Abstract Interpretation Based Robustness Certification For Graph Convolutional Networks
ECAI 2020: 24TH EUROPEAN CONFERENCE ON ARTIFICIAL INTELLIGENCE(2020)
摘要
Graph convolutional networks (GCNs) have attracted much attention and become a powerful tool for graph data analysis. However, recent studies show that these methods are vulnerable to adversarial attacks (e.g. changing node attributes). Although several works have proposed to improve the robustness of GCNs, only a few works address their provable robustness. In this work, we propose a novel Abstract Interpretation (AI) based method for scalable robustness certification of graph convolutional networks. Different from the AI-based certification in the image classification task whose data is continuous, the considered perturbation on node attributes in this paper is binary. To address this challenge, our central idea is to over-approximate all possible perturbations of the first layer output instead of the input layer. Abstract transformers for graph convolutional operations are further defined to prove the robustness automatically. Experimental results on three public graph datasets demonstrate that our method is faster than the state-of-the-art certification approach.
更多查看译文
AI 理解论文
溯源树
样例
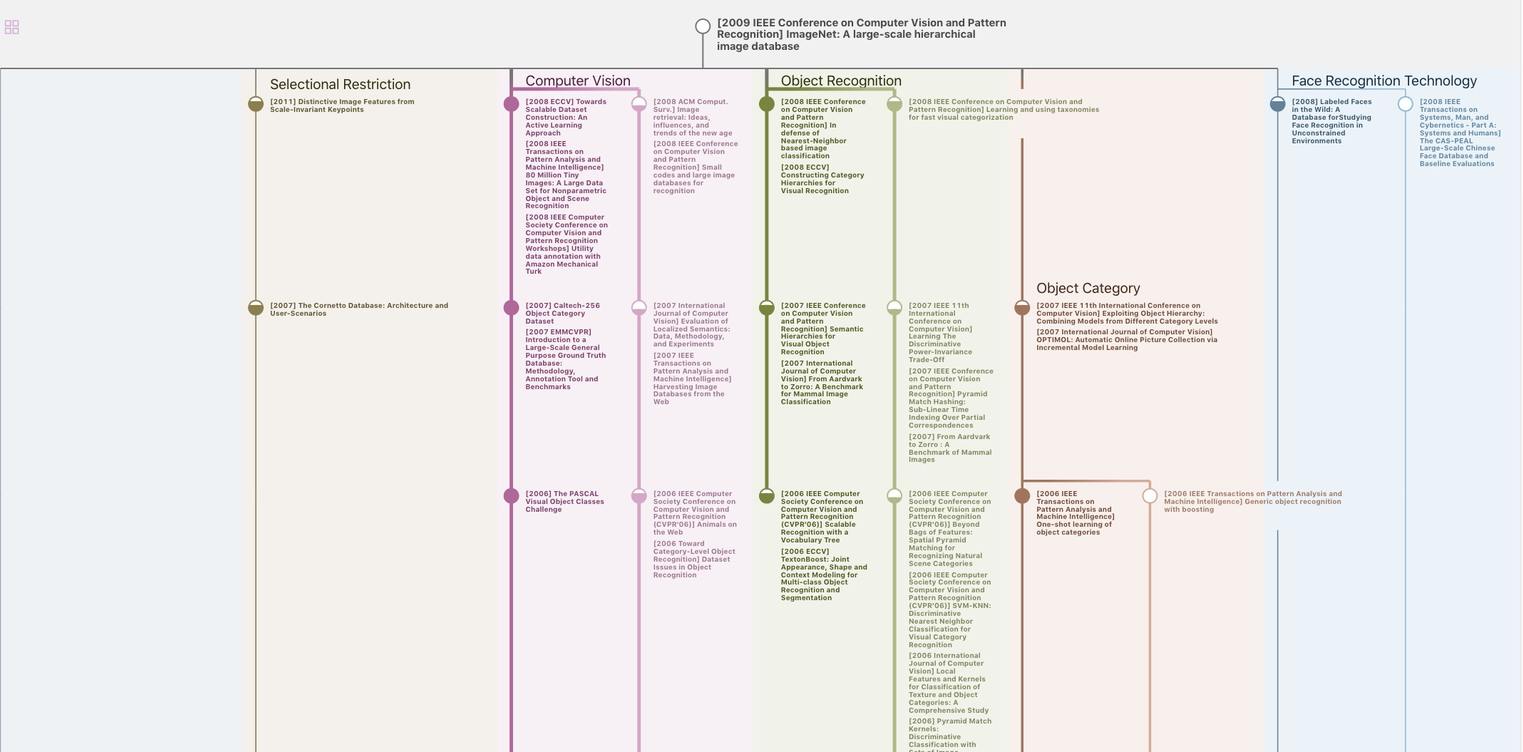
生成溯源树,研究论文发展脉络
Chat Paper
正在生成论文摘要