Neural Networks, Secure by Construction - An Exploration of Refinement Types.
APLAS(2020)
摘要
We present StarChild and Lazuli, two libraries which leverage refinement types to verify neural networks, implemented in F∗ and Liquid Haskell. Refinement types are types augmented, or refined, with assertions about values of that type, e.g., \"integers greater than five\", which are checked by an SMT solver. Crucially, these assertions are written in the language itself. A user of our library can refine the type of neural networks, e.g., \"neural networks which are robust against adversarial attacks\", and expect F∗ to handle the verification of this claim for any specific network, without having to change the representation of the network, or even having to learn about SMT solvers. Our initial experiments indicate that our approach could greatly reduce the burden of verifying neural networks. Unfortunately, they also show that SMT solvers do not scale to the sizes required for neural network verification.
更多查看译文
关键词
Neural networks, Verification, Refinement types
AI 理解论文
溯源树
样例
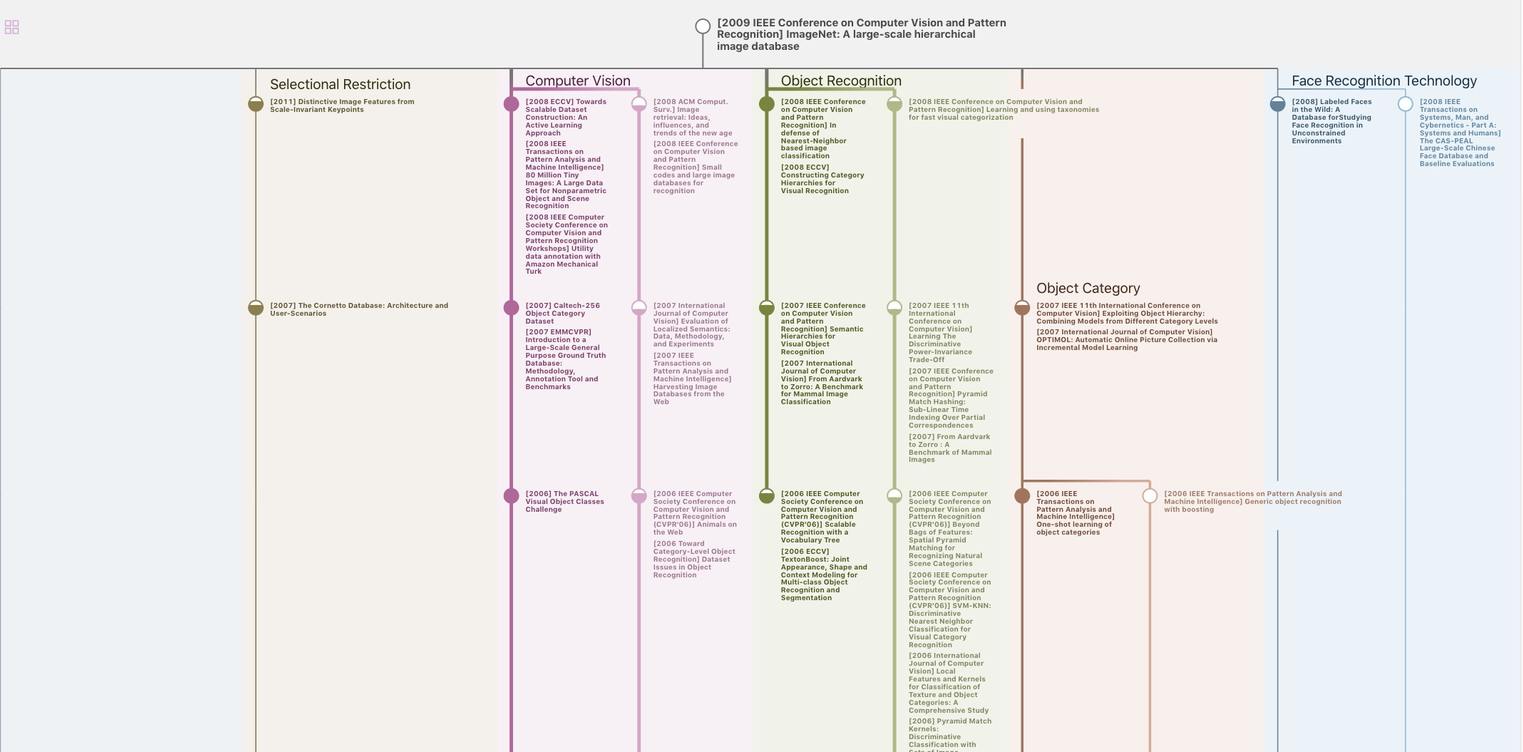
生成溯源树,研究论文发展脉络
Chat Paper
正在生成论文摘要