Evaluation of Structural Hyperparameters for Text Classification with LSTM Networks.
MIPRO(2020)
摘要
In natural language processing, most problems can be interpreted as text classification tasks, which makes this type of task a central one. A natural subdivision of more complex types of text classification can be made according to whether the classification is multilabel or multiclass, where both of these can be tackled with either a multiclass classifier or with a combination of several binary classifiers. An example of the problem which offers a natural way of comparing multiclass and binary classification on the same data, which makes the results comparable, is a classification of text author MBTI personality type. The dataset used is PersonalityCafe MBTI. We focus our comparison on structural hyperparameters, which are the hyperparameters pertaining to network structure. Structural hyperparameters are necessary for specifying the network itself, which makes them a primary concern in its construction. The hyperparameters investigated in this paper are the number of hidden layers and layer size. Through a number of experiments, we demonstrate the choice of hyperparameters and conclude with general hyperparameter selection recommendations based on our results.
更多查看译文
关键词
natural language processing, LSTM, structural hyperparameters, multiclass classification, binary classification
AI 理解论文
溯源树
样例
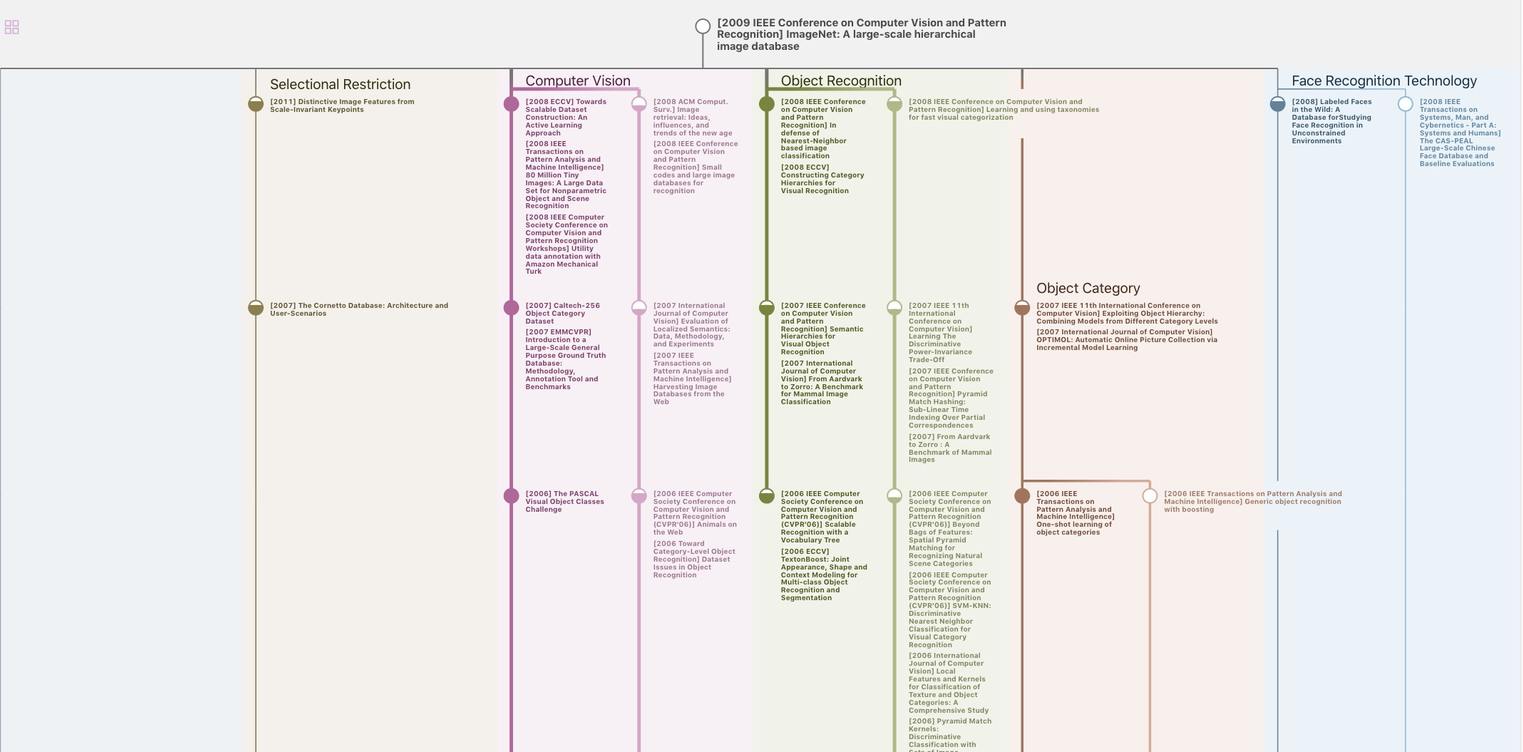
生成溯源树,研究论文发展脉络
Chat Paper
正在生成论文摘要