Sleep Stages Classification Using Cardio-Respiratory Variables
2020 IEEE 16TH INTERNATIONAL CONFERENCE ON AUTOMATION SCIENCE AND ENGINEERING (CASE)(2020)
摘要
Analysis of sleep is important in order to detect health issues and try to prevent them. In particular, sleep dysfunctions may be the first signs of cognitive frailties for elderly persons. The polysomnography (PSG) is considered the golden standard to perform a comprehensive sleep analysis, as it is based on several sensors placements. However, for longitudinal study of sleep that is required to prevent frailty for elderly persons, such medical equipment is not suitable since it is very invasive. Recent technological advances in sensors allow to gather data with a good precision with less intrusive equipment. The main objective of this study consists in developing a new algorithmic approach to analyse sleep using data from low intrusive sensors. In this study we focus on sleep phase detection, i.e. wake, Non-Rapid Eye Movement (NREM) and Rapid Eye Movement (REM). We consider the following sources of data: heart beat rate, as well as user data such as gender, age, etc. The problem is considered as a supervised classification machine learning problem. We propose a benchmark of several machine learning algorithms and compare their performances against the medical gold standard, the PSG. To do so, we use a data-set collected from a published clinical trial. Support Vector Machine (SVM) algorithm globally outperforms all other methods with a 76.5% agreement with the PSG. As a direct perspective of this study, we plan to add other sources of data using custom sensors to improve the performance of the prediction.
更多查看译文
关键词
Sleep stages, machine learning, supervised classification, sleep architecture, polysomnography
AI 理解论文
溯源树
样例
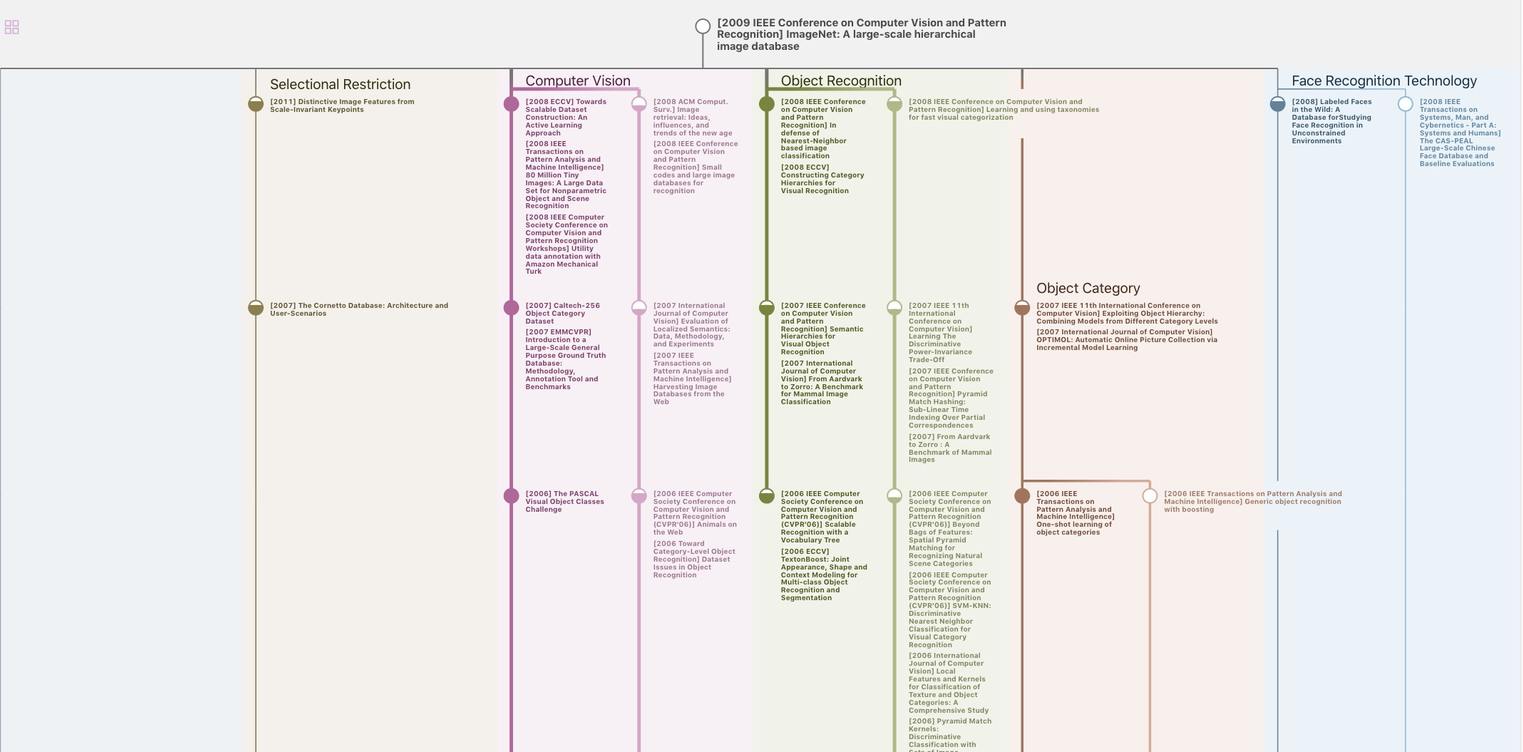
生成溯源树,研究论文发展脉络
Chat Paper
正在生成论文摘要