Content-Based Analytics: Moving beyond Data Size
2020 IEEE Sixth International Conference on Big Data Computing Service and Applications (BigDataService)(2020)
摘要
Efforts on Big Data technologies have been highly directed towards the amount of data a task can access or crunch. Yet, for content-driven decision making, it is not (only) about the size, but about the "right" data: The number of available datasets (a different type of volume) can reach astronomical sizes, making a thorough evaluation of each input prohibitively expensive. The problem is exacerbated as data sources regularly exhibit varying levels of uncertainty and velocity/churn. To date, there exists no efficient method to quantify the impact of numerous available datasets over different analytics tasks and workflows. This visionary work puts the spotlight on data content rather than size. It proposes a novel modeling, planning and processing research bundle that assesses data quality in terms of analytics performance. The main expected outcome is to provide efficient, continuous and intelligent management and execution of content-driven data analytics. Intelligent dataset selection can achieve massive gains on both accuracy and time required to reach a desired level of performance. This work introduces the notion of utilizing dataset similarity to infer operator behavior and, consequently, be able to build scalable, operator-agnostic performance models for Big Data tasks over different domains. We present an overview of the promising results from our initial work with numerical and graph data and respective operators. We then describe a reference architecture with specific areas of research that need to be tackled in order to provide a data-centric analytics ecosystem.
更多查看译文
关键词
modeling,data quality,big data,Machine Learning,scheduling
AI 理解论文
溯源树
样例
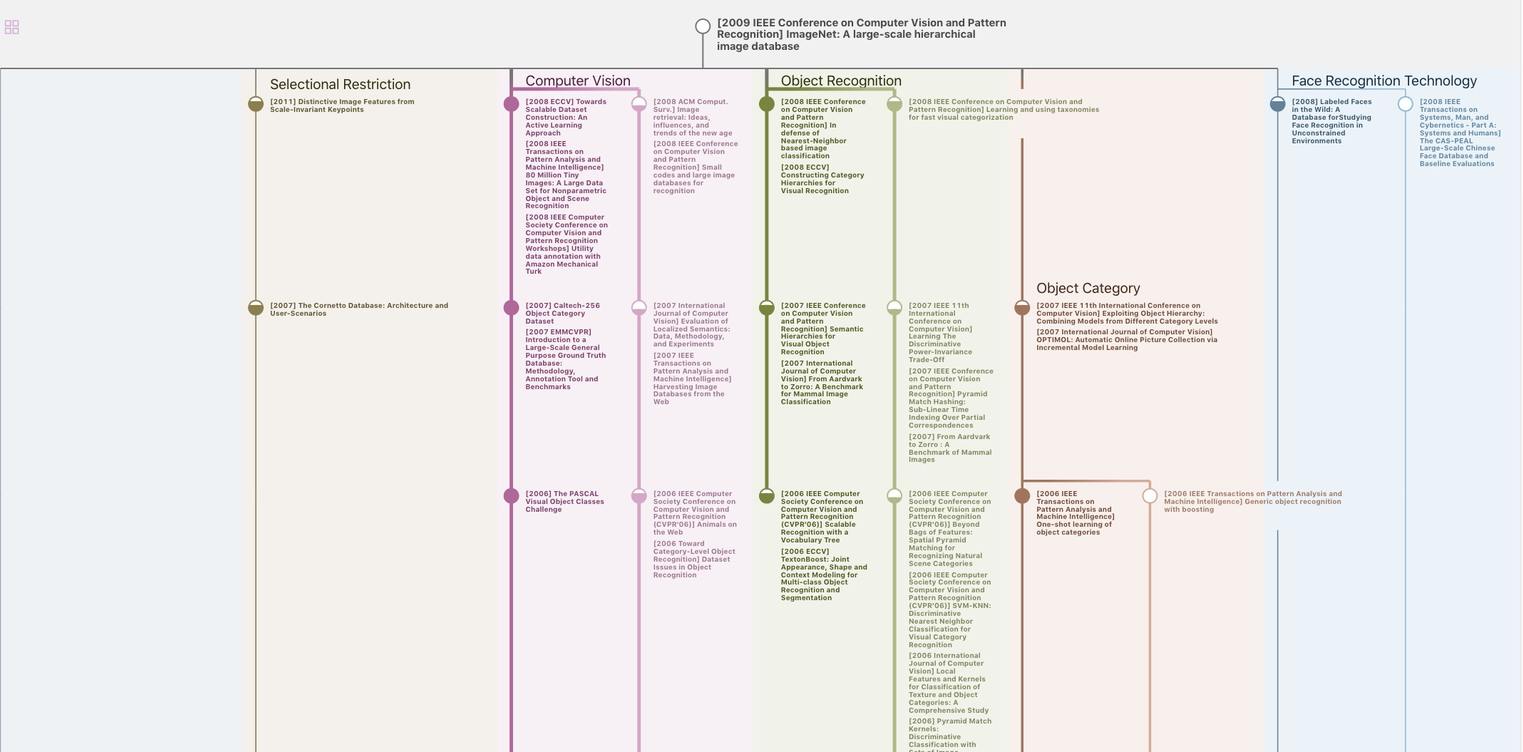
生成溯源树,研究论文发展脉络
Chat Paper
正在生成论文摘要