Apprenticeship learning of flight trajectories prediction with inverse reinforcement learning.
SETN(2020)
摘要
One of the primary goals of Artificial Intelligence research is to develop machines with human-like intelligence, perception and reasoning. In this direction teaching apprentice agents by observing demonstrations delivered by experts is a framework of imitation learning that can provide improved solutions and it is possible to significantly outperform the demonstrator. Inverse reinforcement learning (IRL) is a paradigm relying on Markov Decision Processes (MDPs) that has a twofold target: to learn optimum policies of autonomous agents for solving complex tasks from successful demonstrations, and also to discover the unknown reward function that could explain the expert behavior. In this article we are addressing the trajectory prediction problem in the aviation domain by using an IRL approach. The proposed learning scheme provides an imitation process where the algorithm tries to imitate demonstrated trajectories, exploiting raw trajectory data enriched with contextual features and learn an efficient reward model that is learned during imitation and has generalization capabilities to unknown cases. We show several experimental results using real trajectory data from the Spanish FIR that confirms the effectiveness of our approach in automatically predicting trajectories.
更多查看译文
AI 理解论文
溯源树
样例
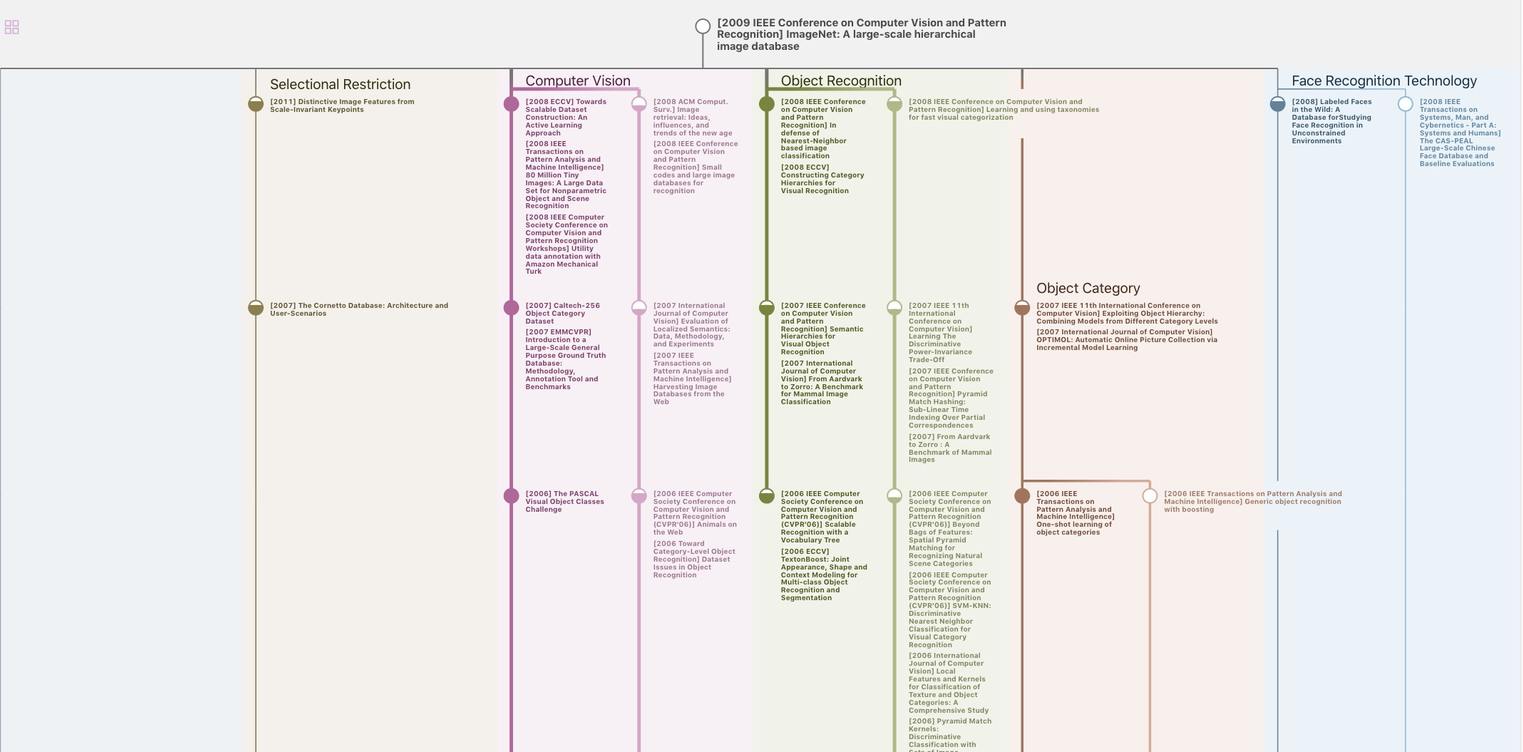
生成溯源树,研究论文发展脉络
Chat Paper
正在生成论文摘要