Tail: An Automated And Lightweight Gradient Compression Framework For Distributed Deep Learning
PROCEEDINGS OF THE 2020 57TH ACM/EDAC/IEEE DESIGN AUTOMATION CONFERENCE (DAC)(2020)
摘要
Existing gradient compression schemes fail to automatically determine the compression ratio or are accompanied by high compression overhead. To address this, we present Tail, an automated and lightweight gradient compression framework stacked by three modules, quantization, sparsification, and encoding. Without any hand-tuned effort, quantization module automatically adjusts the compression ratio along training iterations to retain accuracy first. Then, sparsification and encoding modules are successively applied to the quantized gradient to further improve compression ratio. Moreover, Tail reduces the compression overhead by approximate computing in the automated decision-making process. Experiments validate that Tail can reduce communication traffic by an order of magnitude while retaining or even improving model accuracy.
更多查看译文
关键词
Distributed training, gradient compression, communication, acceleration
AI 理解论文
溯源树
样例
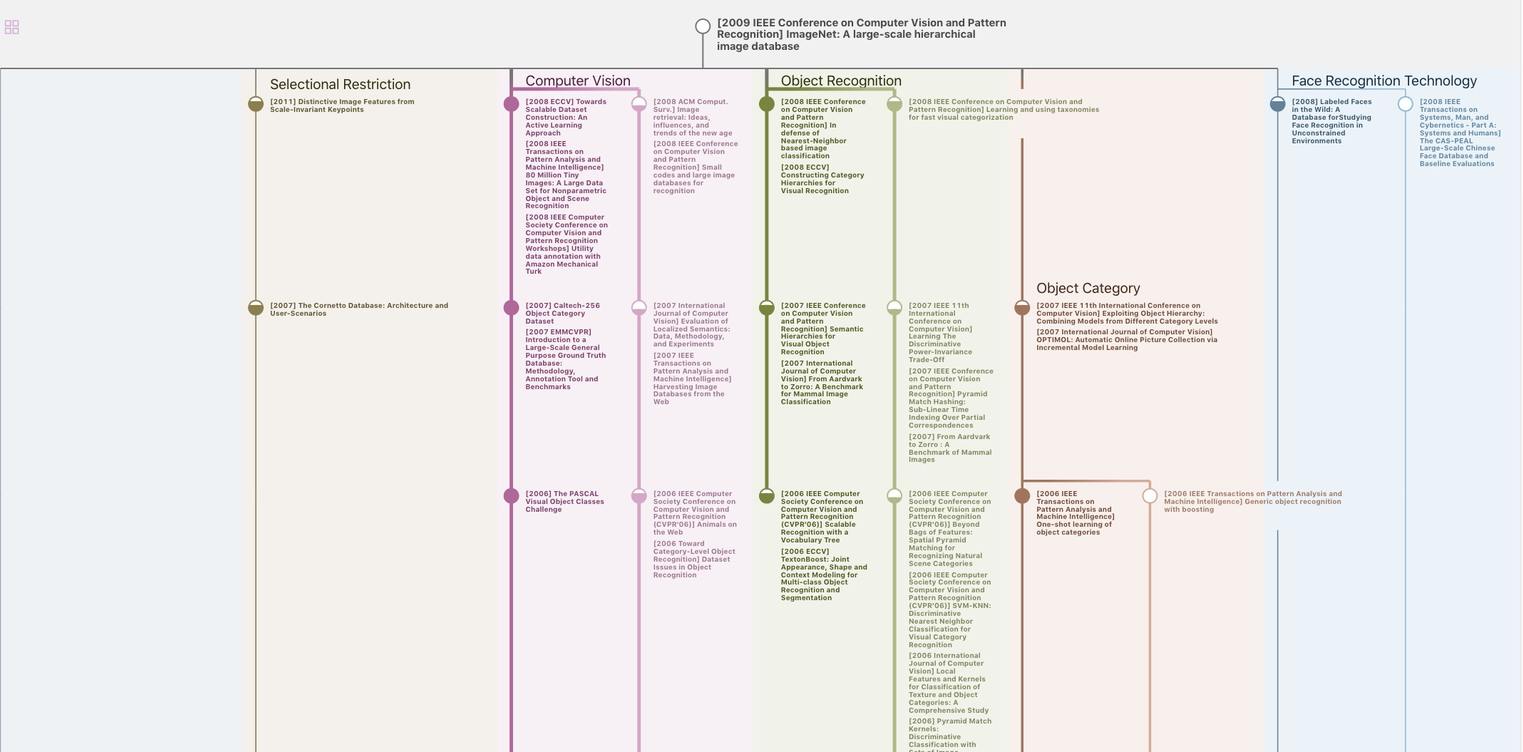
生成溯源树,研究论文发展脉络
Chat Paper
正在生成论文摘要