Learning Chordal Markov Networks Via Stochastic Local Search
ECAI 2020: 24TH EUROPEAN CONFERENCE ON ARTIFICIAL INTELLIGENCE(2020)
摘要
We present a novel stochastic local search (SLS) approach for the computationally hard task of finding a chordal Markov network structure that maximizes a given scoring function (CMSL for short). Current state of the art in exact algorithms for CMSL only scale up to circa 20 variables. Beyond this, the scalability of exact approaches is obstructed by the drastically increasing number of clique scores which grows exponentially with the number of variables. We show that, in practice, using only a fraction of the running times of the exact approaches, our SLS approach provides optimal or very close to optimal solutions for instance sizes that are within the reach of exact algorithms. Furthermore, we propose an on-the-fly clique score computation approach that enables scaling up our SLS approach towards hundreds of variables. In particular, on-the-fly score computation circumvents the need to enforce low treewidth bounds, which enable pre-computation of scores before search, but which also may severely limit the accuracy of the learned models.
更多查看译文
AI 理解论文
溯源树
样例
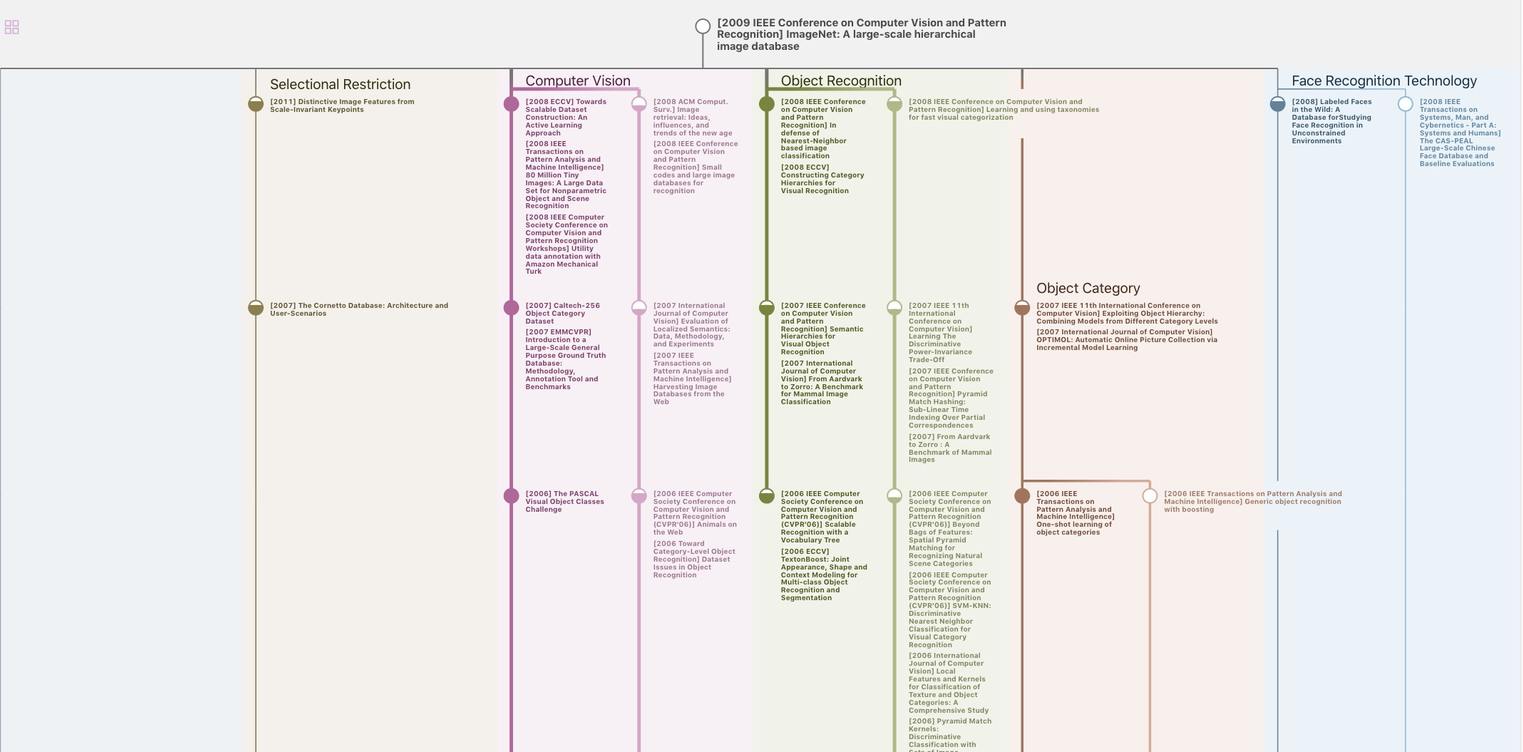
生成溯源树,研究论文发展脉络
Chat Paper
正在生成论文摘要