Sampled Angles In High-Dimensional Spaces
SIMILARITY SEARCH AND APPLICATIONS, SISAP 2020(2020)
摘要
Similarity search using metric indexing techniques is largely a solved problem in low-dimensional spaces. However techniques based only on the triangle inequality property start to fail as dimensionality increases.Since proper metric spaces allow a finite projection of any three objects into a 2D Euclidean space, the notion of angle can be validly applied among any three (but no more) objects. High dimensionality is known to have interesting effects on angles in vector spaces, but to our knowledge this has not been studied in more general metric spaces. Here, we consider the use of angles among objects in combination with distances.As dimensionality becomes higher, we show that the variance in sampled angles reduces. Furthermore, sampled angles also become correlated with inter-object distances, giving different distributions between query solutions and non-solutions. We show the theoretical underpinnings of this observation in unbounded high-dimensional Euclidean spaces, and then examine how the pure property is reflected in some real-world high dimensional spaces. Our experiments on both generated and real world datasets demonstrate that these observations can have an important impact on the tractability of search as dimensionality increases.
更多查看译文
关键词
Metric search, High dimensional space
AI 理解论文
溯源树
样例
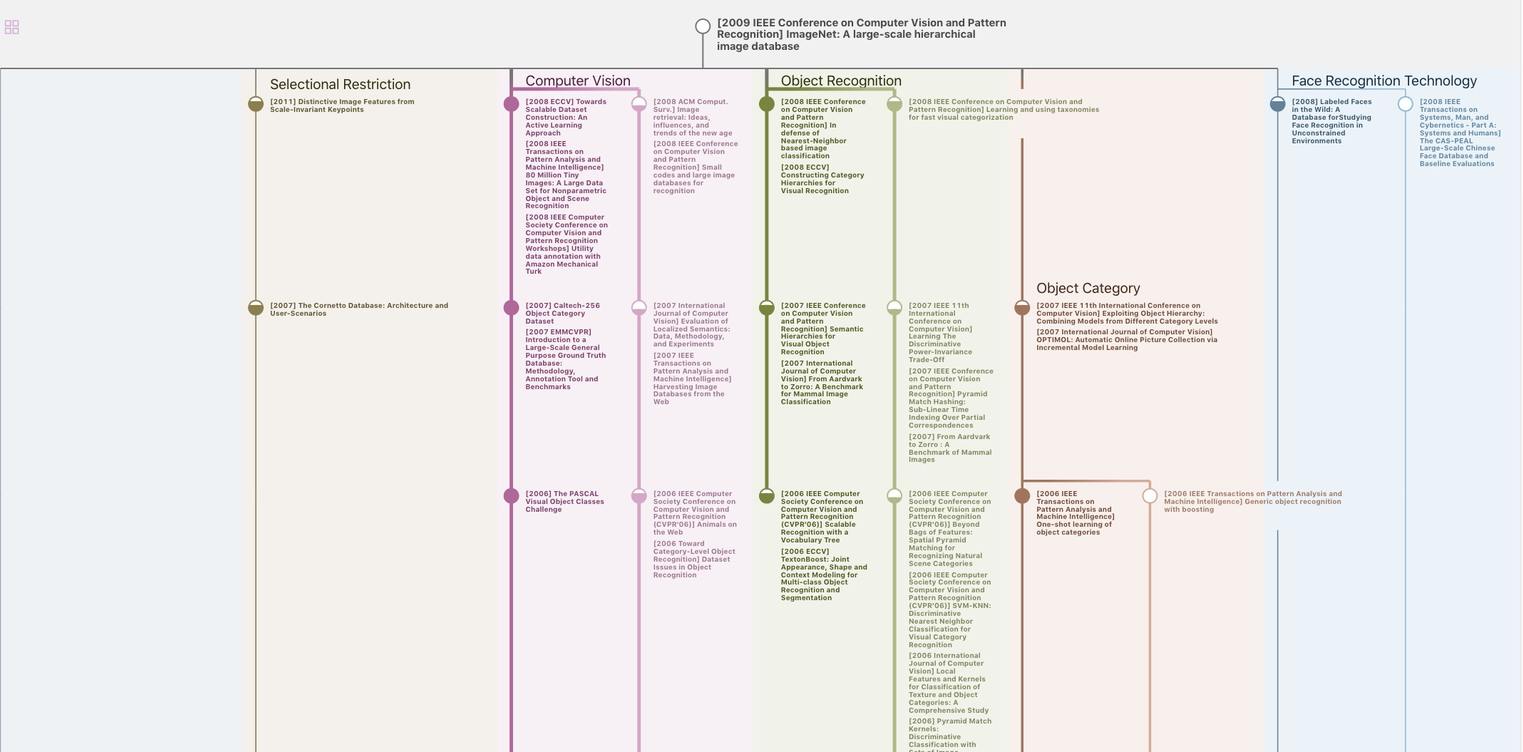
生成溯源树,研究论文发展脉络
Chat Paper
正在生成论文摘要