From Statistical Model Checking to Run-Time Monitoring Using a Bayesian Network Approach.
RV(2020)
摘要
We propose a framework for monitoring and updating, at run-time, the probabilities of temporal properties of stochastic timed automata. Our method is based on Bayesian networks and can be useful in various real-time applications, such as flight control systems and cardiac pacemakers. The framework has been implemented by exploiting the statistical model checking engine of Uppaal-SMC. By run-time monitoring a set of interesting temporal properties of a given stochastic automaton we update their probabilities, modeled through a Bayesian Network. The main advantages of our method are the capacity to discover non-trivial dependencies between properties and to efficiently update probabilities of unobserved properties given real-time observations. We present empirical results on three application scenarios, showing that the query time can keep up with the speed of some realistic real-time applications. We also present experiments demonstrating that the Bayesian Network approach performance-wise enables run-time monitoring while maintaining or even increasing the accuracy of probability estimation compared to statistical model checking.
更多查看译文
关键词
statistical model checking,monitoring,bayesian network approach,statistical model,run-time
AI 理解论文
溯源树
样例
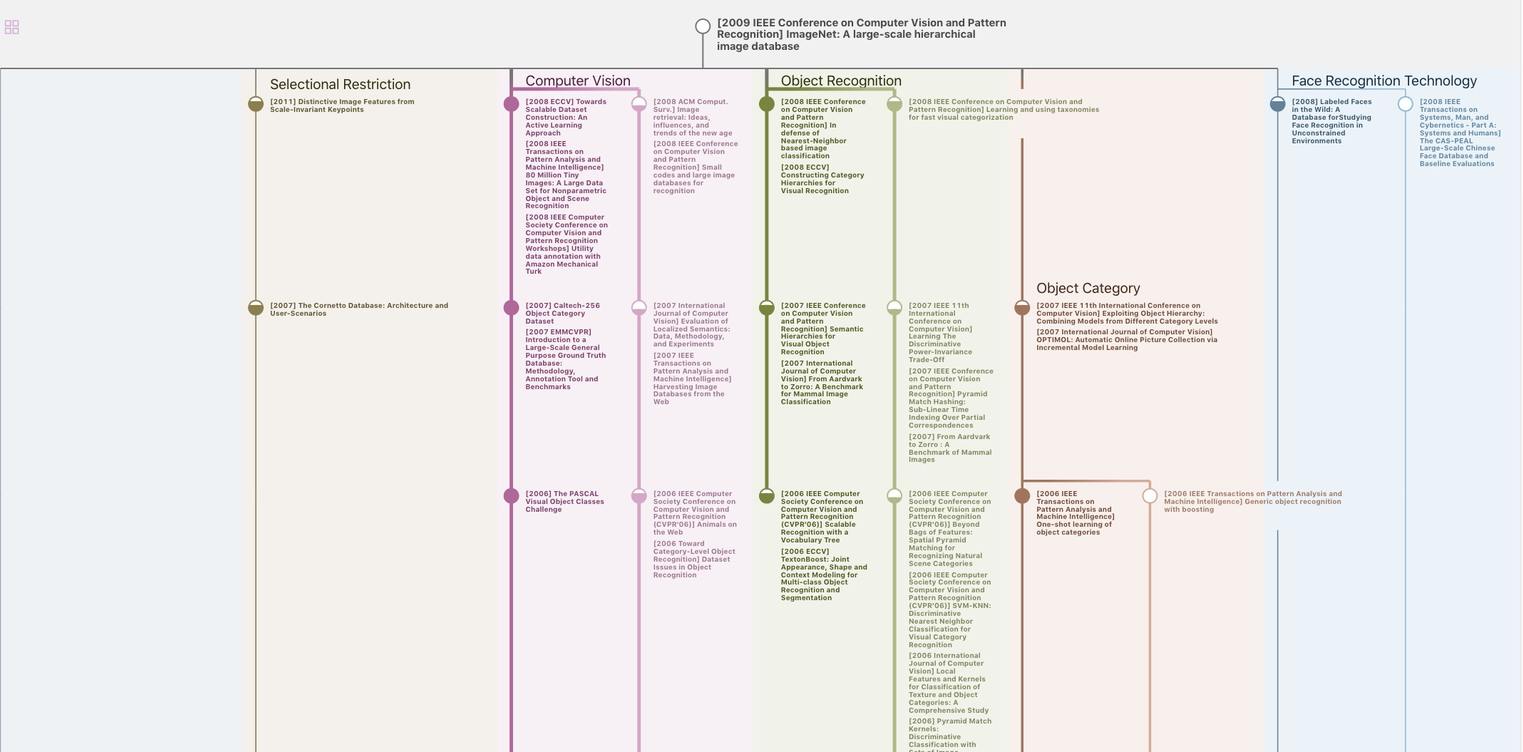
生成溯源树,研究论文发展脉络
Chat Paper
正在生成论文摘要