Explainability for Regression CNN in Fetal Head Circumference Estimation from Ultrasound Images.
iMIMIC/MIL3ID/LABELS@MICCAI(2020)
摘要
The measurement of fetal head circumference (HC) is performed throughout the pregnancy to monitor fetus growth using ultra-sound (US) images. Recently, methods that directly predict biometric from images, instead of resorting to segmentation, have emerged. In our previous work, we have proposed such method, based on a regression con-volutional neural network (CNN). If deep learning methods are the gold standard in most image processing tasks, they are often considered as black boxes and fails to provide interpretable decisions. In this paper, we investigate various saliency maps methods, to leverage their ability at explaining the predicted value of the regression CNN. Since saliency maps methods have been developed for classification CNN mostly, we provide an interpretation for regression saliency maps, as well as an adaptation of a perturbation-based quantitative evaluation of explanations methods. Results obtained on a public dataset of ultrasound images show that some saliency maps indeed exhibit the head contour as the most relevant features to assess the head circumference and also that the map quality depends on the backbone architecture and whether the prediction error is low or high.
更多查看译文
关键词
fetal head circumference estimation,regression cnn,ultrasound,images
AI 理解论文
溯源树
样例
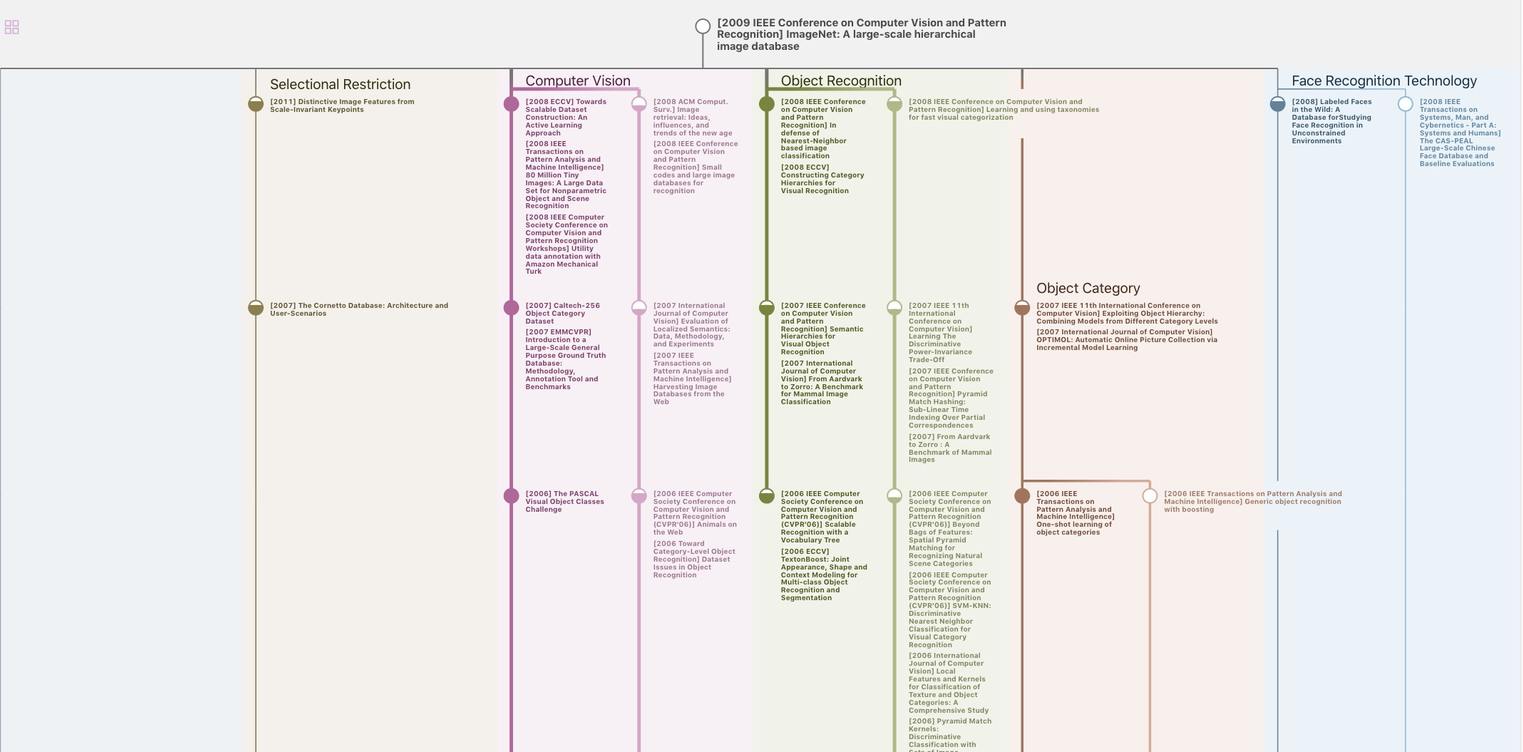
生成溯源树,研究论文发展脉络
Chat Paper
正在生成论文摘要