Uncertainty Quantification for Text Classification
PROCEEDINGS OF THE 46TH INTERNATIONAL ACM SIGIR CONFERENCE ON RESEARCH AND DEVELOPMENT IN INFORMATION RETRIEVAL, SIGIR 2023(2023)
摘要
This full-day tutorial introduces modern techniques for practical uncertainty quantification specifically in the context of multi-class and multi-label text classification. First, we explain the usefulness of estimating aleatoric uncertainty and epistemic uncertainty for text classification models. Then, we describe several state-of-the-art approaches to uncertainty quantification and analyze their scalability to big text data: Virtual Ensemble in GBDT, Bayesian Deep Learning (including Deep Ensemble, Monte-Carlo Dropout, Bayes by Backprop, and their generalization Epistemic Neural Networks), Evidential Deep Learning (including Prior Networks and Posterior Networks), as well as Distance Awareness (including Spectral-normalized Neural Gaussian Process and Deep Deterministic Uncertainty). Next, we talk about the latest advances in uncertainty quantification for pre-trained language models (including asking language models to express their uncertainty, interpreting uncertainties of text classifiers built on large-scale language models, uncertainty estimation in text generation, calibration of language models, and calibration for in-context learning). After that, we discuss typical application scenarios of uncertainty quantification in text classification (including in-domain calibration, cross-domain robustness, and novel class detection). Finally, we list popular performance metrics for the evaluation of uncertainty quantification effectiveness in text classification. Practical hands-on examples/exercises are provided to the attendees for them to experiment with different uncertainty quantification methods on a few real-world text classification datasets such as CLINC150.
更多查看译文
关键词
uncertainty quantification,text classification,language models
AI 理解论文
溯源树
样例
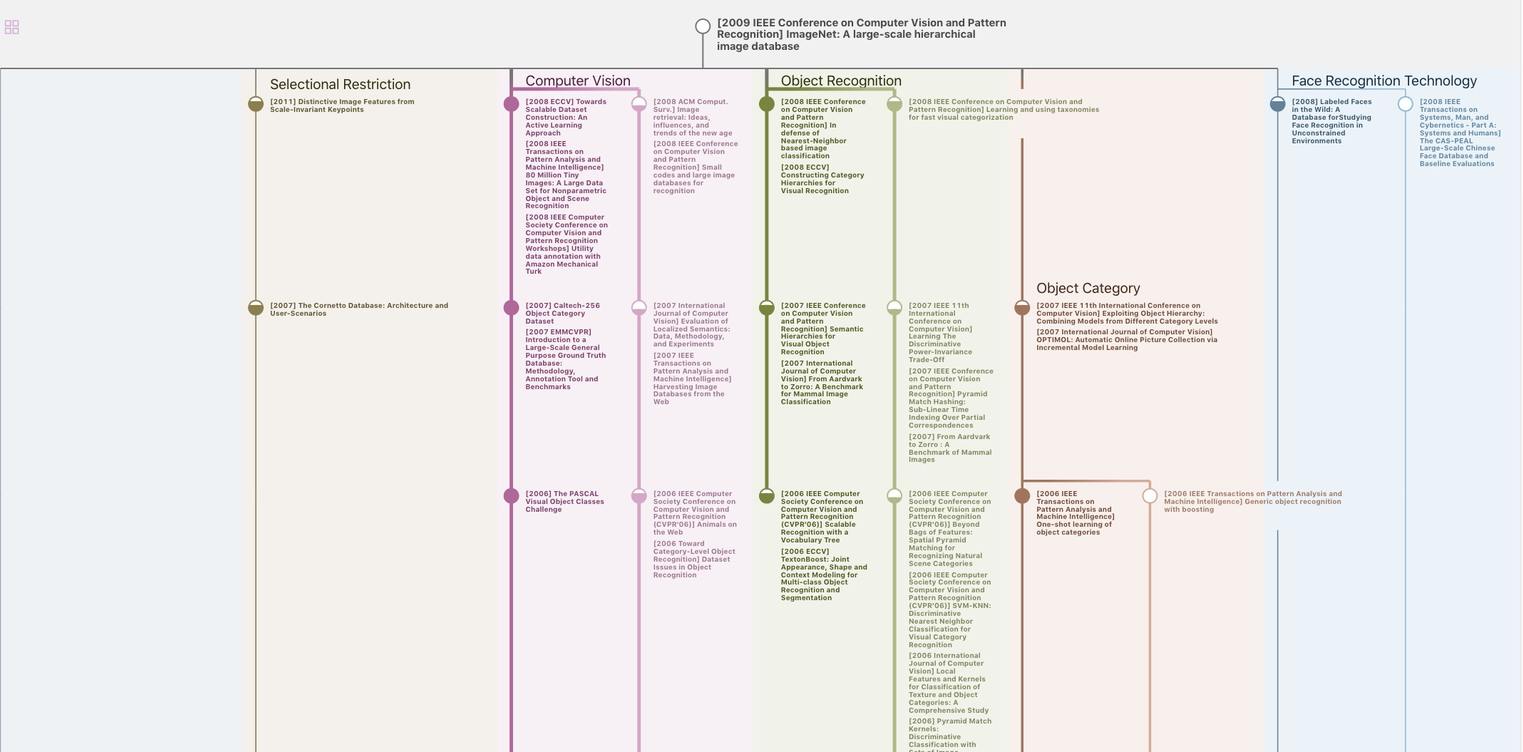
生成溯源树,研究论文发展脉络
Chat Paper
正在生成论文摘要