Multi-label Learning with a Cone-Based Geometric Model.
ICCS(2020)
摘要
Recent approaches for knowledge-graph embeddings aim at connecting quantitative data structures used in machine learning to the qualitative structures of logics. Such embeddings are of a hybrid nature, they are data models that also exhibit conceptual structures inherent to logics. One motivation to investigate embeddings is to design conceptually adequate machine learning (ML) algorithms. This paper investigates a new approach to embedding ontologies into geometric models that interpret concepts by closed convex cones. As a proof of concept this cone-based embedding was implemented in a ML algorithm for weak supervised multi-label learning. The system was tested with the gene ontology and showed a performance similar to comparable approaches, but with the advantage of exhibiting the conceptual structure underlying the data.
更多查看译文
关键词
Concept learning, Knowledge graph embedding, Multi-label learning
AI 理解论文
溯源树
样例
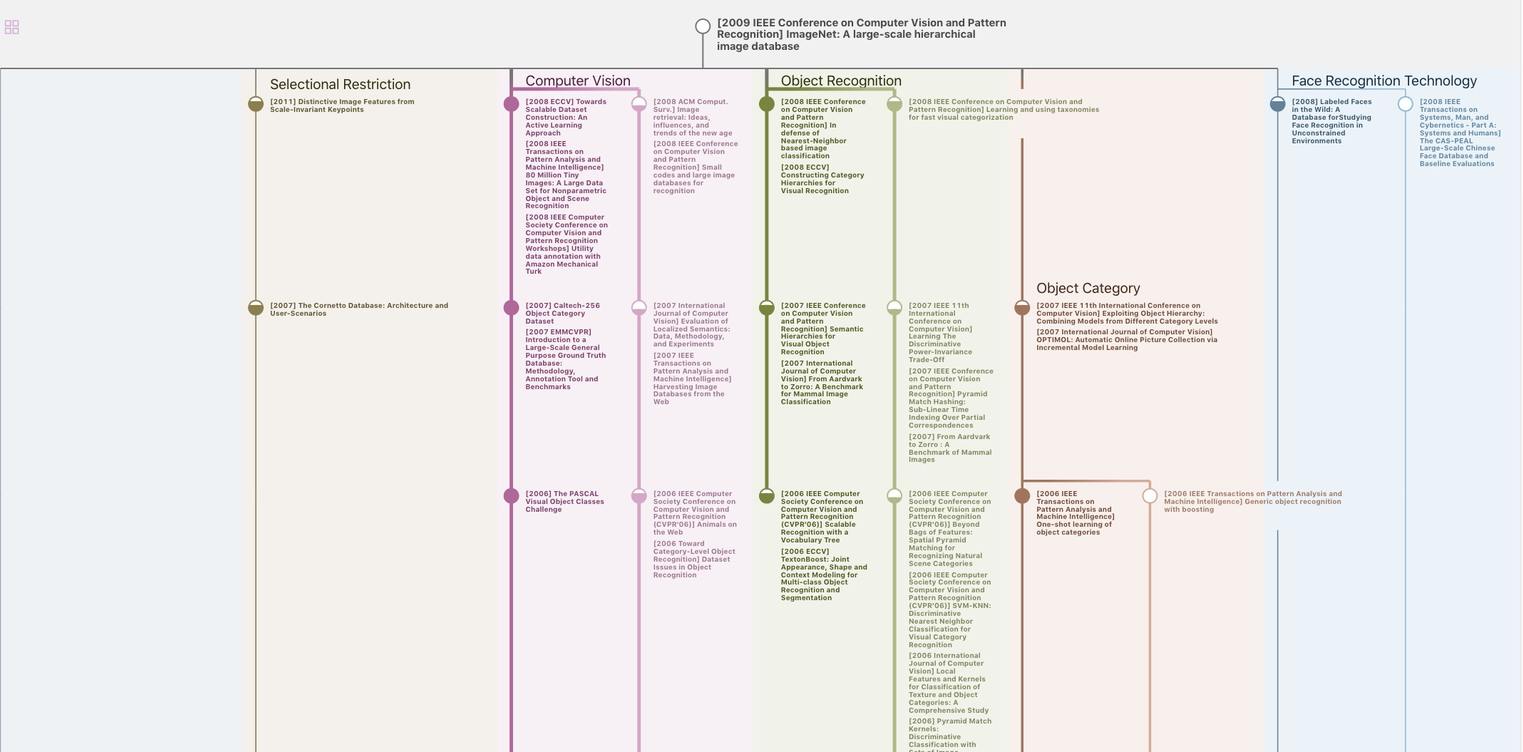
生成溯源树,研究论文发展脉络
Chat Paper
正在生成论文摘要