Dynamic Multi Objective Particle Swarm optimization with Cooperative Agents
CEC(2020)
摘要
Dynamic Multi-objective optimization problems (DMOPs) involve multiple objectives, constraints, and parameters that may change over time. For solving such types of problems, the conventional particle swarm optimization algorithm should not only be able to evolve near-optimal and diverse optimal solutions but also continually track the time-changing environment. To address these challenges, we propose a novel dynamic multiobjective particle swarm optimization approach with cooperative agents. In this strategy, the ability of tracking based on particle memory and multiple populations with sharing knowledge are combined to deal with environmental changing. If a change is detected, solutions with no improvement are re-evaluated and the worst solutions are replaced with the newly generated one. In addition, a movement strategy based on the sharing of the best knowledge is introduced to promote the population diversity. Experiments on several optimization problems are carried out to prove the performance of the proposed algorithm and the statistical results show that the proposed algorithm performs well with DMOPs.
更多查看译文
关键词
optimization, particle swarm optimization, dynamic multi-objective optimization, multi-agent system
AI 理解论文
溯源树
样例
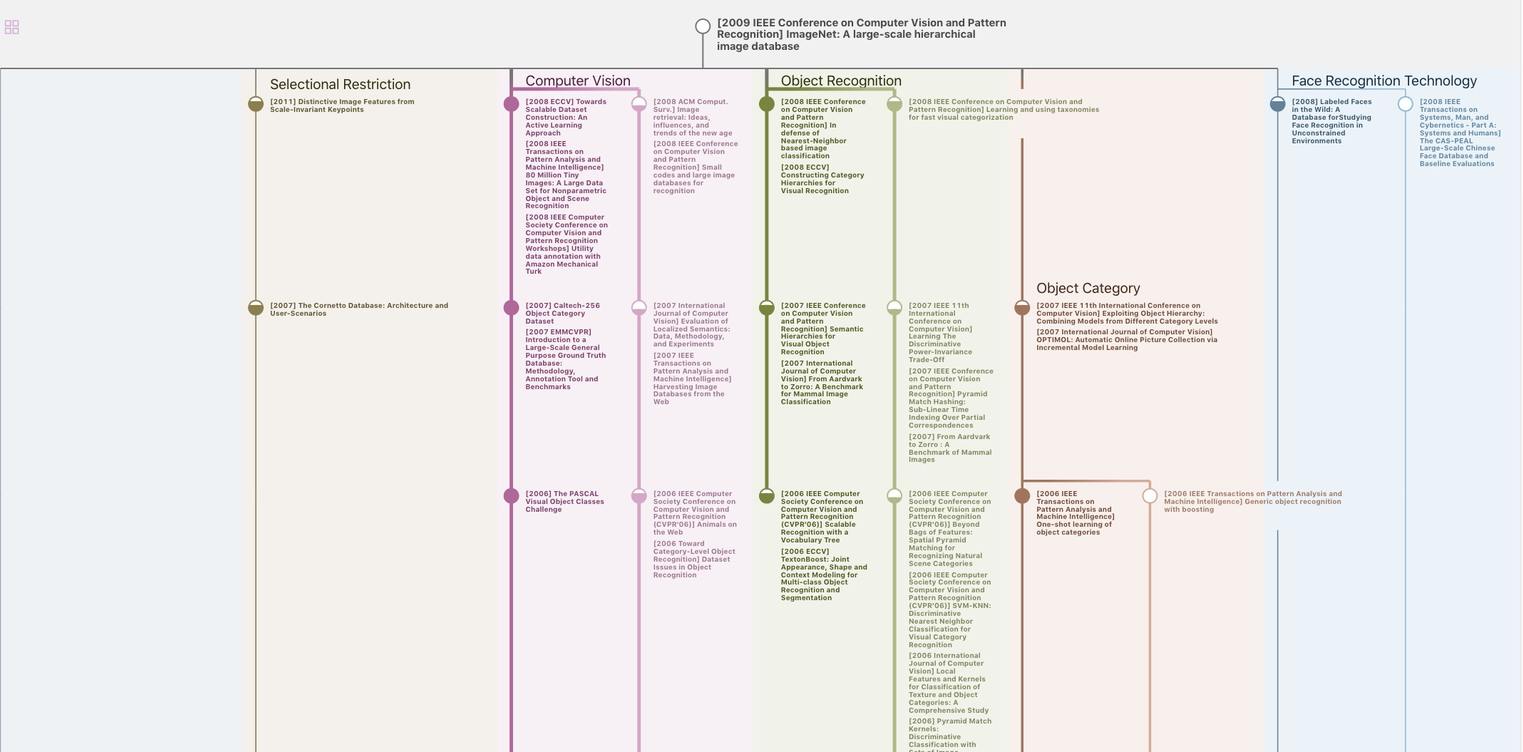
生成溯源树,研究论文发展脉络
Chat Paper
正在生成论文摘要