Adjusting BERT’s Pooling Layer for Large-Scale Multi-Label Text Classification
TEXT, SPEECH, AND DIALOGUE (TSD 2020)(2020)
摘要
In this paper, we present our experiments with BERT models in the task of Large-scale Multi-label Text Classification (LMTC). In the LMTC task, each text document can have multiple class labels, while the total number of classes is in the order of thousands. We propose a pooling layer architecture on top of BERT models, which improves the quality of classification by using information from the standard [CLS] token in combination with pooled sequence output. We demonstrate the improvements on Wikipedia datasets in three different languages using public pre-trained BERT models.
更多查看译文
关键词
Text classification,BERT model
AI 理解论文
溯源树
样例
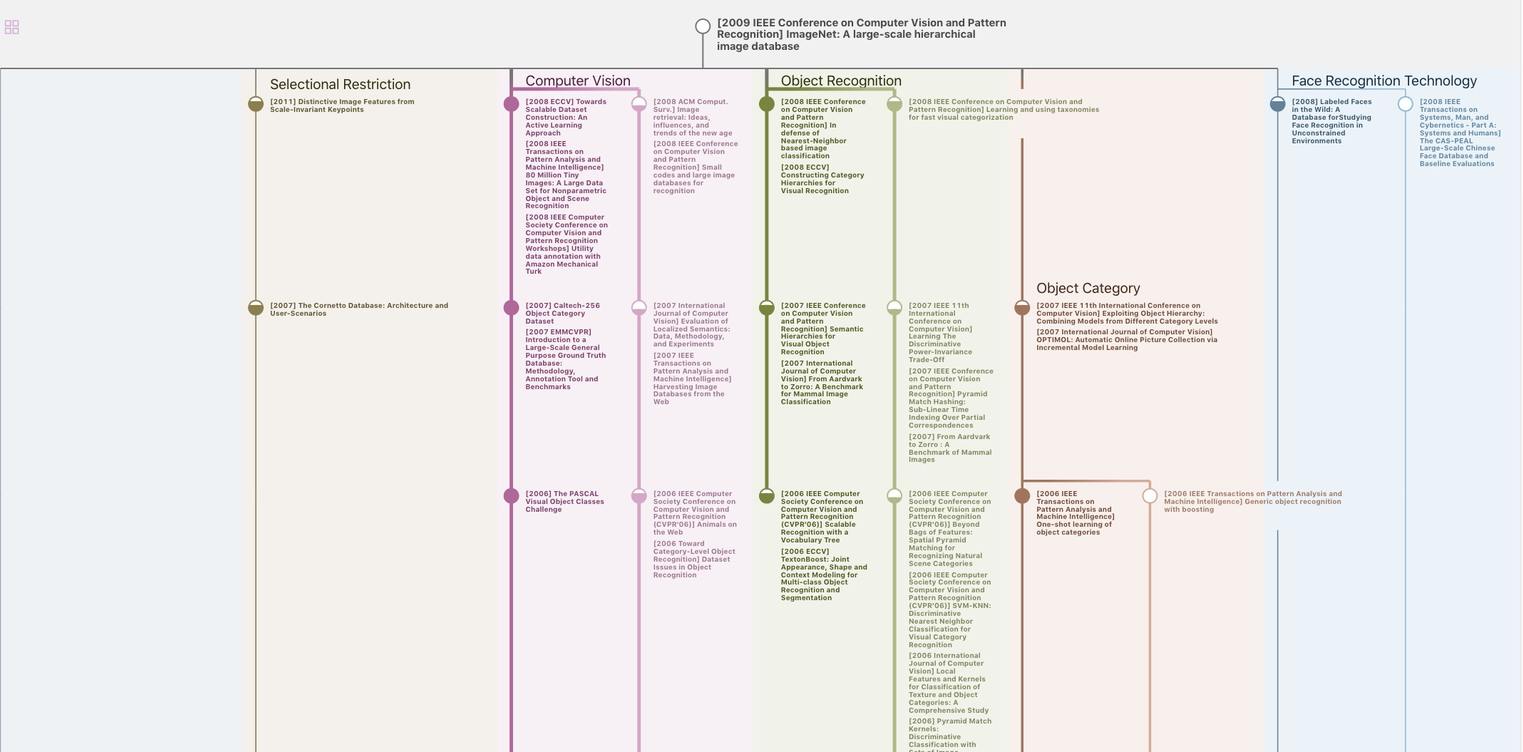
生成溯源树,研究论文发展脉络
Chat Paper
正在生成论文摘要