Template-Oriented Multi-task Sparse Low-Rank Learning for Parkinson's Diseases Diagnosis.
PRIME@MICCAI(2020)
摘要
Parkinson’s disease (PD) is a long-term degenerative disorder of the central nervous system. Early diagnosis of PD has great clinical significance as patients would be able to receive specialized treatment earlier to slow down the PD progression. Many researchers proposed various machine learning methods to classify the different stages of PD using magnetic resonance imaging (MRI). However, these methods usually extract features from MRI only using a single template. In this paper, we propose a new template-oriented multi-task sparse low-rank learning (TMSLRL) method using MRI for multi-classification of PD patients. Firstly, we extract features from MRI using different templates where each template is corresponding to a particular task. These tasks form a template-oriented multi-task learning to concurrently obtain an inner relationship of each task. Secondly, sparse low-rank learning is performed to capture the potential relationships between the inputs and the outputs and select the most class-discriminative features. Finally, we feed the selected features to train the classifier to get the final classification result. Our proposed model is evaluated by the data from the Parkinson’s progression markers initiative (PPMI) dataset. Furthermore, the results of experiments we performed indicate our method have greater performance than the similar methods.
更多查看译文
关键词
parkinsons,learning,diagnosis,template-oriented,multi-task,low-rank
AI 理解论文
溯源树
样例
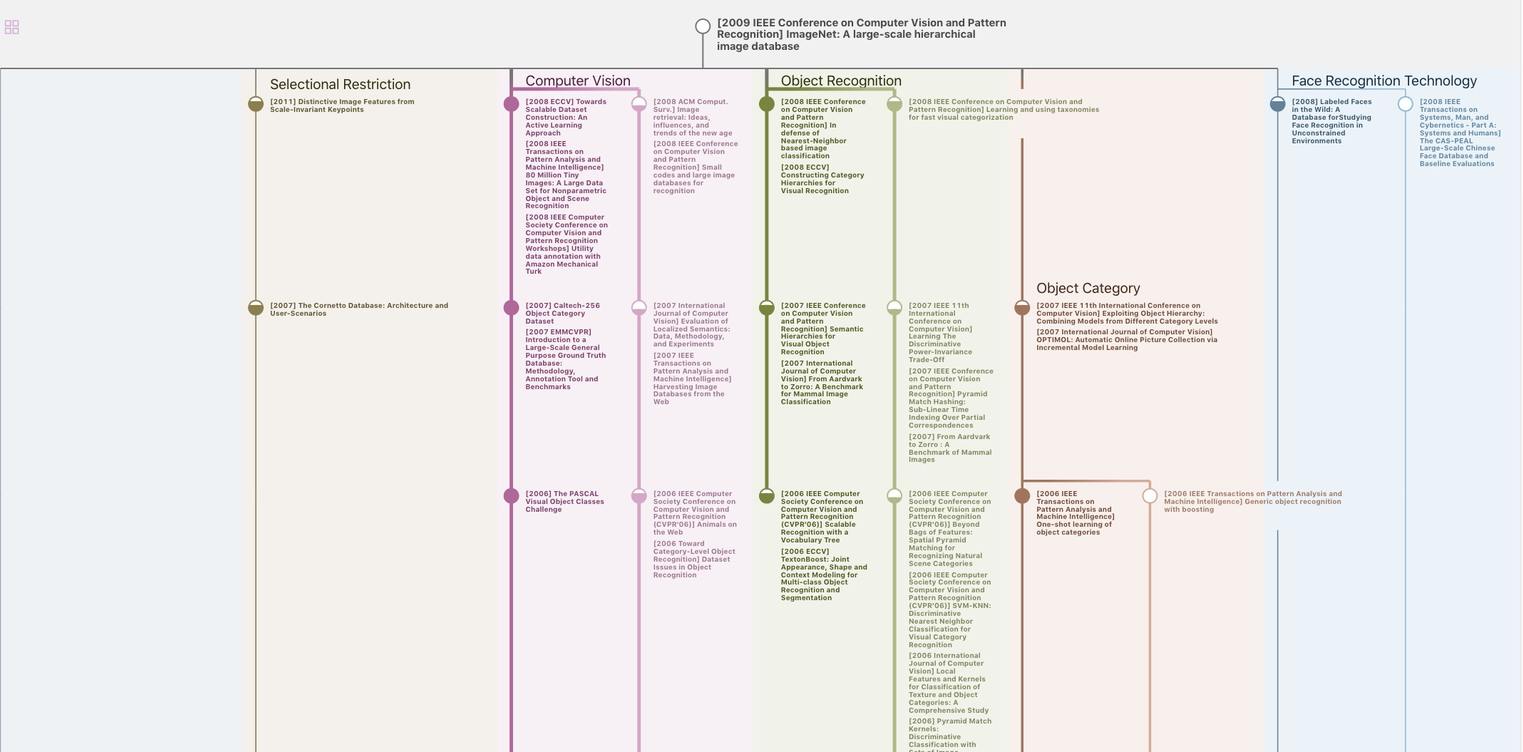
生成溯源树,研究论文发展脉络
Chat Paper
正在生成论文摘要