Eeg Signals Analysis For Epileptic Seizures Detection Using Polynomial Transforms, Linear Discriminant Analysis And Support Vector Machines
BIOMEDICAL SIGNAL PROCESSING AND CONTROL(2020)
摘要
Electroencephalogram (EEG) signals are useful in understanding the human brain diseases like epilepsy which is characterized by an enduring predisposition to generate epileptic seizures and by neurologic, cognitive, psychological and social consequences of these conditions. That is why this paper proposed a novel full processing chain of EEG signals analysis for epileptic seizures detection that applied a new alternative approach for EEG rhythms decomposition. EEG signals are decomposed into their different background rhythms using discrete Jacobi polynomial transforms (JPTs) and a 28-dimension feature vector is extracted in frequency and time domains. Due to the high dimension and redundancy of these features, the linear discriminant analysis (LDA) is applied for dimensionality reduction and informative and discriminative low dimension feature vectors are computed and feed as inputs of the support vector machines (SVM) classifiers. The validation of this processing chain is done experimentally using an online available database which consists of five hundred EEG signals. Analysis demonstrated that the EEG rhythms decomposition using JPTs can be implemented as a single-step process and provides more abundant information for class discrimination. In addition, twelve experiments closely related to clinical applications are examined and promising results are achieved with maximum accuracies between 96.25-100 %. Overall, it is found that the proposed processing chain will be useful in providing an accurate and objective scheme for automatic EEG rhythms decomposition and epileptic seizures detection that can be integrated into implantable devices intended to predict the onset of seizures and trigger a focal treatment to block the seizures progression.
更多查看译文
关键词
Epileptic seizures detection, Rhythms decomposition, Jacobi polynomial transforms (JPTs), Low dimension feature vectors, Linear discriminant analysis (LDA), Support vector machines (SVM)
AI 理解论文
溯源树
样例
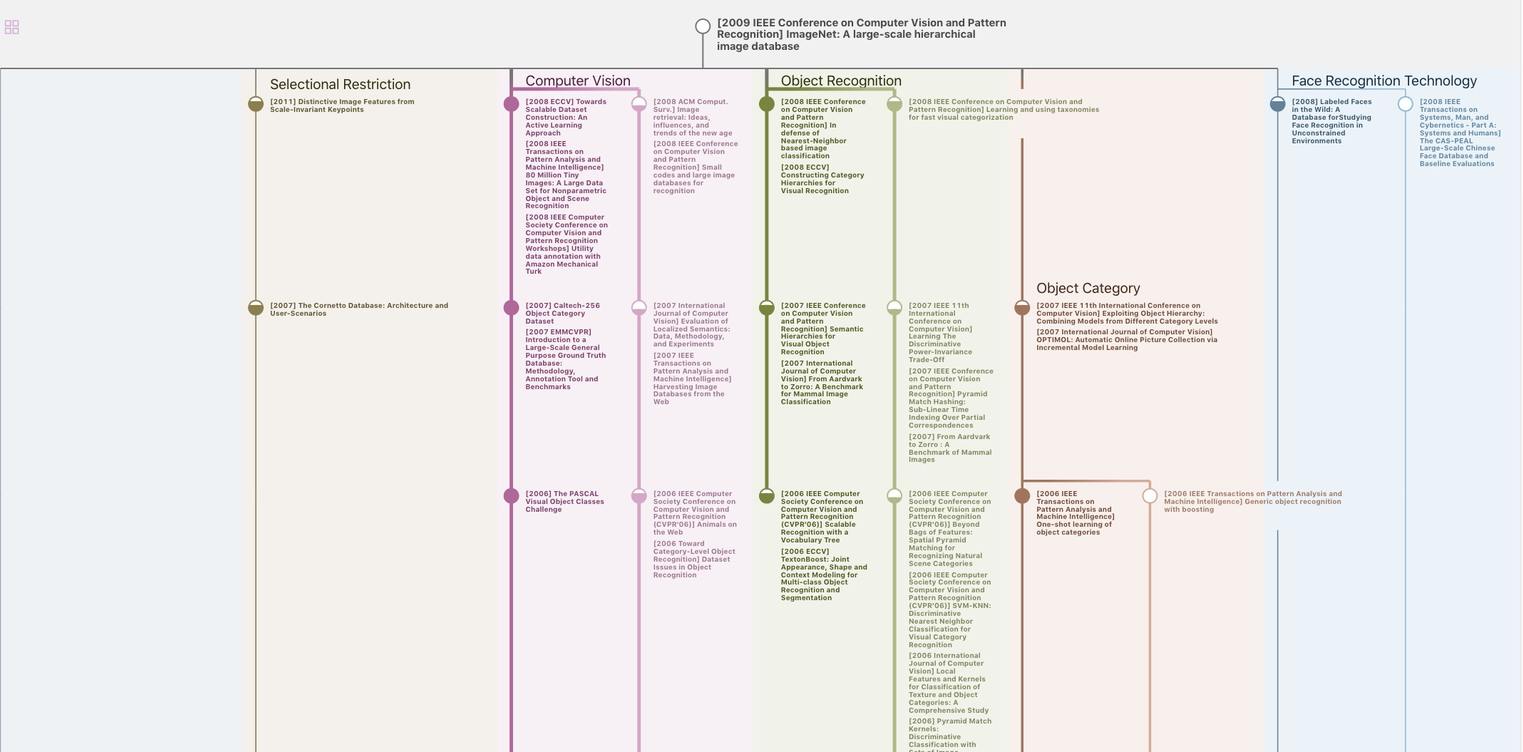
生成溯源树,研究论文发展脉络
Chat Paper
正在生成论文摘要