Asymmetric Convolution Networks Based on Multi-feature Fusion for Object Detection
2020 IEEE 16TH INTERNATIONAL CONFERENCE ON AUTOMATION SCIENCE AND ENGINEERING (CASE)(2020)
摘要
Real-time object detection on mobile platforms is a crucial but challenging computer vision task. However, it is widely recognized that although the lightweight object detectors have a high detection speed, the detection accuracy is relatively low. To address this problem, in this paper, we propose a new lightweight one-stage generic object detector, named ACFNet. The design goal of ACFNet is to improve the detection accuracy of object detectors while maintaining a high detection speed. In the backbone part, we design an asymmetric convolution unit, namely AC block, which employs three asymmetric convolution branches to enhance multi-feature representation of CNNs. We follow the backbone part design in ShuffleNetV2, and present a backbone network called ACNet that exploits AC blocks to replace the standard convolutional layers, e.g., 3×3 layers. Besides, in order to make all feature maps stronger semantically, we design a deconvolution block. In the prediction block, spatial attention can make the network better locate the feature distribution. Following this principle, we add a spatial attention building block to the residual block of each prediction layer. Experiments on PASCAL VOC 2007 and VOC 2012 datasets demonstrate the effectiveness of our proposed method and can run at 19 FPS on a single GPU.
更多查看译文
关键词
PASCAL VOC 2007 dataset,VOC 2012 dataset,ACNet,ShuffleNetV2,multifeature representation,lightweight one-stage generic object detector,computer vision task,mobile platforms,real-time object detection,multifeature fusion,asymmetric convolution networks,residual block,spatial attention building block,feature distribution,prediction block,deconvolution block,feature maps,backbone network,backbone part design,asymmetric convolution branches,AC block,asymmetric convolution unit,ACFNet,detection accuracy,detection speed
AI 理解论文
溯源树
样例
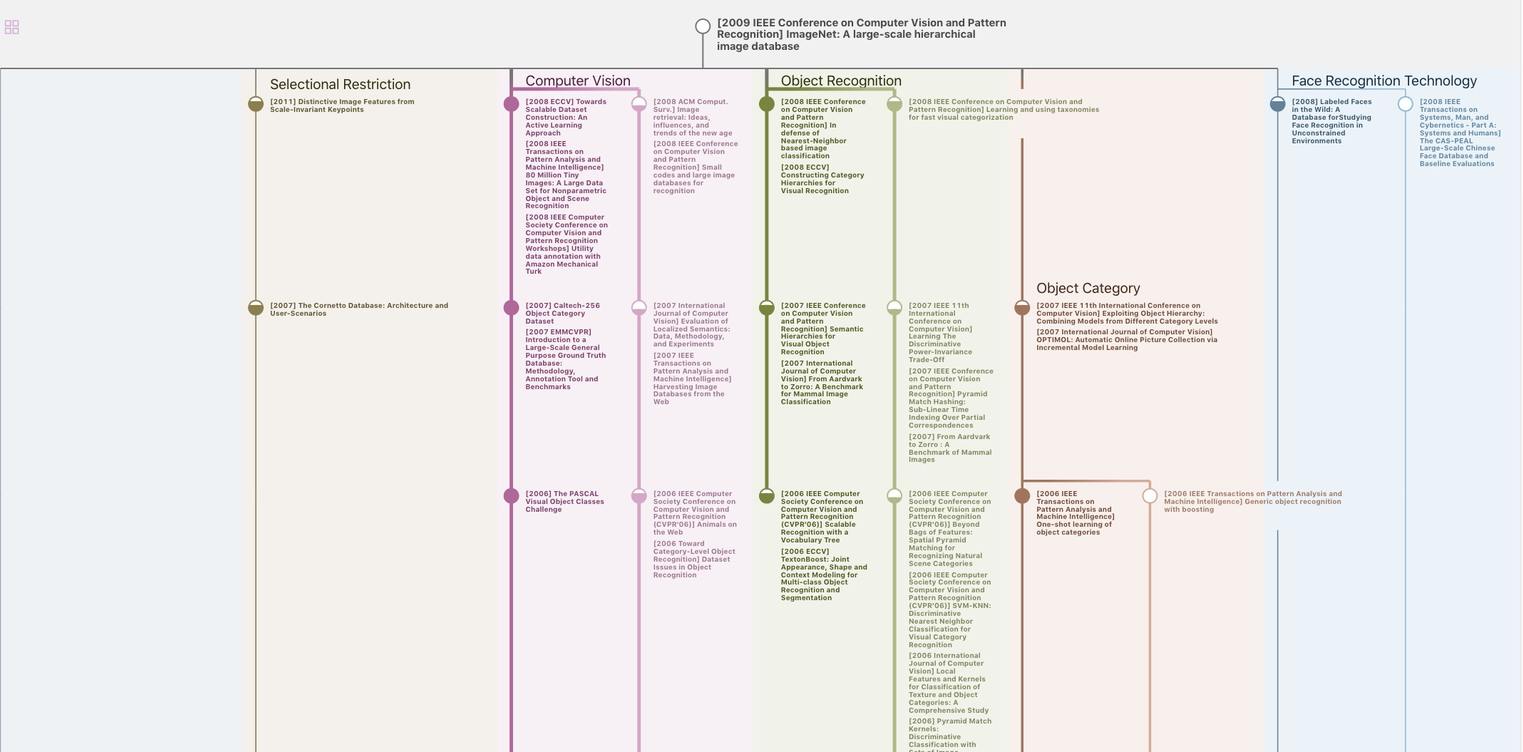
生成溯源树,研究论文发展脉络
Chat Paper
正在生成论文摘要