A Temporal Logic-Based Measurement Framework for Process Mining
2020 2nd International Conference on Process Mining (ICPM)(2020)
摘要
The assessment of behavioral rules with respect to a given dataset is key in several research areas, including declarative process mining, association rule mining, and specification mining. The assessment is required to check how well a set of discovered rules describes the input data, as well as to determine to what extent data complies with predefined rules. In declarative process mining, in particular, some measures have been taken from association rule mining and adapted to support the assessment of temporal rules on event logs. Among them, support and confidence are used most often, yet they are reportedly unable to provide a sufficiently rich feedback to users and often cause spurious rules to be discovered from logs. In addition, these measures are designed to work on a predefined set of rules, thus lacking generality and extensibility. In this paper, we address this research gap by developing a general measurement framework for temporal rules based on Linear-time Temporal Logic with Past on Finite Traces (LTLp
f
). The framework is independent from the rule-specification language of choice and allows users to define new measures. We show that our framework can seamlessly adapt well-known measures of the association rule mining field to declarative process mining. Also, we test our software prototype implementing the framework on synthetic and real-world data, and investigate the properties characterizing those measures in the context of process analysis.
更多查看译文
关键词
Declarative Process Mining,Specification Mining,Association Rule Mining,Quality Measures,Temporal Rules
AI 理解论文
溯源树
样例
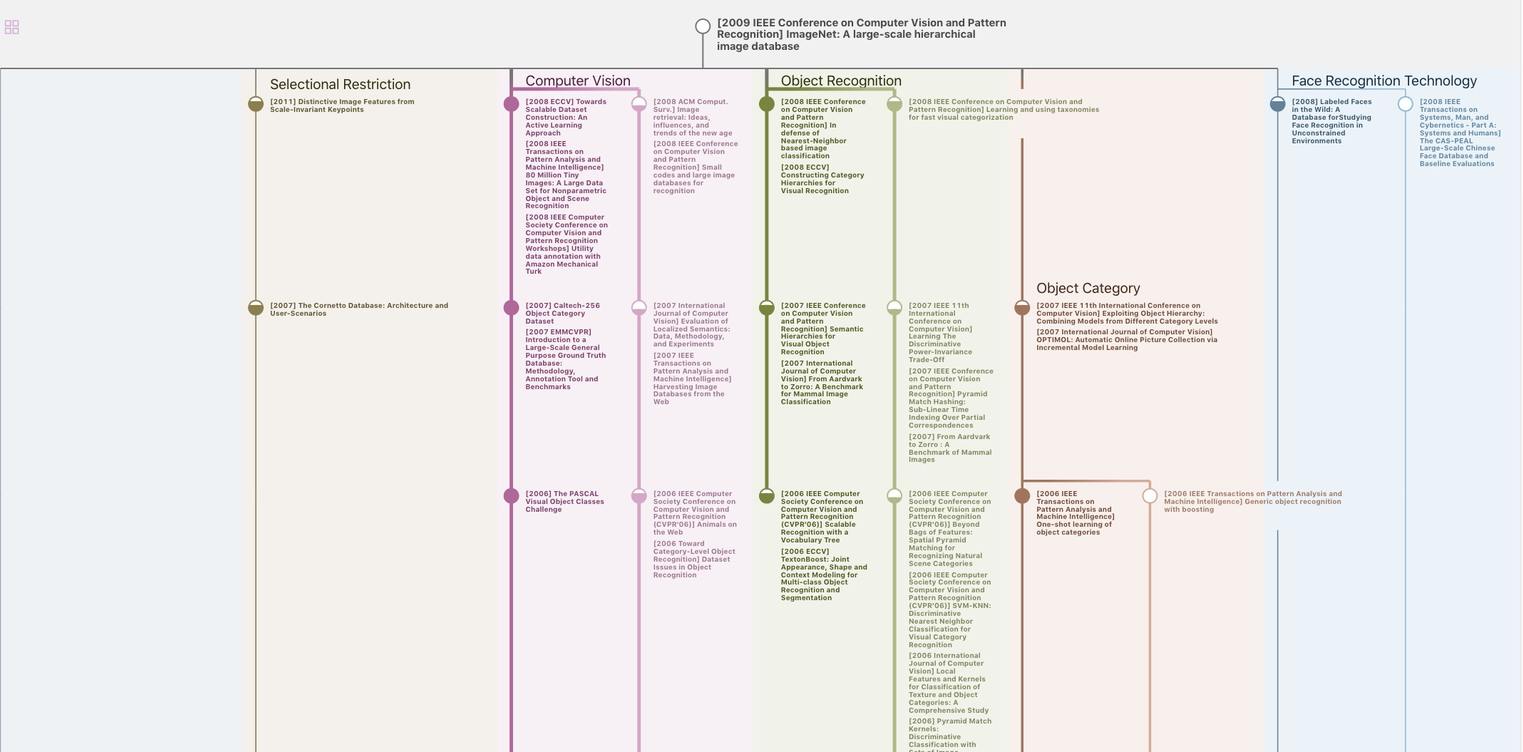
生成溯源树,研究论文发展脉络
Chat Paper
正在生成论文摘要