Corner Case Data Description and Detection
2021 IEEE/ACM 1st Workshop on AI Engineering - Software Engineering for AI (WAIN)(2021)
摘要
As the major factors affecting the safety of deep learning models, corner cases and related detection are crucial in AI quality assurance for constructing safety- and security-critical systems. The generic corner case researches involve two interesting topics. One is to enhance DL models' robustness to corner case data via the adjustment on parameters/structure. The other is to generate new corner cases for model retraining and improvement. However, the complex architecture and the huge amount of parameters make the robust adjustment of DL models not easy, meanwhile it is not possible to generate all real-world corner cases for DL training. Therefore, this paper proposes a simple and novel approach aiming at corner case data detection via a specific metric. This metric is developed on surprise adequacy (SA) which has advantages on capture data behaviors. Furthermore, targeting at characteristics of corner case data, three modifications on distanced-based SA are developed for classification applications in this paper. Consequently, through the experiment analysis on MNIST data and industrial data, the feasibility and usefulness of the proposed method on corner case data detection are verified.
更多查看译文
关键词
corner case data detection,surprise adequacy,modified distanced-based SA,AI quality testing
AI 理解论文
溯源树
样例
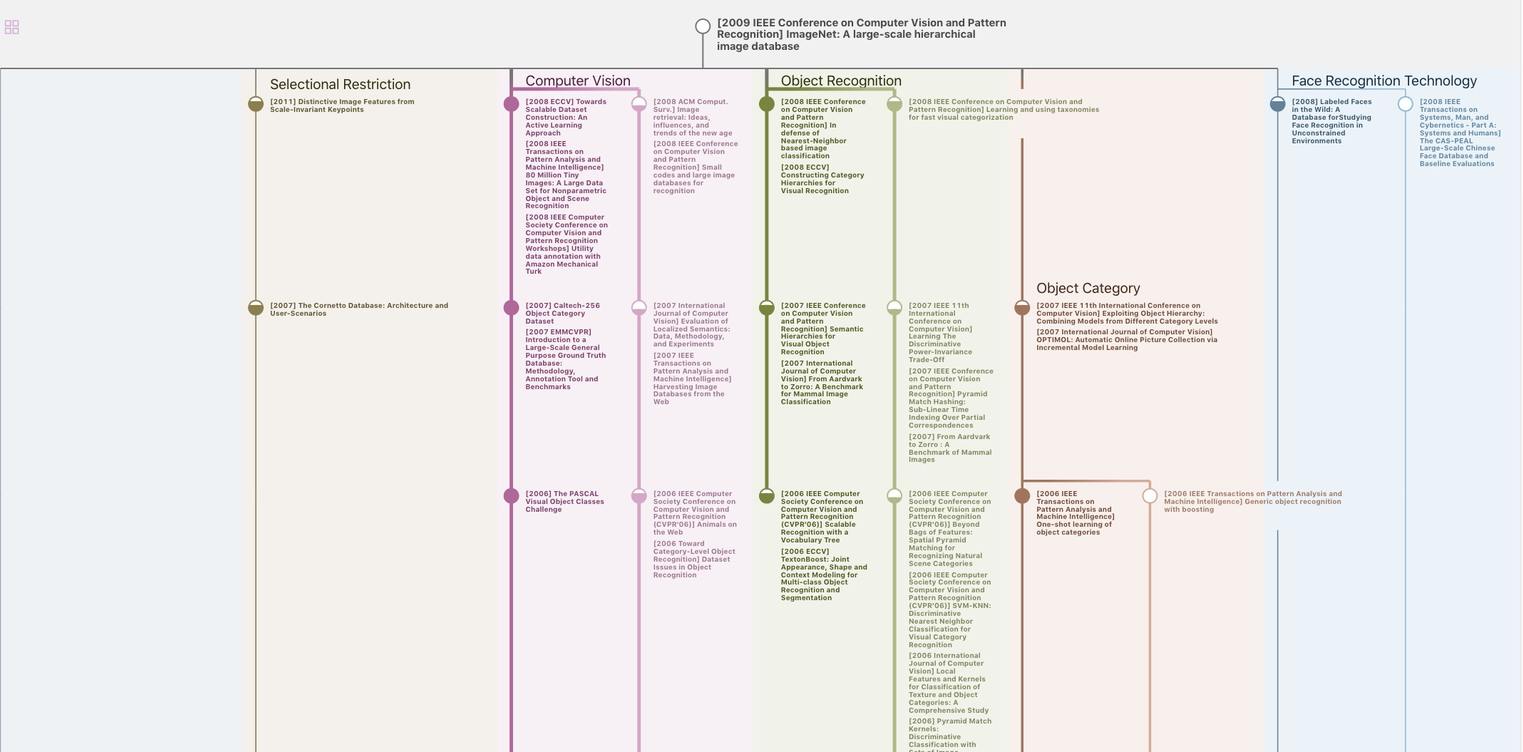
生成溯源树,研究论文发展脉络
Chat Paper
正在生成论文摘要