Are you on Mobile or Desktop? On the Impact of End-User Device on Web QoE Inference from Encrypted Traffic
2020 16th International Conference on Network and Service Management (CNSM)(2020)
摘要
Web browsing is one of the key applications of the Internet, if not the most important one. We address the problem of Web Quality-of-Experience (QoE) monitoring from the ISP perspective, relying on in-network, passive measurements. As a proxy to Web QoE, we focus on the analysis of the well-known SpeedIndex (SI) metric. Given the lack of application-level-data visibility introduced by the wide adoption of end-to-end encryption, we resort to machine-learning models to infer the SI and the QoE level of individual web-page loading sessions, using as input only packet- and flow-level data. In this paper, we study the impact of different end-user device types (e.g., smartphone, desktop, tablet) on the performance of such models. Empirical evaluations on a large, multi-device, heterogeneous corpus of Web-QoE measurements for the most popular websites demonstrate that the proposed solution can infer the SI as well as estimate QoE ranges with high accuracy, using either packet-level or flow-level measurements. In addition, we show that the device type adds a strong bias to the feasibility of these Web-QoE models, putting into question the applicability of previously conceived approaches on single-device measurements. To improve the state of the art, we conceive cross-device generalizable models operating at both packet and flow levels, offering a feasible solution for Web-QoE monitoring in operational, multi-device networks. To the best of our knowledge, this is the first study tackling the analysis of Web QoE from encrypted network traffic in multi-device scenarios.
更多查看译文
关键词
Web QoE,Smartphone vs. Desktop,Network Monitoring,Machine Learning,SpeedIndex,Encrypted Traffic
AI 理解论文
溯源树
样例
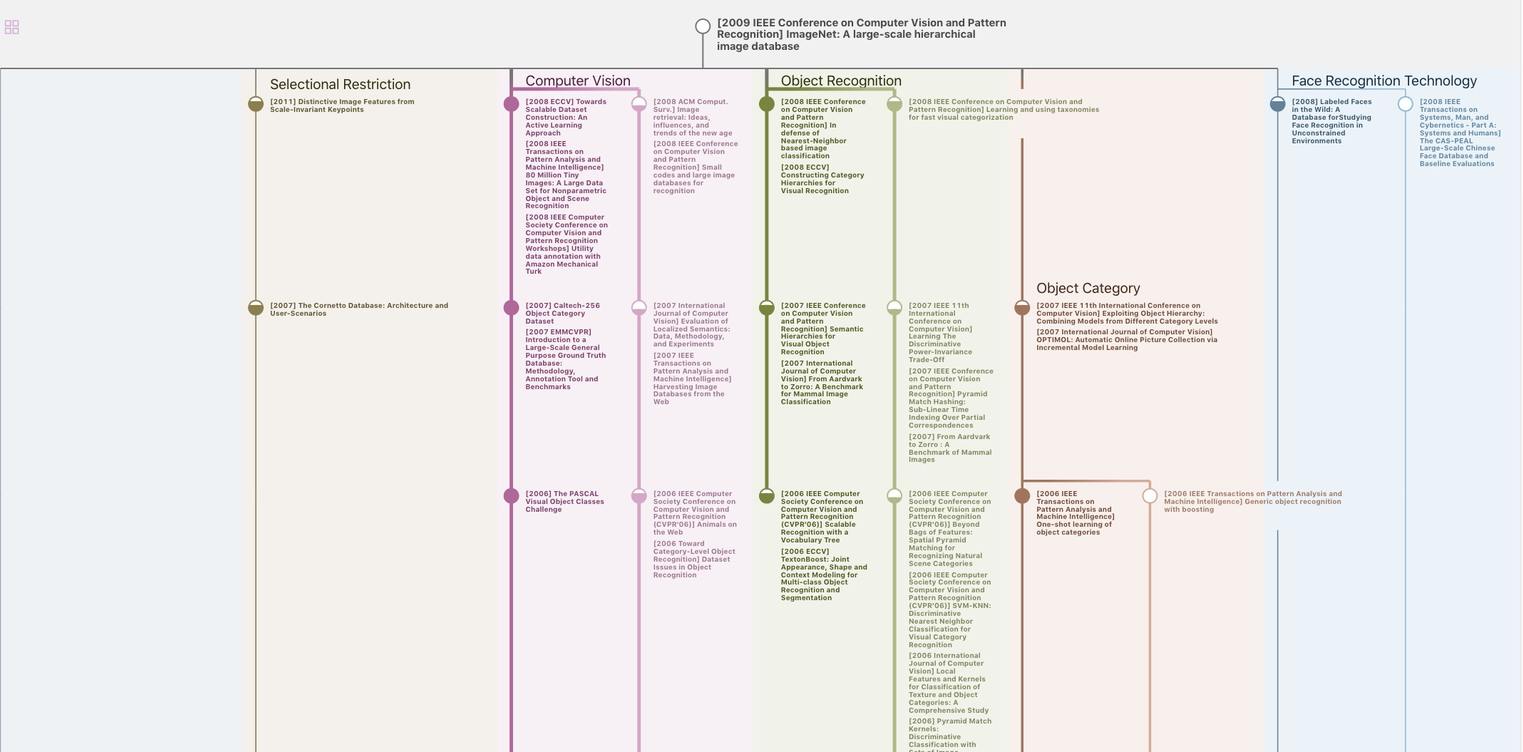
生成溯源树,研究论文发展脉络
Chat Paper
正在生成论文摘要