Self-Supervised Adversarial Multi-Task Learning for Vocoder-Based Monaural Speech Enhancement.
INTERSPEECH(2020)
摘要
In our previous study, we introduce the neural vocoder into monaural speech enhancement, in which a flow-based generative vocoder is used to synthesize speech waveforms from the Mel power spectra enhanced by a denoising autoencoder. As a result, this vocoder-based enhancement method outperforms several state-of-the-art models on a speaker-dependent dataset. However, we find that there is a big gap between the enhancement performance on the trained and untrained noises. Therefore, in this paper, we propose the self-supervised adversarial multi-task learning (SAMLE) to improve the noise generalization ability. In addition, the speaker dependence is also evaluated for the vocoder-based methods, which is important for real-life applications. Experimental results show that the proposed SAMLE further improves the enhancement performance on both trained and untrained noises, resulting in a better noise generalization ability. Moreover, we find that vocoder-based enhancement methods can be speaker-independent through a large-scale training.
更多查看译文
关键词
self-supervised, multi-task learning, vocoder-based speech enhancement
AI 理解论文
溯源树
样例
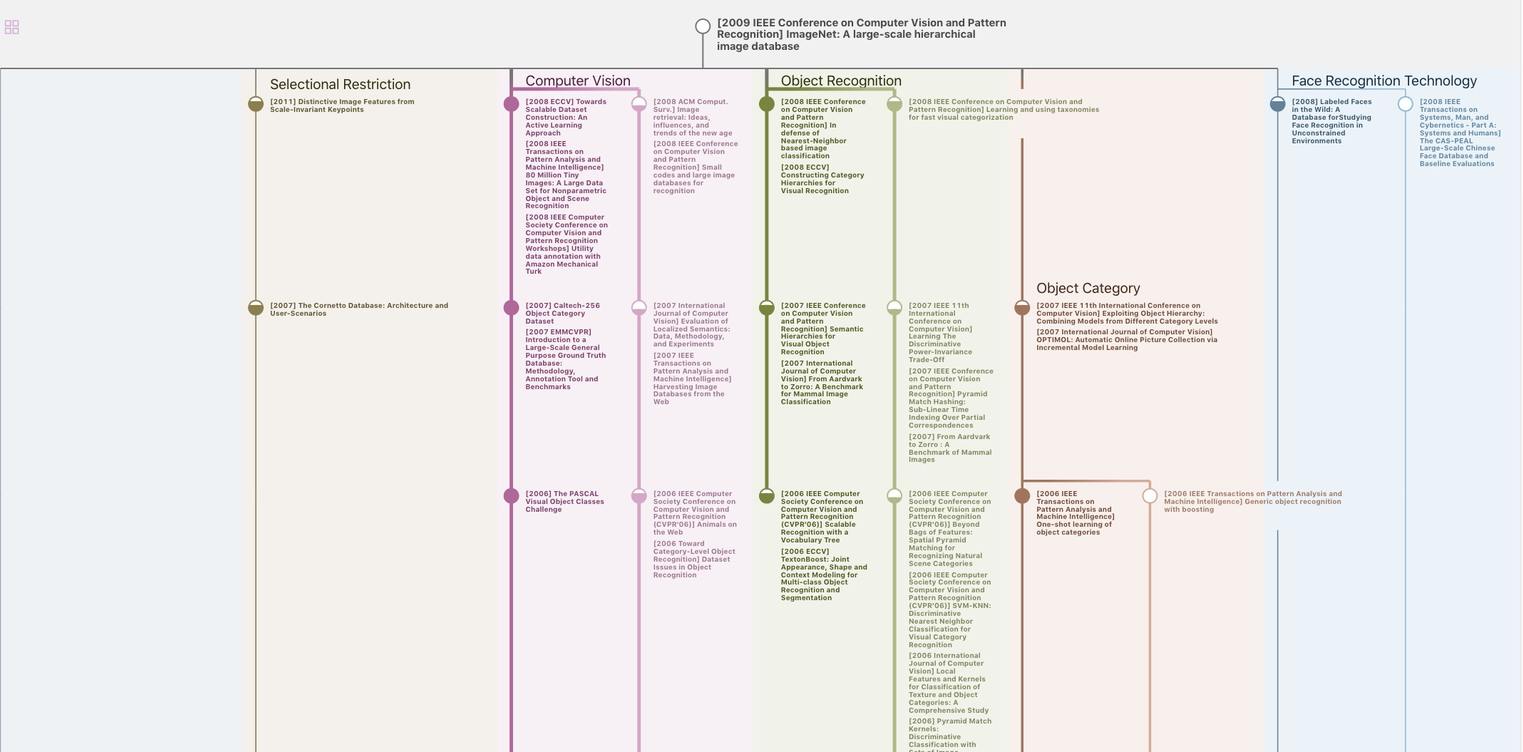
生成溯源树,研究论文发展脉络
Chat Paper
正在生成论文摘要