On Leveraging Pretrained GANs for Generation with Limited Data.
ICML(2020)
摘要
Recent work has shown generative adversarial networks (GANs) can generate highly realistic images, that are often indistinguishable (by humans) from real images. Most images so generated are not contained in the training dataset, suggesting potential for augmenting training sets with GAN-generated data. While this scenario is of particular relevance when there are limited data available, there is still the issue of training the GAN itself based on that limited data. To facilitate this, we leverage existing GAN models pretrained on large-scale datasets (like ImageNet) to introduce additional knowledge (which may not exist within the limited data), following the concept of transfer learning. Demonstrated by natural-image generation, we reveal that low-level filters (those close to observations) of both the generator and discriminator of pretrained GANs can be transferred to facilitate generation in a perceptually-distinct target domain with limited training data. To further adapt the transferred filters to the target domain, we propose adaptive filter modulation (AdaFM). An extensive set of experiments is presented to demonstrate the effectiveness of the proposed techniques on generation with limited data.
更多查看译文
关键词
pretrained gans,generation
AI 理解论文
溯源树
样例
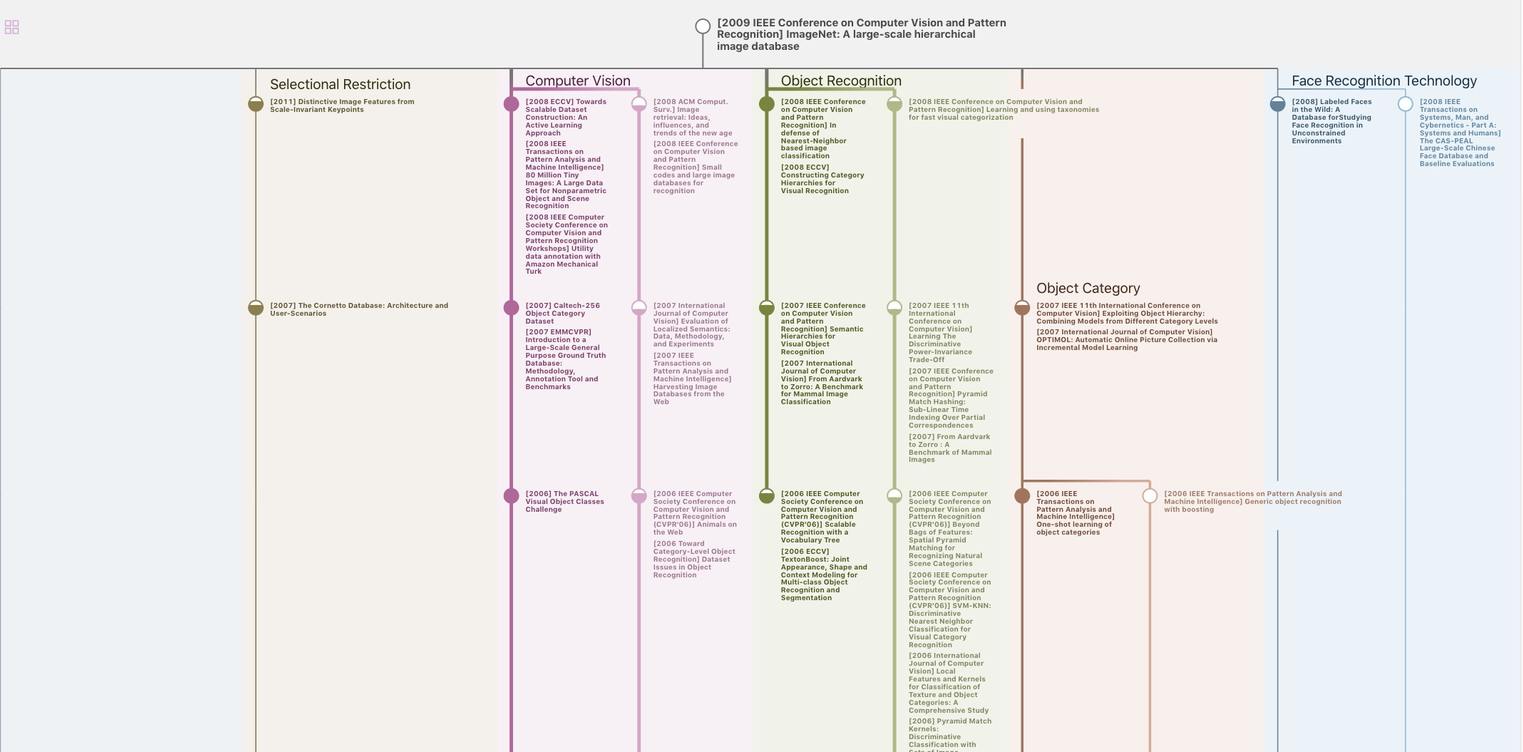
生成溯源树,研究论文发展脉络
Chat Paper
正在生成论文摘要