Incentives Needed for Low-Cost Fair Lateral Data Reuse.
FODS(2020)
摘要
A central goal of algorithmic fairness is to build systems with fairness properties that compose gracefully. A major effort and step towards this goal in data science has been the development offair representations which guarantee demographic parity under sequential composition by imposing ademographic secrecy constraint. In this work, we elucidate limitations of demographically secret fair representations and propose a fresh approach to potentially overcome them by incorporating information about parties' incentives into fairness interventions. Specifically, we show that in a stylized model, it is possible to relax demographic secrecy to obtainincentive-compatible representations, where rational parties obtain exponentially greater utilities vis-à-vis any demographically secret representation and satisfy demographic parity. These substantial gains are recovered not from the well-knowncost of fairness, but rather from acost of demographic secrecy which we formalize and quantify for the first time. We further show that the sequential composition property of demographically secret representations is not robust to aggregation. Our results open several new directions for research in fair composition, fair machine learning and algorithmic fairness.
更多查看译文
AI 理解论文
溯源树
样例
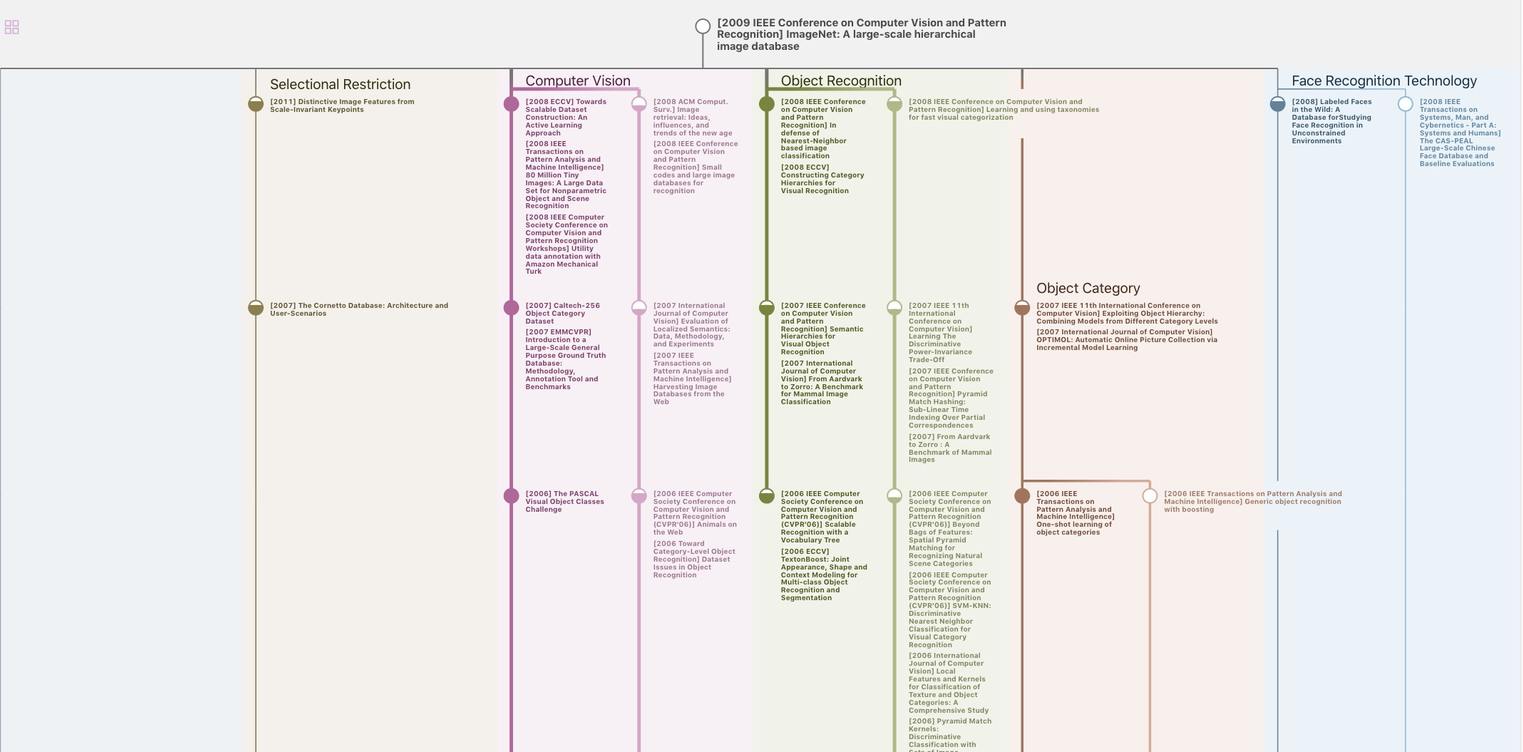
生成溯源树,研究论文发展脉络
Chat Paper
正在生成论文摘要