End-to-End Extraction of Structured Information from Business Documents with Pointer-Generator Networks.
SPNLP@EMNLP(2020)
摘要
The predominant approaches for extracting key information from documents resort to classifiers predicting the information type of each word. However, the word level ground truth used for learning is expensive to obtain since it is not naturally produced by the extraction task. In this paper, we discuss a new method for training extraction models directly from the textual value of information. The extracted information of a document is represented as a sequence of tokens in the XML language. We learn to output this representation with a pointer-generator network that alternately copies the document words carrying information and generates the XML tags delimiting the types of information. The ability of our end-to-end method to retrieve structured information is assessed on a large set of business documents. We show that it performs competitively with a standard word classifier without requiring costly word level supervision.
更多查看译文
关键词
structured information,business documents,end-to-end,pointer-generator
AI 理解论文
溯源树
样例
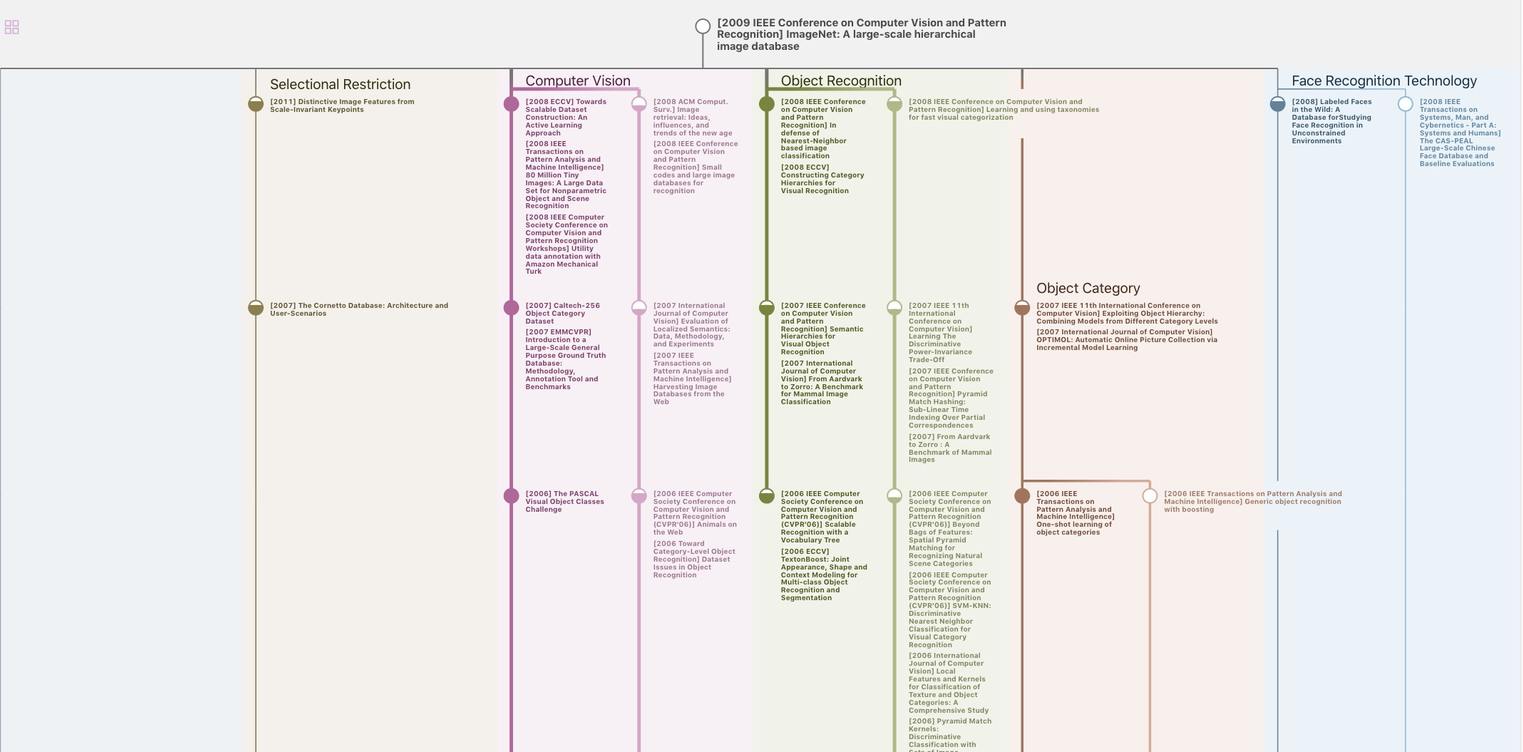
生成溯源树,研究论文发展脉络
Chat Paper
正在生成论文摘要