End-To-End Speech Emotion Recognition Based On Time And Frequency Information Using Deep Neural Networks
ECAI 2020: 24TH EUROPEAN CONFERENCE ON ARTIFICIAL INTELLIGENCE(2020)
摘要
We propose a speech emotion recognition system based on deep neural networks, operating on raw speech data in an end-to-end manner to predict continuous emotions in arousal-valence space. The model is trained using time and frequency information of speech recordings of the publicly available part of the multi-modal RECOLA database. We use the Concordance Correlation Coefficient (CCC) as it was proposed by the Audio-Visual Emotion Challenges to measure the similarity between the network prediction and goldstandard. The CCC prediction results of our model outperform the results achieved by other state-of-the-art end-to-end models. The innovative aspect of our study is an end-to-end approach to using data that previously was mostly used by approaches involving combinations of pre-processing or post-processing. Our study used only a small subset of the RECOLA dataset and obtained better results than previous studies that used the full dataset.
更多查看译文
AI 理解论文
溯源树
样例
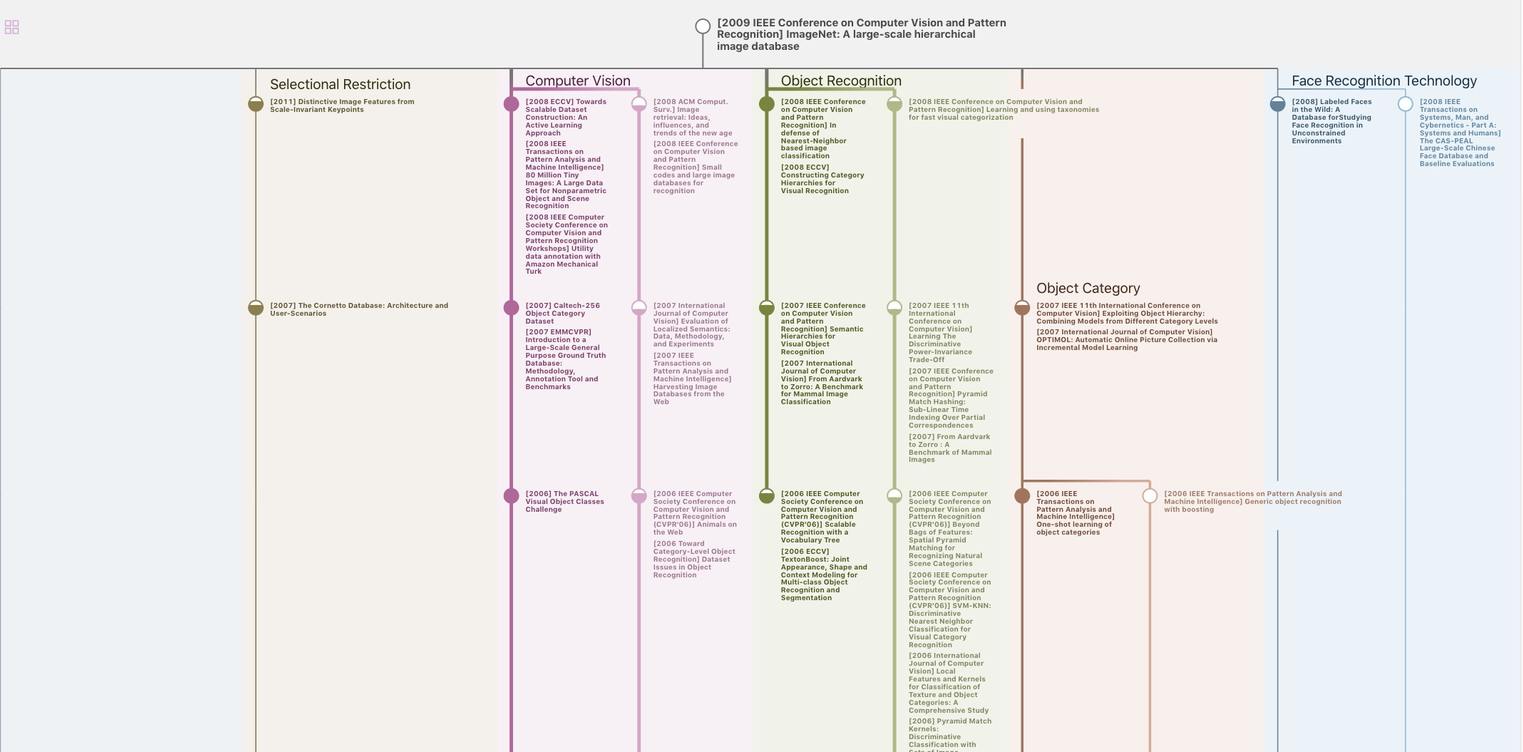
生成溯源树,研究论文发展脉络
Chat Paper
正在生成论文摘要