Short-Term Memory Networks.
AIME(2020)
摘要
Sepsis is a severe medical condition that results in millions of deaths globally each year. In this paper, we propose a Channelled Long-Short Term Memory Network model tasked with predicting 48-hour mortality in sepsis against the Sequential Organ Failure Assessment (SOFA) score. We use the MIMIC-III critical care database. Our research demonstrates the viability of deep learning in predicting patient outcomes in sepsis. When compared with published literature for similar tasks, our channelled LSTM models demonstrated a comparable AUROC with superior precision score. The results showed that deep learning models outperformed the SOFA score in predicting 48-hour mortality in sepsis in AUROC (0.846–0.896 vs 0.696) and average precision score (0.299–0.485 vs 0.110). Finally, our Fully-Channelled LSTM outperforms a baseline LSTM by \\(5.4\\%\\) in AUROC and \\(59.9\\%\\) in average precision score.
更多查看译文
关键词
Sepsis, Machine learning, Deep learning, Recurrent neural networks, Long short-term memory networks
AI 理解论文
溯源树
样例
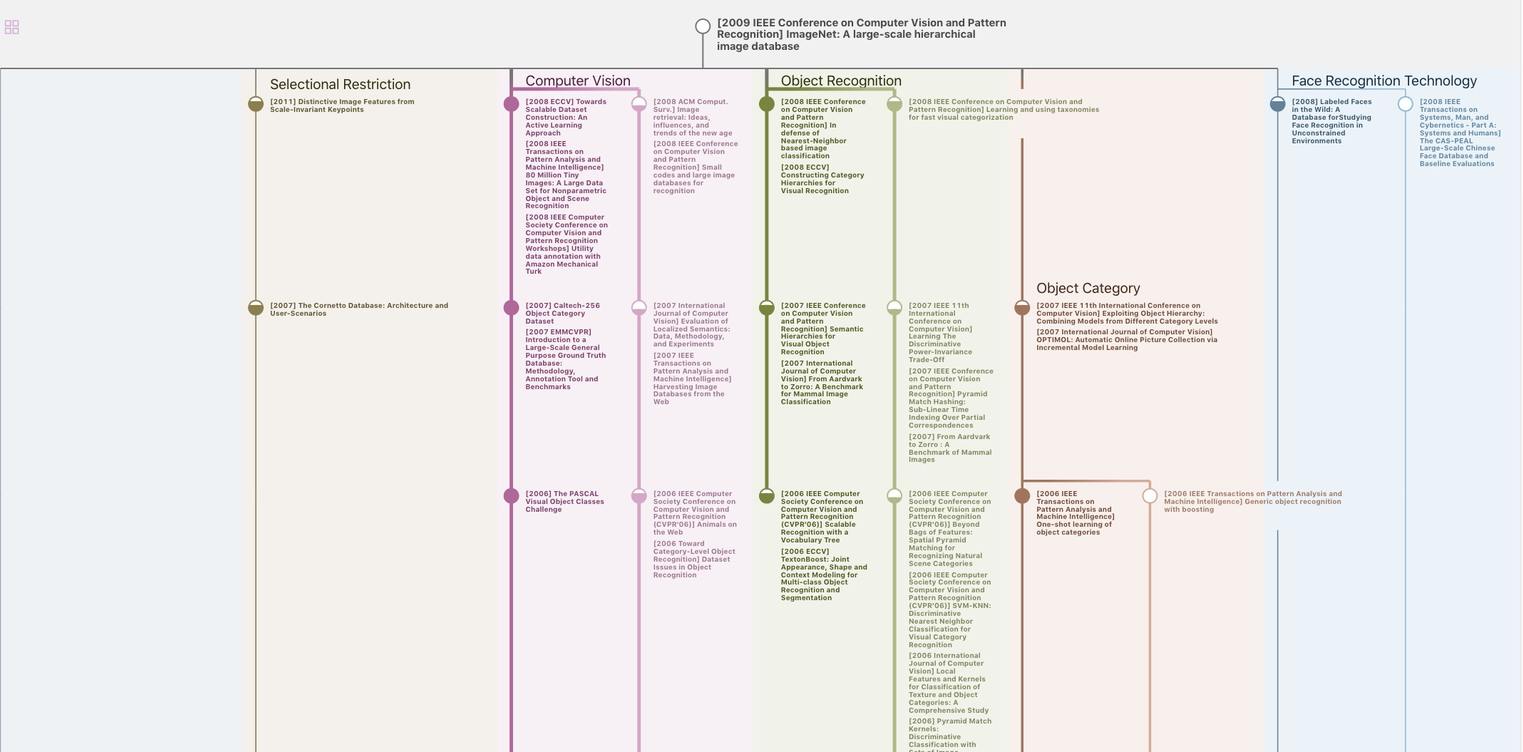
生成溯源树,研究论文发展脉络
Chat Paper
正在生成论文摘要