Impact Of Injection Attacks On Sensor-Based Continuous Authentication For Smartphones
COMPUTER COMMUNICATIONS(2020)
摘要
Given the relevance of smartphones for accessing personalized services in smart cities, Continuous Authentication (CA) mechanisms are attracting attention to avoid impersonation attacks. Some of them leverage Data Stream Mining (DSM) techniques applied over sensorial information. Injection attacks can undermine the effectiveness of DSM-based CA by fabricating artificial sensorial readings.The goal of this paper is to study the impact of injection attacks in terms of accuracy and immediacy to illustrate the time the adversary remains unnoticed. Two well-known DSM techniques (K-Nearest Neighbours and Hoeffding Adaptive Trees) and three data sources (location, gyroscope and accelerometer) are considered due to their widespread usage Results show that even if the attacker does not previously know anything about the victim, a significant attack surface arises - 1.35 min are needed, in the best case, to detect the attack on gyroscope and accelerometer and 7.27 min on location data. Moreover, we show that the type of sensor at stake and configuration settings may have a dramatic effect on countering this threat.
更多查看译文
关键词
Continuous Authentication (CA), Injection attacks, Data Stream Mining, Smartphones
AI 理解论文
溯源树
样例
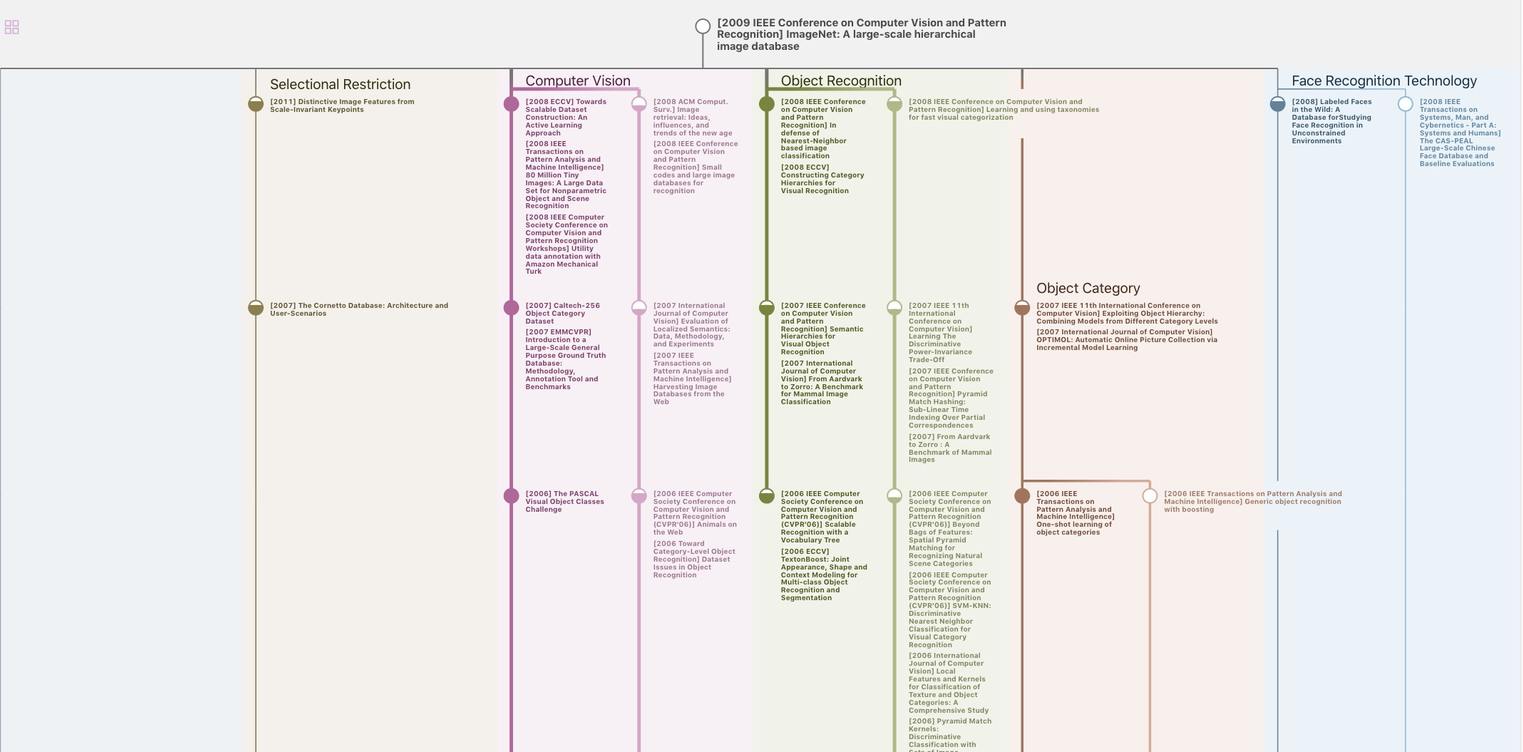
生成溯源树,研究论文发展脉络
Chat Paper
正在生成论文摘要