Next Position Prediction using LSTM Neural Networks.
SETN(2020)
摘要
Movement data is a valuable source of information in the context of many applications. Deep Learning (DL) provides useful methods for the modeling and the knowledge extraction from them. An adaptation of a DL approach to time series analysis can improve significantly the prediction accuracy with the cost of increasing the training time and consequently the resource demands. In this paper we propose the use of Artificial Neural Networks (ANN) with LSTM layers for the next position prediction of moving objects using a genetic algorithm and a transfer learning method. The genetic algorithm makes a smart search in the the hypothesis space to estimate a close to optimal ANN architecture and the model leverages a transfer learning method to exploit a repository of well trained ANN models in order to speed up the training process. We evaluated our proposed model with real data from the trajectory of various vessels and compared with a state of the art trajectory prediction method and two well-known AutoML meta models. The experimental results showed that the proposed model has better accuracy than other models and the transfer learning process decreases dramatically the training time. The results encourage us for the applicability of our method.
更多查看译文
AI 理解论文
溯源树
样例
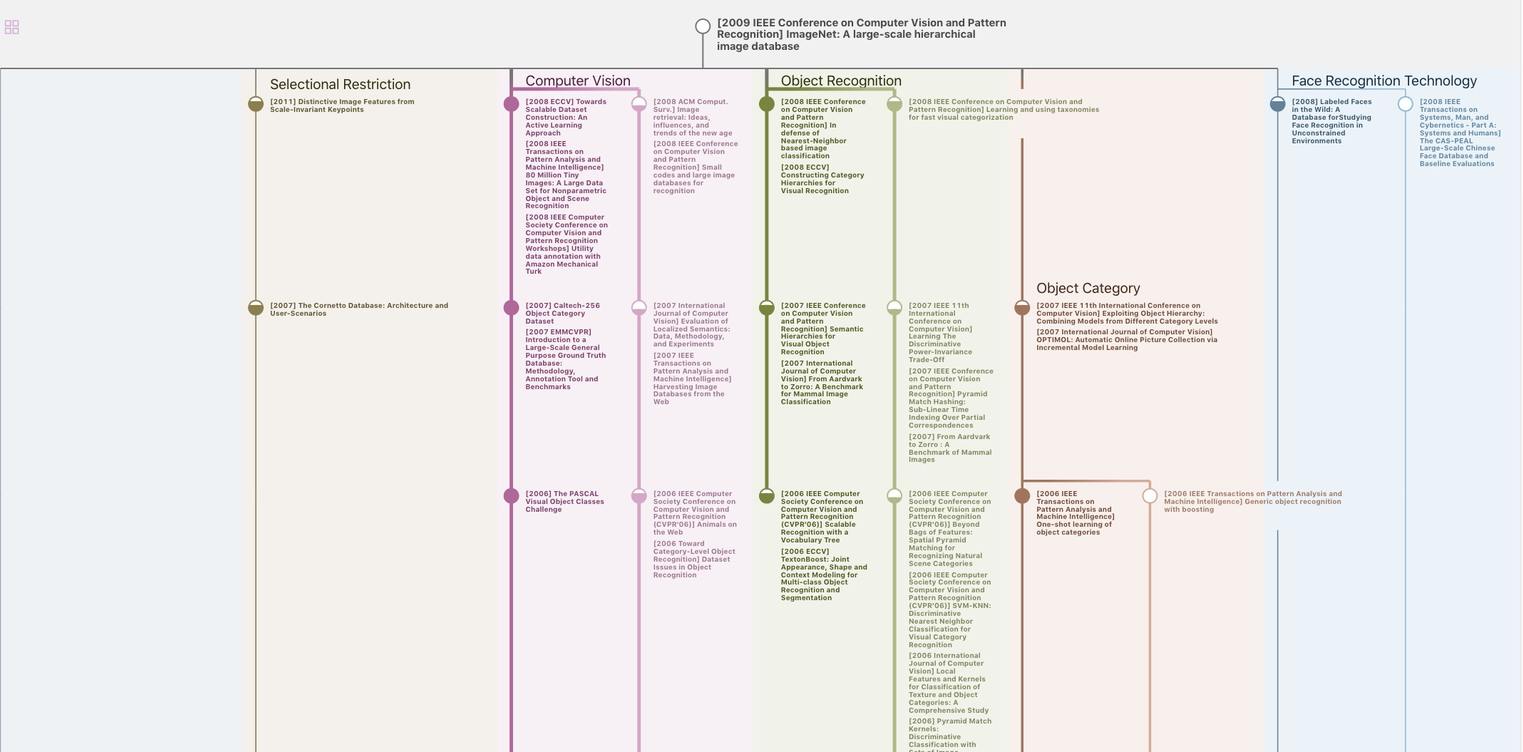
生成溯源树,研究论文发展脉络
Chat Paper
正在生成论文摘要