Directional Adversarial Training For Recommender Systems
ECAI 2020: 24TH EUROPEAN CONFERENCE ON ARTIFICIAL INTELLIGENCE(2020)
摘要
Adversarial training is shown as an effective method to improve the generalization ability of deep learning models by making random perturbations in the input space during model training. A recent study has successfully applied adversarial training into recommender systems by perturbing the embeddings of users and items through a minimax game. However, this method ignores the collaborative signal in recommender systems and fails to capture the smoothness in data distribution. We argue that the collaborative signal, which reveals the behavioural similarity between users and items, is critical to modeling recommender systems. In this work, we develop the Directional Adversarial Training (DAT) strategy by explicitly injecting the collaborative signal into the perturbation process. That is, both users and items are perturbed towards their similar neighbours in the embedding space with proper restriction. To verify its effectiveness, we demonstrate the use of DAT on Generalized Matrix Factorization (GMF), one of the most representative collaborative filtering methods. Our experimental results on three public datasets show that our method (called DAGMF) achieves a significant accuracy improvement over GMF and meanwhile, it is less prone to overfitting than GMF.
更多查看译文
AI 理解论文
溯源树
样例
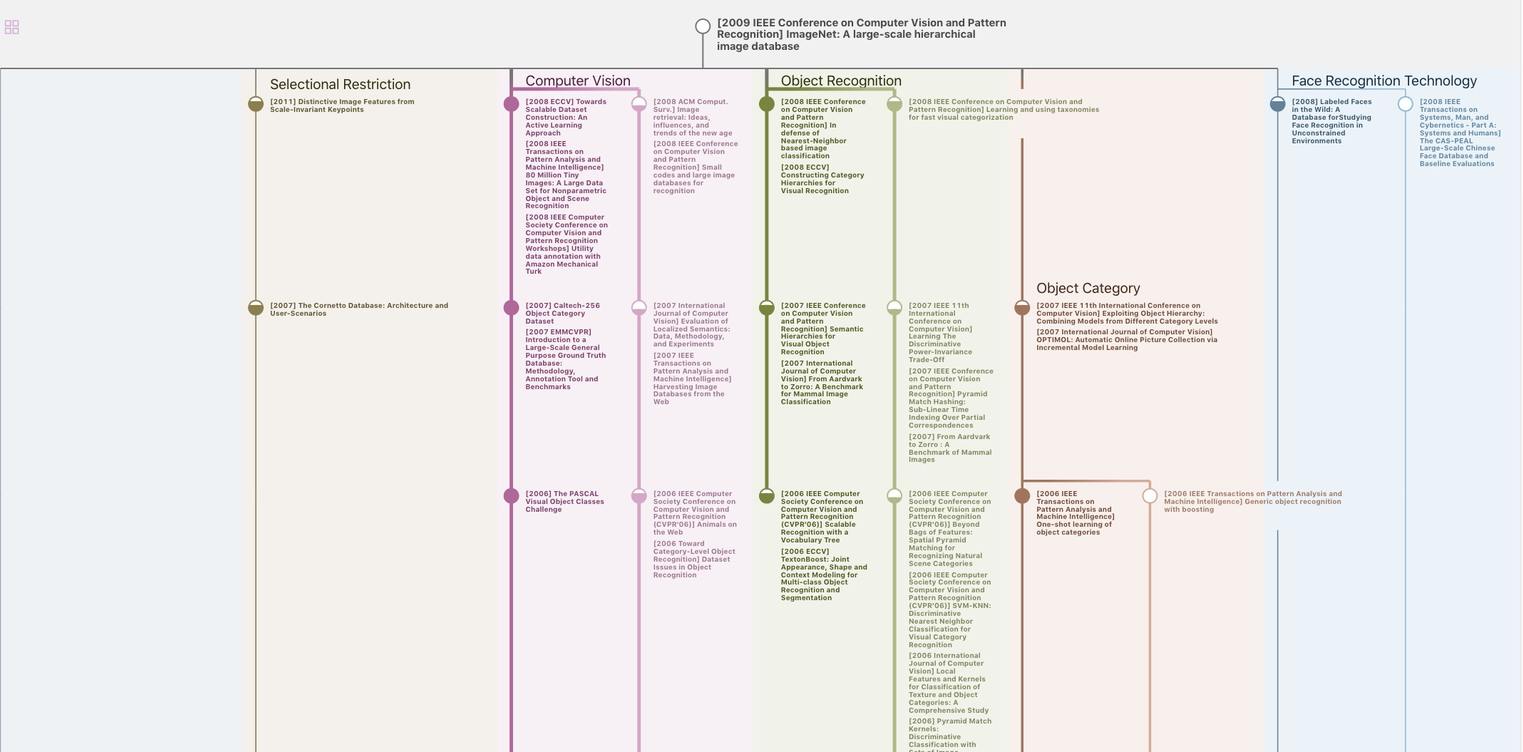
生成溯源树,研究论文发展脉络
Chat Paper
正在生成论文摘要