A Deep Profiling and Visualization Framework to Audit Clinical Assessment Variation.
CBMS(2020)
摘要
Clinical assessment variation (CAV) has a profound impact on patient outcomes, and appropriate tooling is critically needed to help understand and guide necessary interventions. In this study, we propose an intuitive approach to visualizing CAV and summarizing the contexts pertinent to decision-making. By superimposing the response variable and clusters learned according to the explanatory variables, a color-coded 2D scatter plot can be rendered to show the spatial proximity and semantic composition of the clusters. Without loss of generality, an example application on preoperative patient assessment demonstrated the approach can assist in auditing inconsistent human decisions and informing the reconciliation process. The methods will also benefit refining of clinical assessment guidelines by systematically eliciting practice-based knowledge.
更多查看译文
关键词
Clinical Practice Variation, Deep Learning, Patient Similarity, Information Visualization, Decision-Support System
AI 理解论文
溯源树
样例
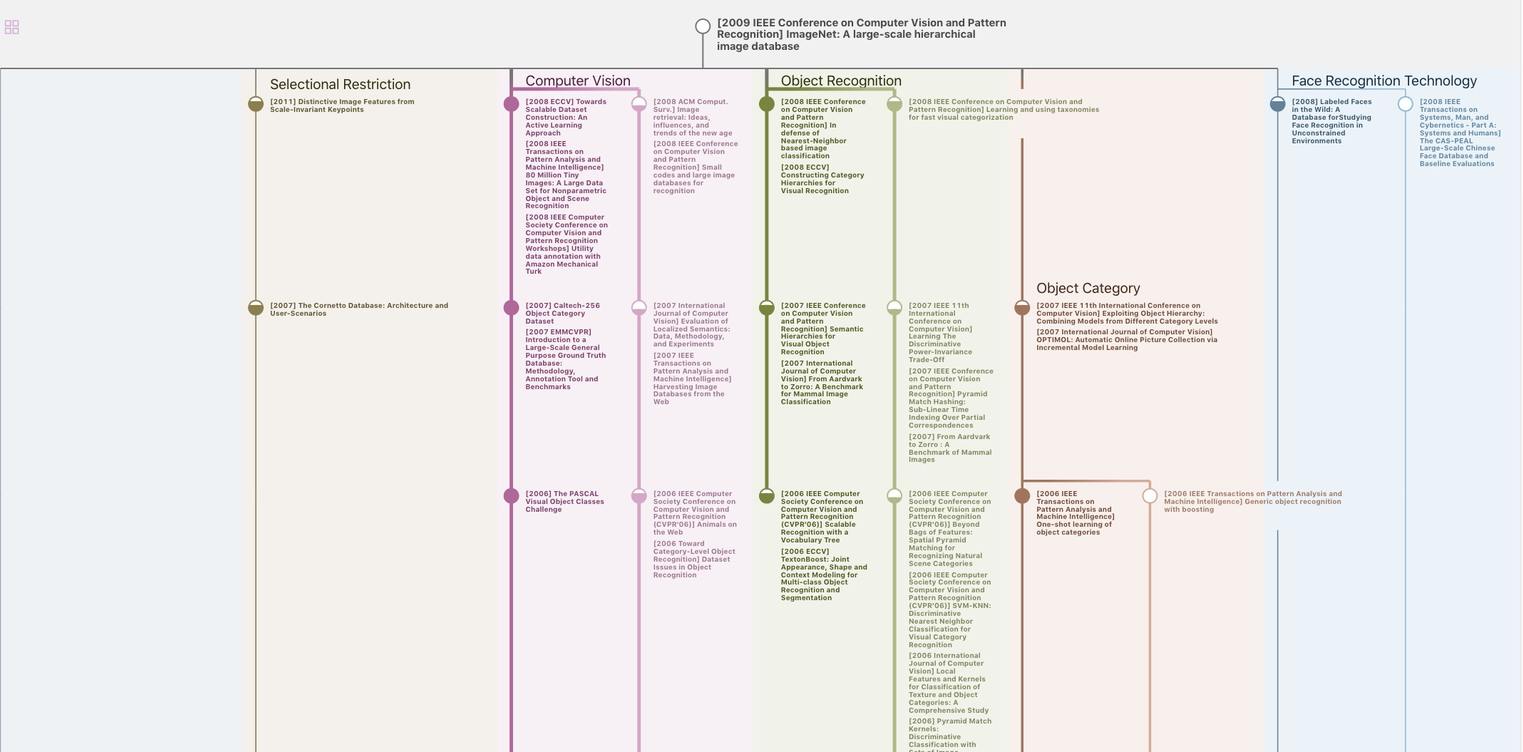
生成溯源树,研究论文发展脉络
Chat Paper
正在生成论文摘要