Performance Evaluation of Selected 3D Keypoint Detector-Descriptor Combinations.
ICCVG(2020)
摘要
Nowadays, with easily accessible 3D point cloud acquisition tools, the field of point cloud processing gained a lot of attention. Extracting features from 3D data became main computer vision task. In this paper, we reviewed methods of extracting local features from objects represented by point clouds. The goal of the work was to make theoretical overview and evaluation of selected point cloud detectors and descriptors. We performed an experimental assessment of the repeatability and computational efficiency of individual methods using the well known Stanford 3D Scanning Repository database with the aim of identifying a method which is computationally-efficient in finding good corresponding points between two point clouds. We combine the detectors with several feature descriptors and show which combination of detector and descriptor is suitable for object recognition task in cluttered scenes. Our tests show that choosing the right detector impacts the descriptor’s performance in the recognition process. The repeatability tests of the detectors show that the data which contained occlusions have a high impact on their performance. We summarized the results into graphs and described them with respect to the individual tested properties of the methods.
更多查看译文
关键词
3D detector, 3D descriptor, Point cloud, Feature extraction
AI 理解论文
溯源树
样例
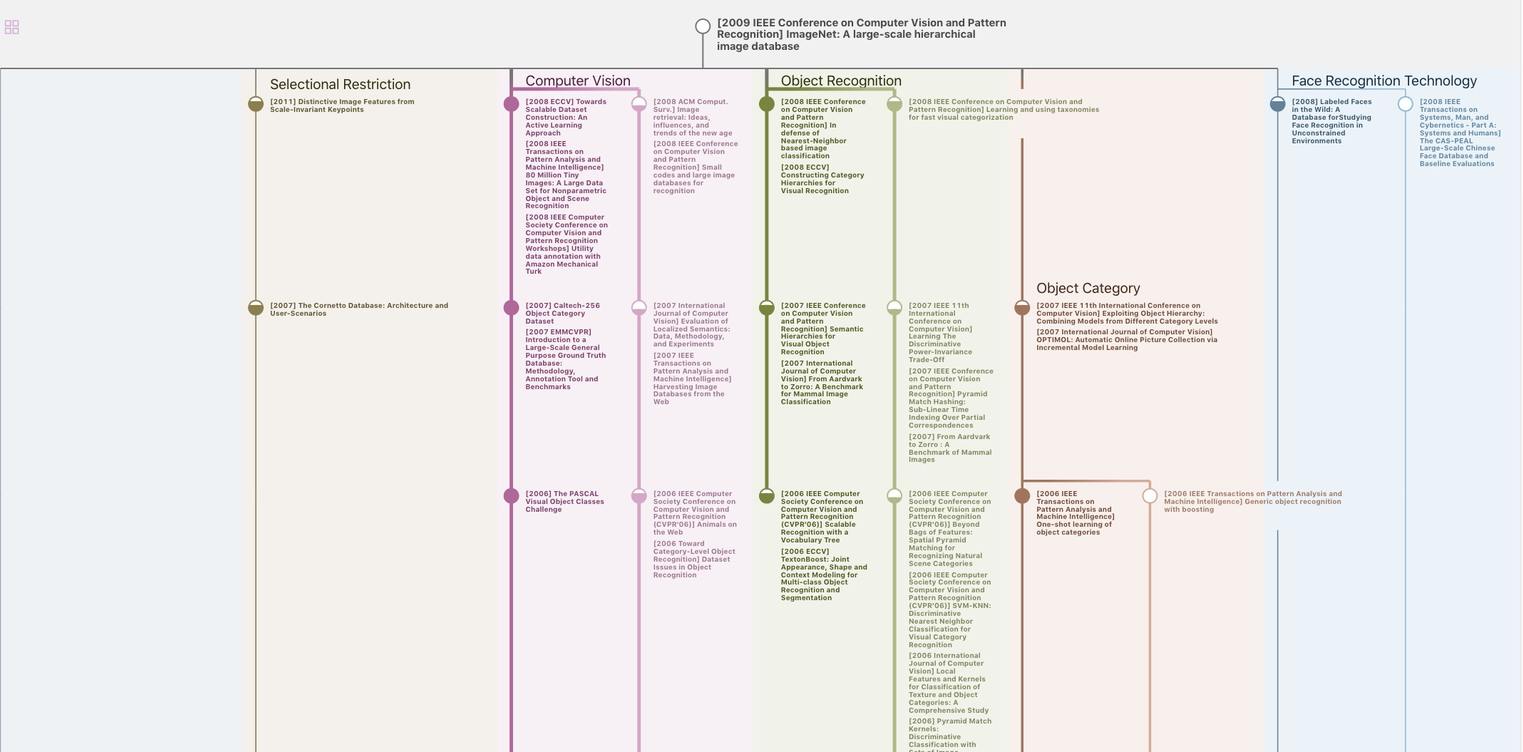
生成溯源树,研究论文发展脉络
Chat Paper
正在生成论文摘要