Block-Aware Item Similarity Models for Top-N Recommendation
ACM Transactions on Information Systems(2020)
摘要
AbstractTop-N recommendations have been studied extensively. Promising results have been achieved by recent item-based collaborative filtering (ICF) methods. The key to ICF lies in the estimation of item similarities. Observing the block-diagonal structure of the item similarities in practice, we propose a block-diagonal regularization (BDR) over item similarities for ICF. The intuitions behind BDR are as follows: (1) with BDR, item clustering is embedded into the learning of ICF methods; (2) BDR induces sparsity of item similarities, which guarantees recommendation efficiency; and (3) BDR captures in-block transitivity to overcome rating sparsity. By regularizing the item similarity matrix of item similarity models with BDR, we obtain a block-aware item similarity model. Our experimental evaluations on a large number of datasets show that the block-diagonal structure is crucial to the performance of top-N recommendation.
更多查看译文
关键词
Item collaborative filtering, item similarity model, top-N recommendation
AI 理解论文
溯源树
样例
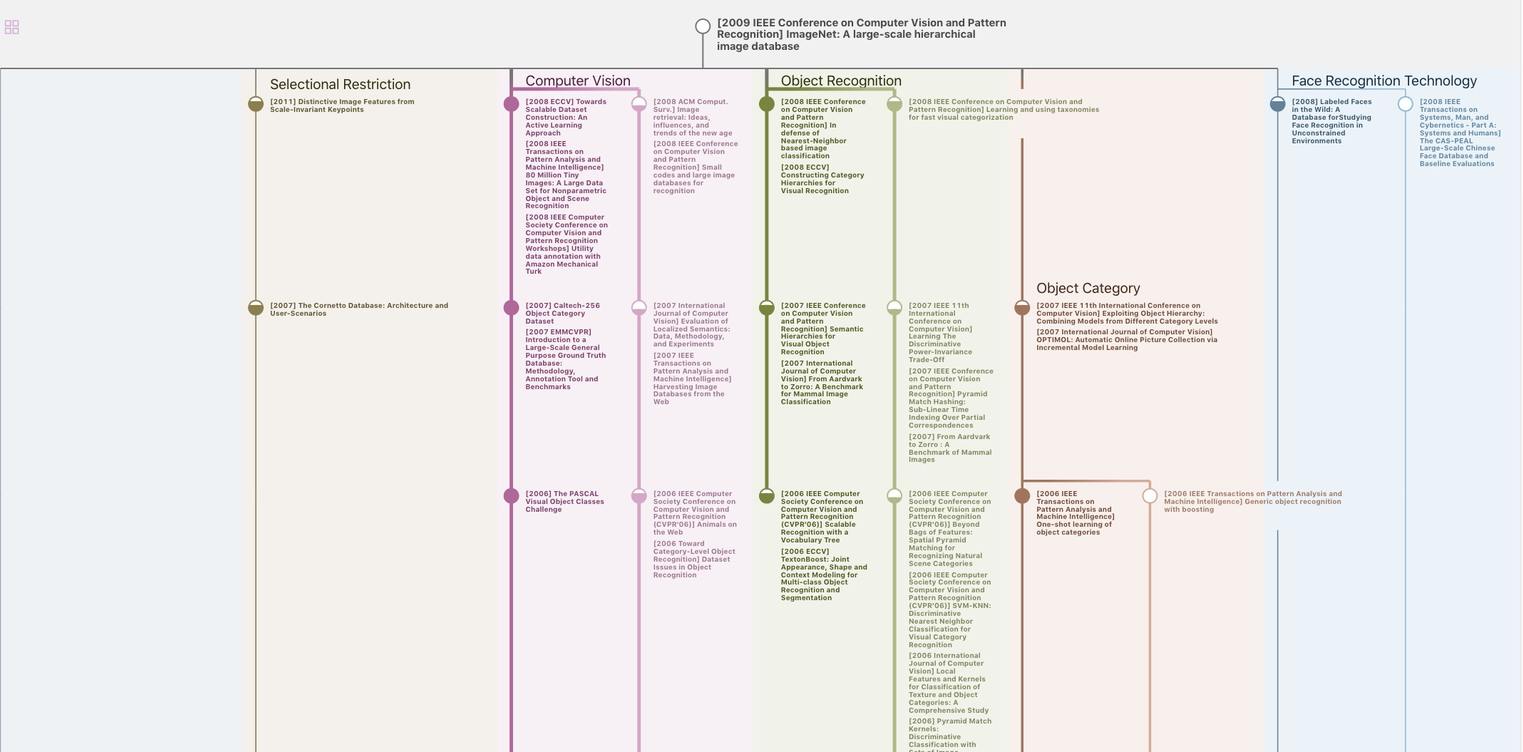
生成溯源树,研究论文发展脉络
Chat Paper
正在生成论文摘要