AONet: Active Offset Network for crowd flow prediction
Engineering Applications of Artificial Intelligence(2021)
摘要
Predicting crowd flow is of great importance to public safety and traffic management. The crowd flow is difficult to predict accurately and timely due to the uncertainty of the future positions. In this paper, we propose a novel Active Offset Network (AONet), in which ActiveGRU (Active Gate Recurrent Unit) is designed to predict the variation of pedestrians’ positions in the crowd flow. Its inner location-variant recurrent structure is implemented by utilizing convolution operation on low dimensional spatio-temporal sequences to obtain fractional offset locations. Afterwards, the sampling locations are determined by bilinear interpolation on fractional offset locations. Moreover, a probabilistic sparse strategy is introduced to reduce the links between sampling locations during supervised training. Finally, the experiments over popular benchmarks demonstrate that our method can actively characterize the future positions of pedestrians. Meanwhile, the performance of the proposed AONet is superior over state-of-art baselines with regard to both accuracy and computational savings.
更多查看译文
关键词
Crowd flow,Bilinear interpolation,Location-variant,Probabilistic sparse
AI 理解论文
溯源树
样例
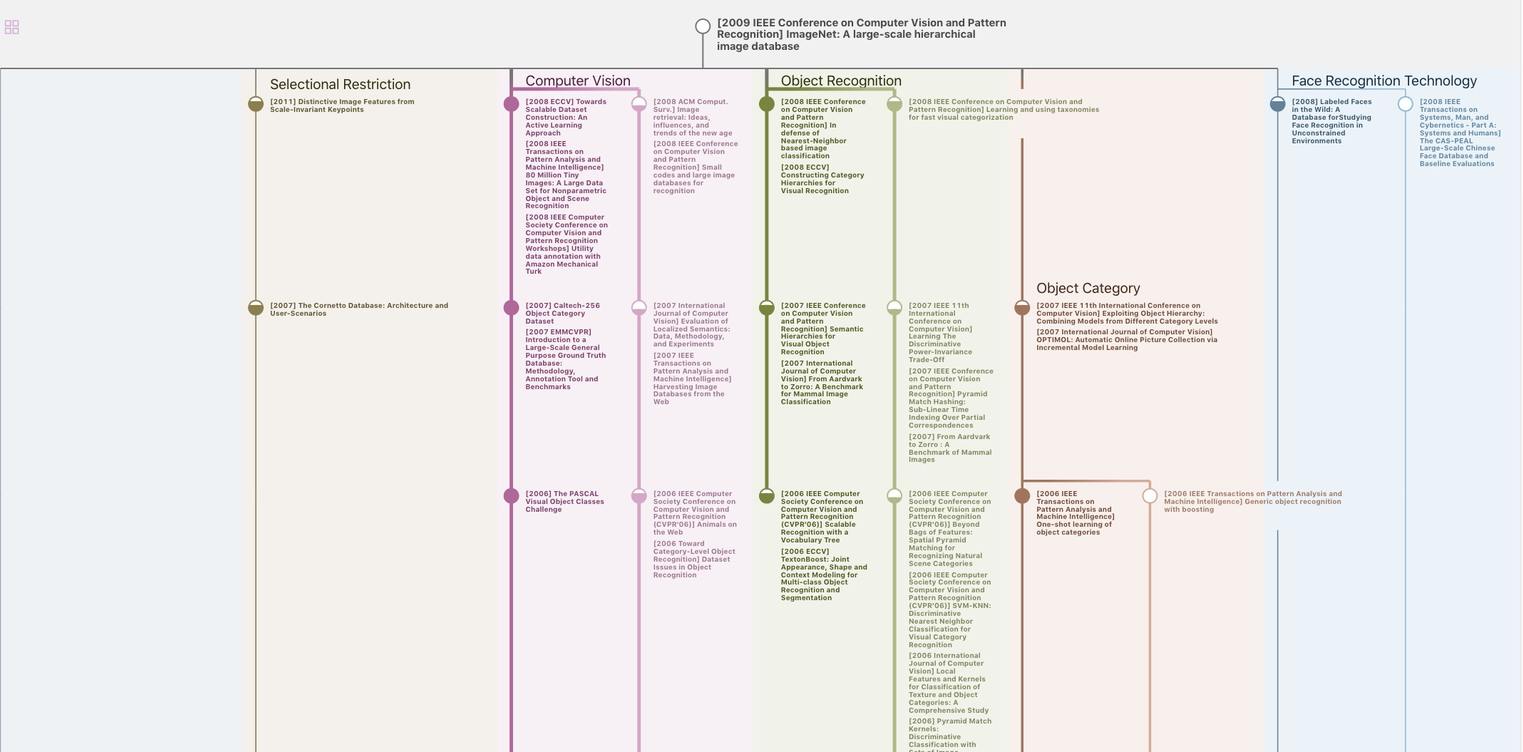
生成溯源树,研究论文发展脉络
Chat Paper
正在生成论文摘要