Deep Plug-and-Play Video Super-Resolution.
ECCV Workshops(2020)
摘要
Video super-resolution (VSR) has been drawing increasing research attention due to its wide practical applications. Despite the unprecedented success of deep single image super-resolution (SISR), recent deep VSR methods devote much effort to designing modules for spatial alignment and feature fusion of multiple adjacent frames while failing to leverage the progress in SISR. In this paper, we propose a plug-and-play VSR framework, through which the state-of-the-art SISR models can be readily employed without re-training, and the proposed temporal consistency refinement network (TCRNet) can enhance the temporal consistency and visual quality. In particular, an SISR model is firstly adopted to super-resolve low-resolution video in a frame-by-frame manner. Instead of using multiple frames, our TCRNet only takes two adjacent frames as input. To alleviate the issue of spatial misalignments, we present an iterative residual refinement module for motion offset estimation. Furthermore, a deformable convolutional LSTM is proposed to exploit long-distance temporal information. The proposed TCRNet can be easily and stably trained using \(\ell _2\) loss function. Moreover, the VSR performance is further boosted by a bidirectional process. On popular benchmark datasets, our TCRNet can significantly enhance the temporal consistency when collaborating with various SISR models, and is superior to or at least on par with state-of-the-art VSR methods in terms of quantitative metrics and visually quality.
更多查看译文
关键词
Deep learning,Video super-resolution,Single image super-resolution,CNN,LSTM
AI 理解论文
溯源树
样例
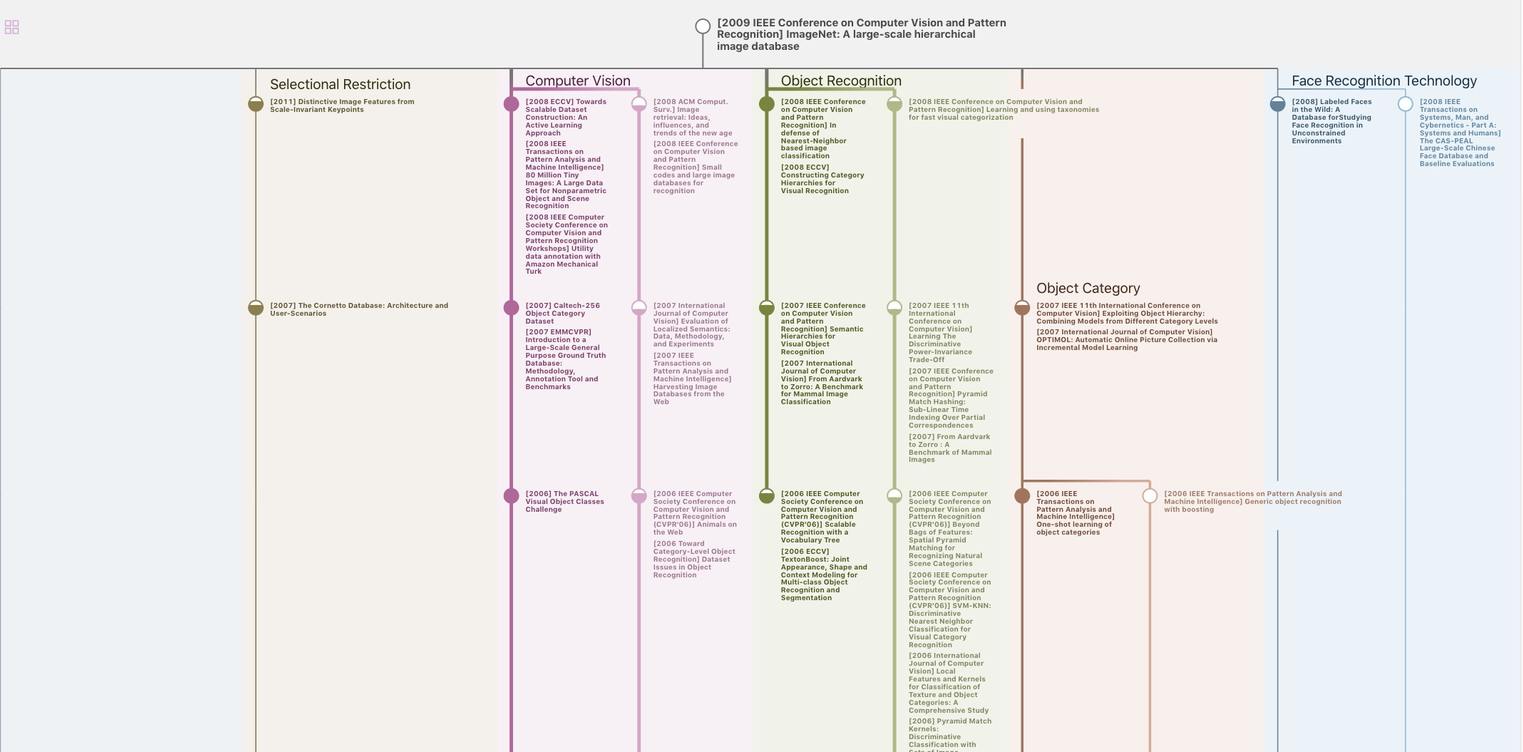
生成溯源树,研究论文发展脉络
Chat Paper
正在生成论文摘要