OAFuser: Online Adaptive Extended Object Tracking and Fusion using automotive Radar Detections
2020 IEEE International Conference on Multisensor Fusion and Integration for Intelligent Systems (MFI)(2020)
摘要
This paper presents the Online Adaptive Fuser: OAFuser, a novel method for online adaptive estimation of motion and measurement uncertainties for efficient tracking and fusion by applying a system of several estimators for ongoing noise along with the conventional state and state covariance estimation. In our system, process and measurement noises are estimated with steady-state filters to obtain combined measurement noise and process noise estimators for all sensors in order to obtain state estimation with a linear Minimum Mean Square Error (MMSE) estimator and accelerating the system's performance. The proposed adaptive tracking and fusion system was tested based on high fidelity simulation data and several real-world scenarios for automotive radar, where ground truth data is available for evaluation. We demonstrate the proposed method's accuracy and efficiency in a challenging, highly dynamic scenario where our system is benchmarked with Multiple Model filter in terms of error statistics and run time performance.
更多查看译文
关键词
state covariance estimation,measurement noise,steady-state filters,process noise estimators,linear minimum mean square error estimator,adaptive tracking,OAFuser,automotive radar detections,Online Adaptive Fuser,online adaptive estimation,measurement uncertainty,tracking efficiency,online adaptive extended object tracking,sensor fusion,multiple model filter,error statistics
AI 理解论文
溯源树
样例
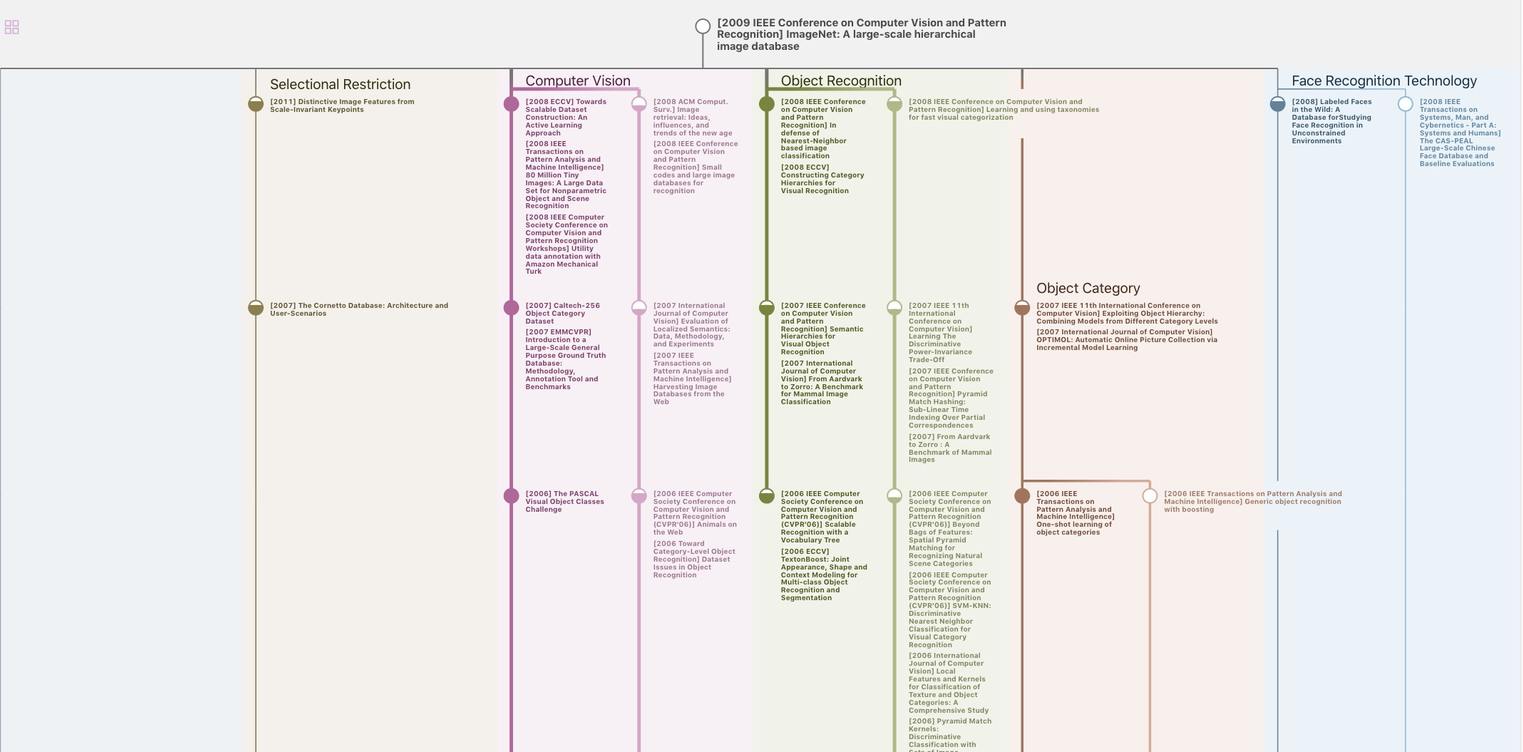
生成溯源树,研究论文发展脉络
Chat Paper
正在生成论文摘要