Question Generation Through Transfer Learning.
IEA/AIE(2020)
摘要
An automatic question generation (QG) system aims to produce questions from a text, such as a sentence or a paragraph. Traditional approaches are mainly based on heuristic, hand-crafted rules to transduce a declarative sentence to a related interrogative sentence. However, creating such a set of rules requires deep linguistic knowledge and most of these rules are language-specific. Although a data-driven approach reduces the participation of linguistic experts, to get sufficient labeled data for QG model training is still a difficult task. In this paper, we applied a neural sequence-to-sequence pointer-generator network with various transfer learning strategies to capture the underlying information of making a question, on a target domain with rare training pairs. Our experiment demonstrates the viability of domain adaptation in QG task. We also show the possibility that transfer learning is helpful in a semi-supervised approach when the amount of training pairs in the target QG dataset is not large enough.
更多查看译文
关键词
generation,learning,transfer
AI 理解论文
溯源树
样例
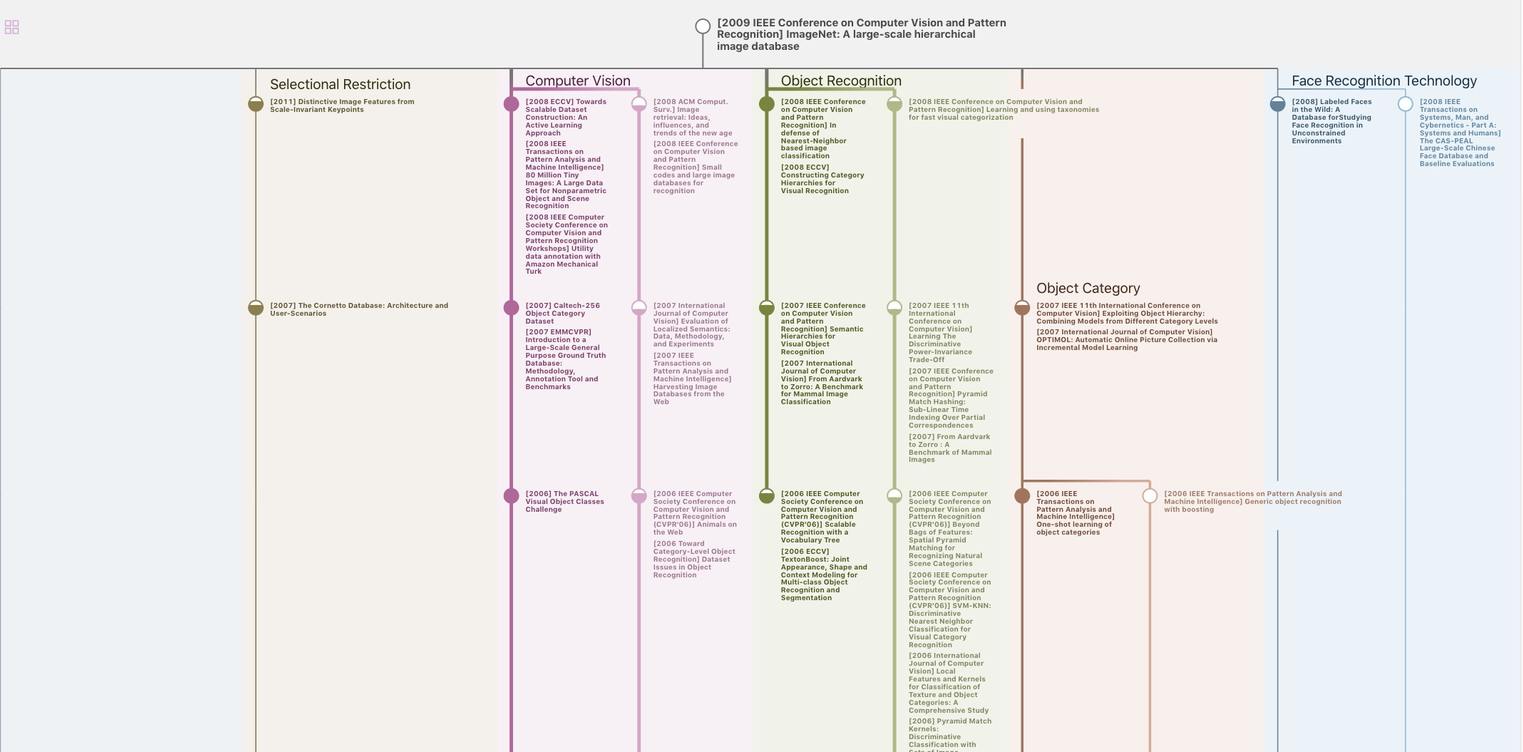
生成溯源树,研究论文发展脉络
Chat Paper
正在生成论文摘要