Pixel-Based Tool Segmentation in Cataract Surgery Videos with Mask R-CNN.
CBMS(2020)
摘要
Automatically detecting surgical tools in recorded surgery videos is an important building block of further content-based video analysis. In ophthalmology, the results of such methods can support training and teaching of operation techniques and enable investigation of medical research questions on a dataset of recorded surgery videos. While previous methods used frame-based classification techniques to predict the presence of surgical tools - but did not localize them, we apply a recent deep-learning segmentation method (Mask R-CNN) to localize and segment surgical tools used in ophthalmic cataract surgery. We add ground-truth annotations for multi-class instance segmentation to two existing datasets of cataract surgery videos and make resulting datasets publicly available for research purposes. In the absence of comparable results from literature, we tune and evaluate the Mask R-CNN approach on these datasets for instrument segmentation/localization and achieve promising results (61% mean average precision on 50% intersection over union for instance segmentation, working even better for bounding box detection or binary segmentation), establishing a reasonable baseline for further research. Moreover, we experiment with common data augmentation techniques and analyze the achieved segmentation performance with respect to each class (instrument), providing evidence for future improvements of this approach.
更多查看译文
关键词
cataract surgeries,instrument segmentation,tool annotation,deep neural networks,ophthalmology
AI 理解论文
溯源树
样例
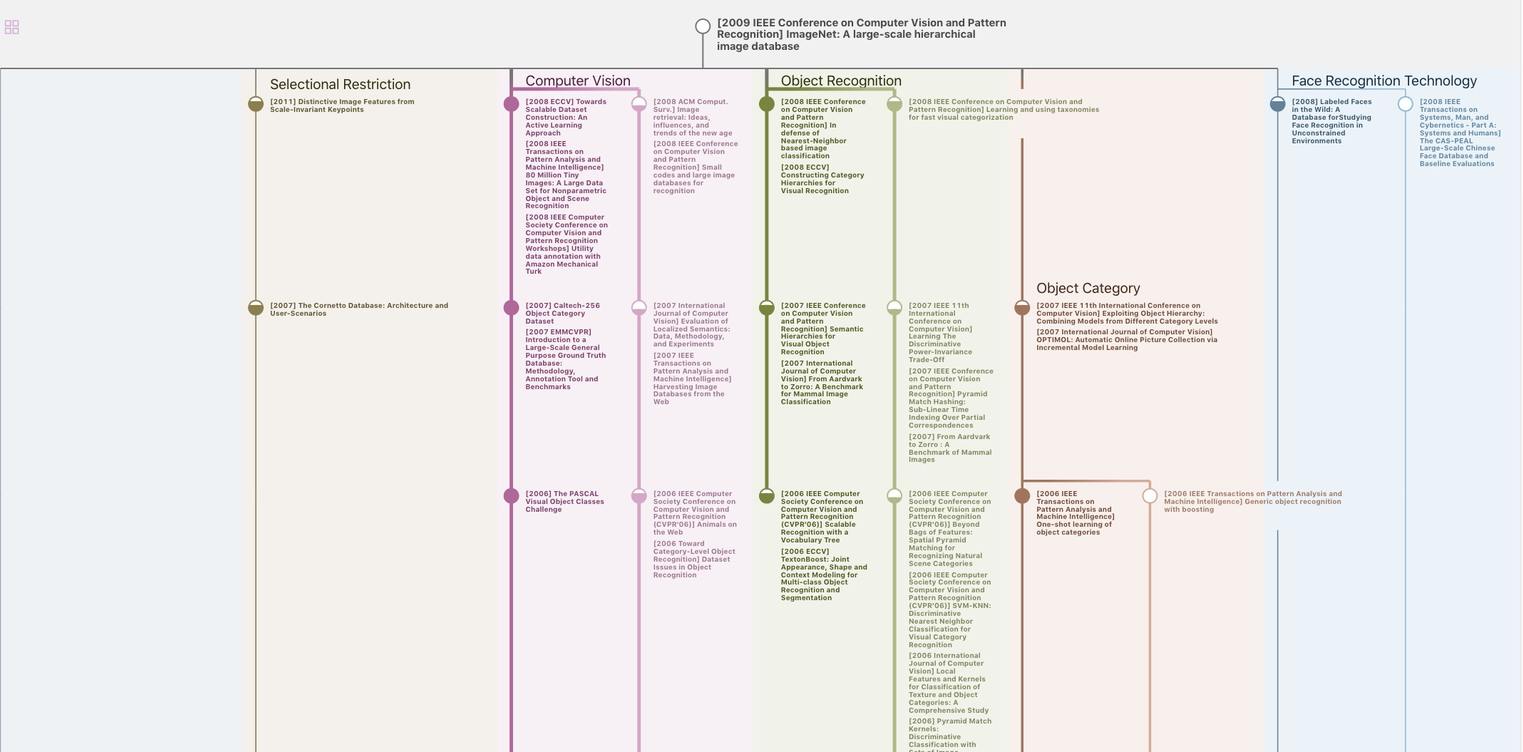
生成溯源树,研究论文发展脉络
Chat Paper
正在生成论文摘要