Partial Label Learning Via Generative Adversarial Nets
ECAI 2020: 24TH EUROPEAN CONFERENCE ON ARTIFICIAL INTELLIGENCE(2020)
摘要
Partial label learning (PLL) is a weakly supervised learning framework, in which each sample is provided with multiple candidate labels while only one of them is correct. Most of the existing methods are designed based on some conventional machine learning techniques, from kNN to SVM and logistic regression. Till now, it is still unclear whether we can use adversarial networks to solve partial label problems. This paper gives a positive answer to this question for the first time. We are the first to solve partial label learning with the network structure of CGAN combine SSGAN. In partial label learning with adversarial networks, it is interesting to find that some fake samples close to real sample distribution are generated, and then all these samples gradually promote discriminator to disambiguate the candidate labels of real samples. We give theoretical justifications of PL-GAN on challenging partial label data classification. Numerical experiments on artificial and real-world partial label datasets show that our approach significantly outperforms state-of-the-art counter-parts.
更多查看译文
AI 理解论文
溯源树
样例
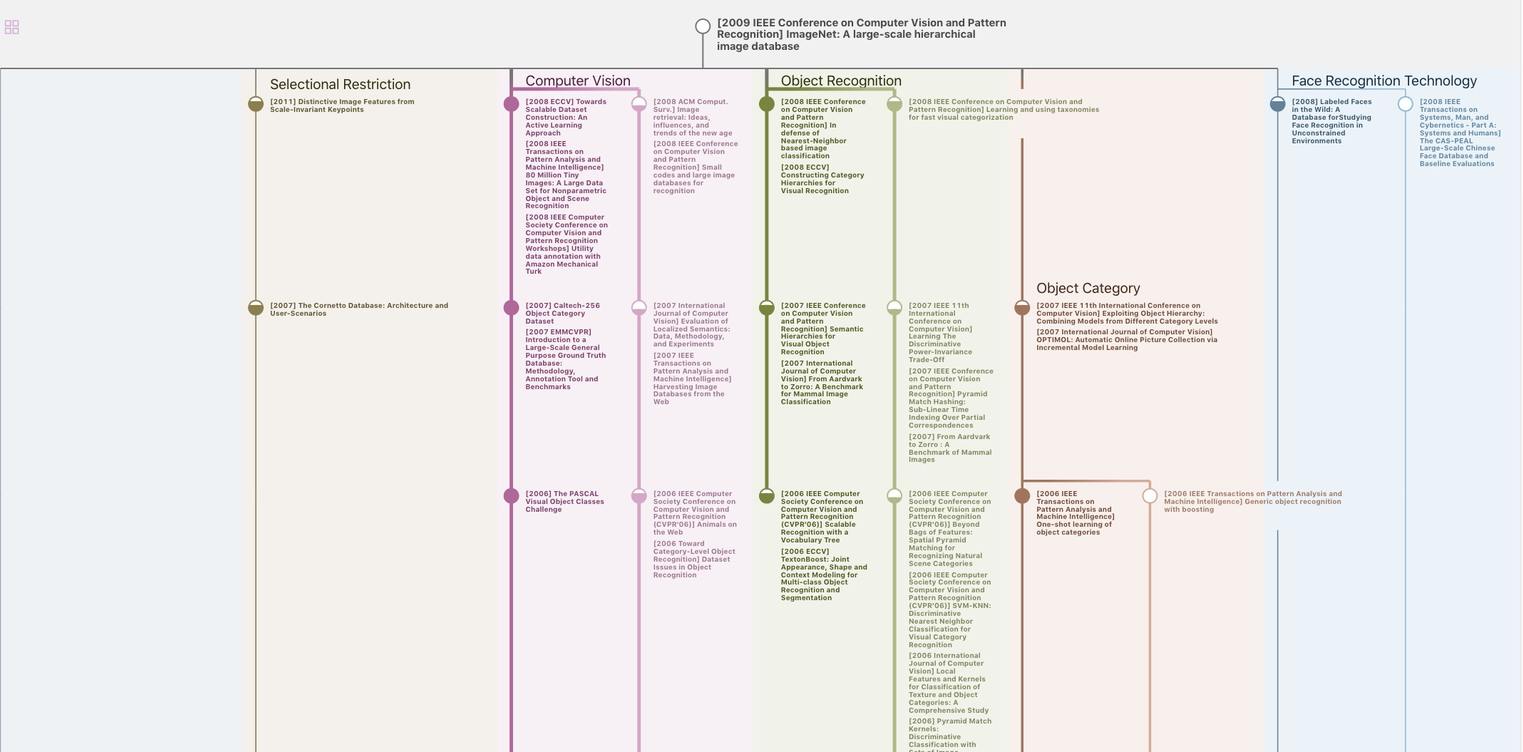
生成溯源树,研究论文发展脉络
Chat Paper
正在生成论文摘要