3D Noise and Adversarial Supervision Is All You Need for Multi-modal Semantic Image Synthesis.
ECCV Workshops(2020)
摘要
Semantic image synthesis models suffer from training instabilities and poor image quality when trained with adversarial supervision alone. Historically, this was alleviated via an additional VGG-based perceptual loss. Hence, we propose a new simplified GAN model, which needs only adversarial supervision to achieve high-quality results. In doing so, we also show that the VGG supervision decreases image diversity and can hurt image quality. We achieve the improvement by re-designing the discriminator as a semantic segmentation network. The resulting stronger supervision makes the VGG loss obsolete. Moreover, in contrast to previous work, we enable high-quality multi-modal image synthesis through a novel noise sampling scheme. Compared to the state of the art, we achieve an average improvement of 6 FID and 7 mIoU.
更多查看译文
关键词
adversarial supervision,3d,synthesis,multi-modal
AI 理解论文
溯源树
样例
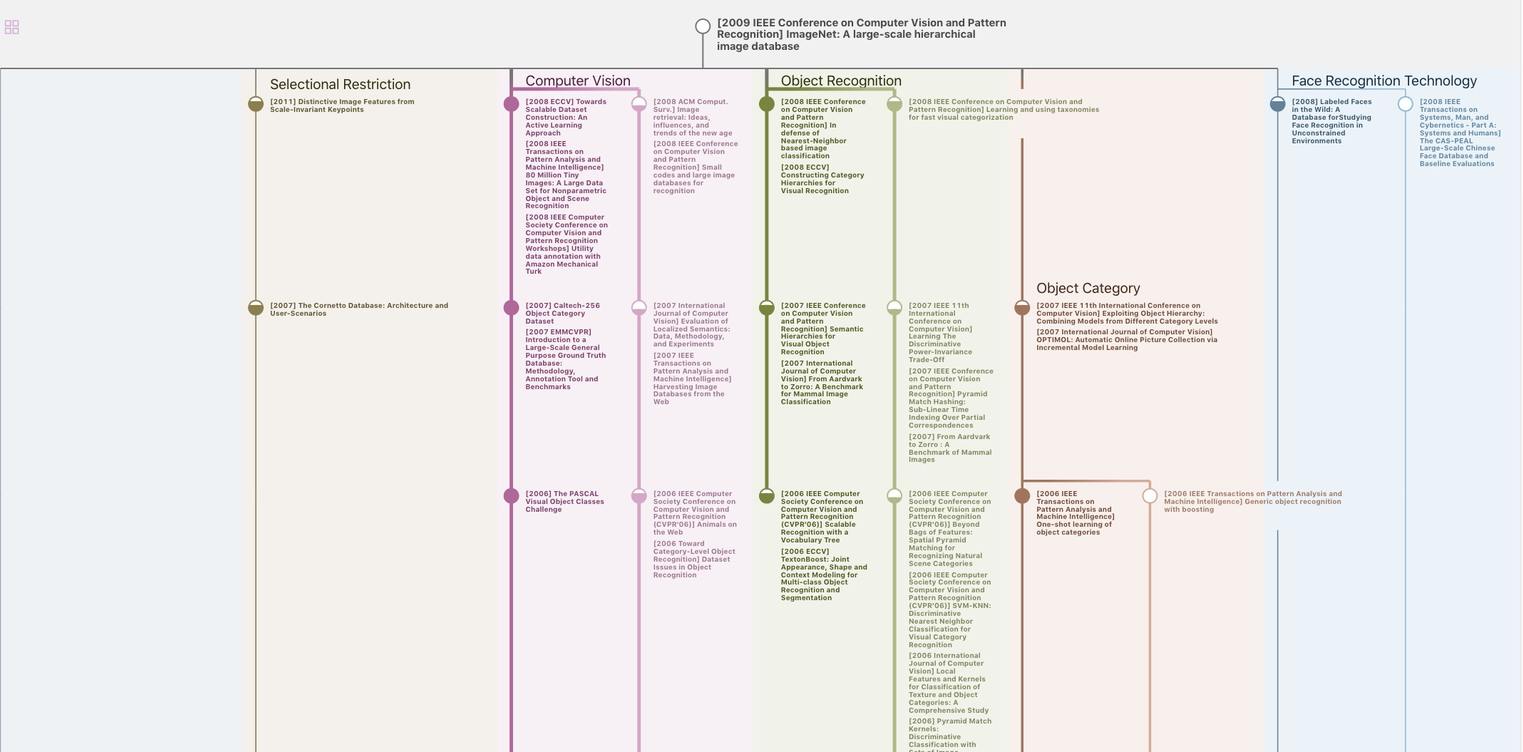
生成溯源树,研究论文发展脉络
Chat Paper
正在生成论文摘要