Common And Discriminative Semantic Pursuit For Multi-Modal Multi-Label Learning
ECAI 2020: 24TH EUROPEAN CONFERENCE ON ARTIFICIAL INTELLIGENCE(2020)
摘要
Multi-modal multi-label (MMML) learning provides an important framework to learn complex objects with diverse representations and annotations. Most existing multi-modal multi-label learning approaches focus on exploiting shared information of all modalities, but neglect specific information of each modality. Besides, how to effectively utilize relationship among modalities is also a challenging issue. In this paper, we propose a novel MMML learning approach called Common and Discriminative Semantic Pursuit (CoDiSP), which learns low-dimensional common representation with all modalities, and extracts discriminative information of each modality by enforcing orthogonal constraint. Meanwhile, the common representation is used as a new modality and added to the specific modal sequence. Furthermore, CoDiSP learns deep models with adaptive depth and exploits label correlations simultaneously based on the extracted modal sequence. Finally, extensive experiments on several benchmark MMML datasets show superior performance of CoDiSP compared with other state-of-the-art approaches.
更多查看译文
AI 理解论文
溯源树
样例
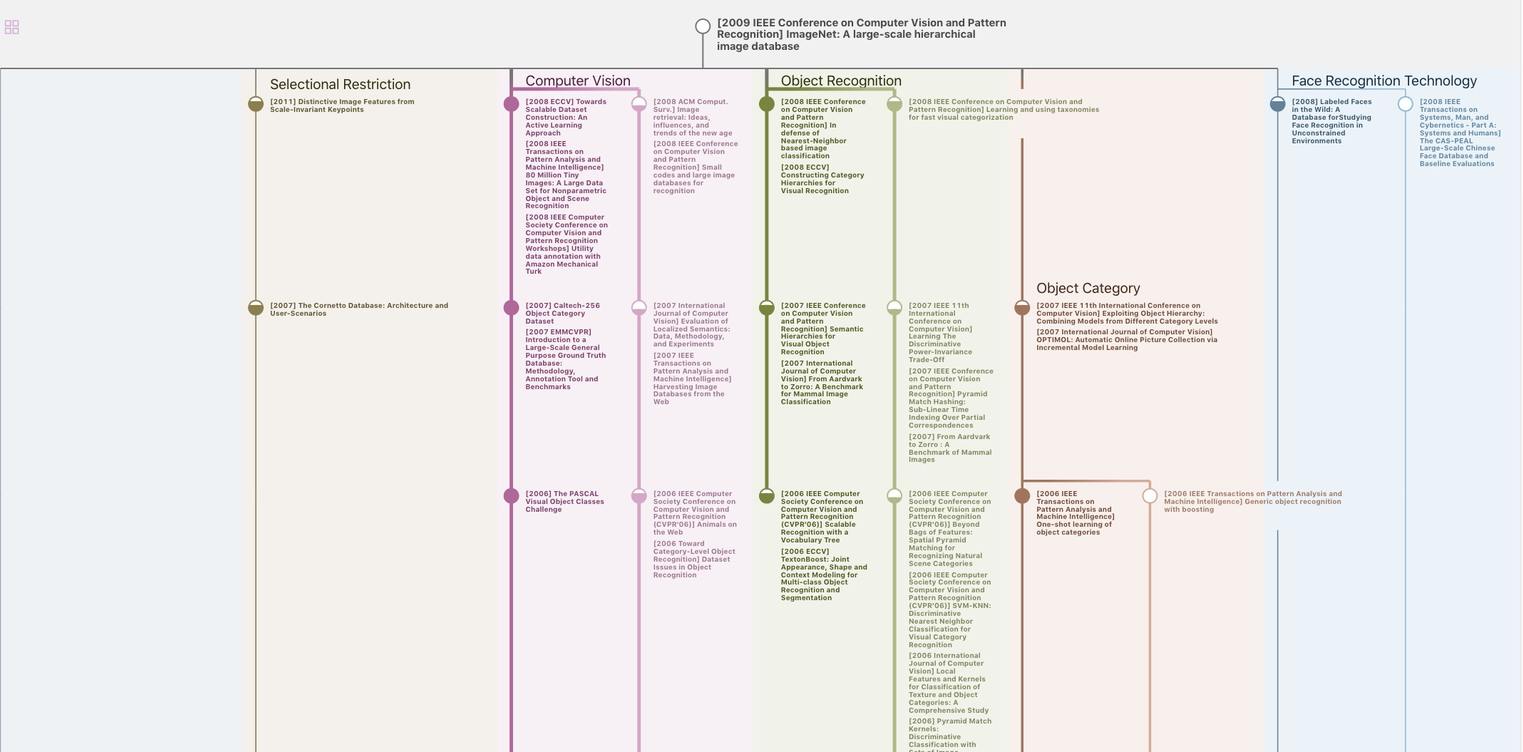
生成溯源树,研究论文发展脉络
Chat Paper
正在生成论文摘要