Leveraging active learning to reduce human effort in the generation of ground-truth for entity resolution.
COMPUTATIONAL INTELLIGENCE(2020)
摘要
Several methods of entity resolution (ER) have been developed in academia and industry over the years, with the intention to identify duplicate entities (eg, records) in datasets. To evaluate the efficacy of such methods, it is necessary to compare their results with a ground-truth, which consists of a document containing all known duplicate record pairs in a dataset. In general, the generation of ground-truths for real datasets is performed manually from the inspection of all combinations of pairs of records in a dataset. This is subject to error and presents quadratic complexity, with respect to the size(s) of the dataset(s), requiring a long time to be performed. In this context, some works present (semi)automatic approaches for the generation of ground-truths for the ER task. However, such approaches are either not applicable to several domains or still present a considerable manual effort. In this work, we propose GTGenERAL, a semiautomatic approach that combines results from multiple algorithms of ER together with active learning to generate accurate ground-truths employing reduced manual effort. Experiments using real datasets show that, with great manual effort reduction, GTGenERAL is able to generate ground-truths close to those generated by the state-of-the-art approach.
更多查看译文
关键词
active learning,classification,deduplication,ground-truth,machine learning,record linkage
AI 理解论文
溯源树
样例
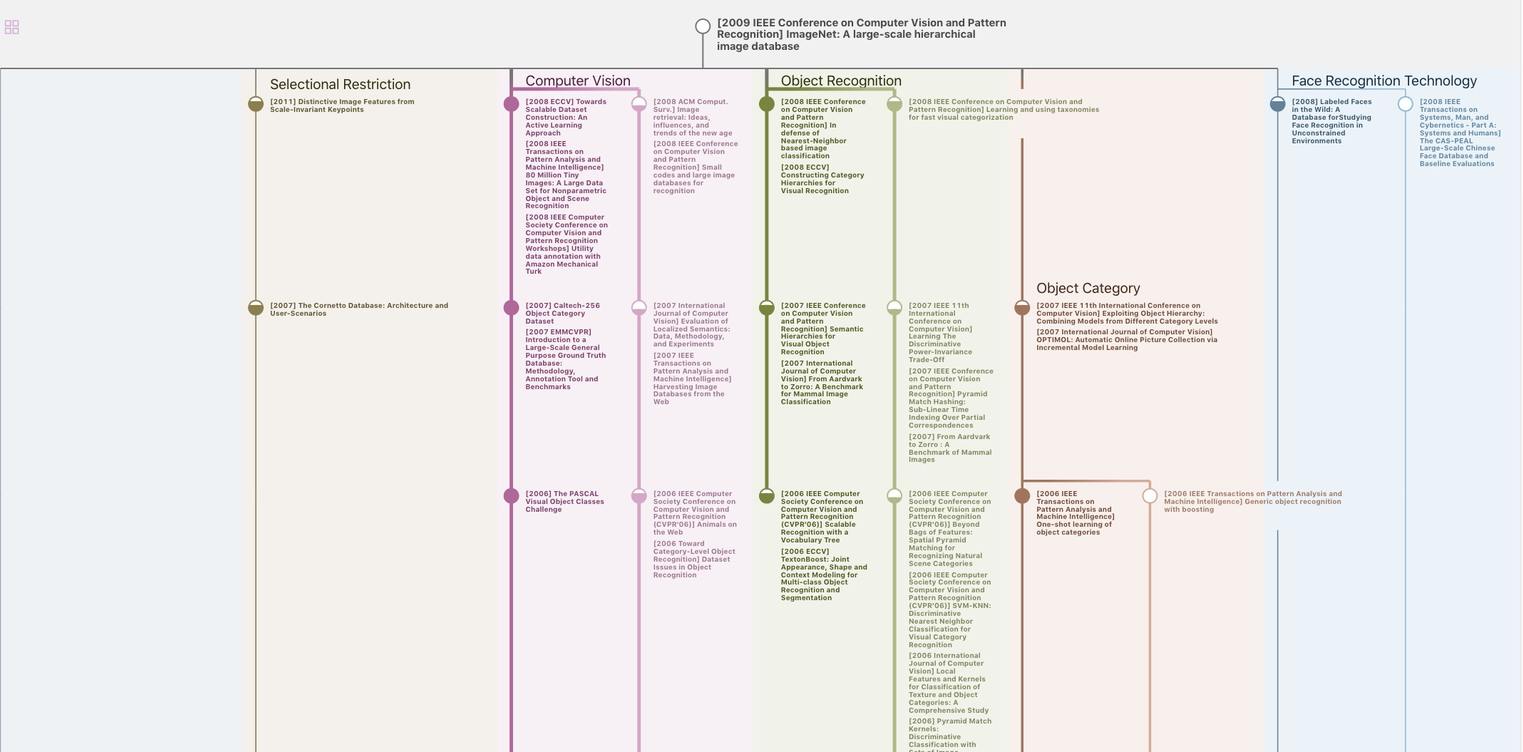
生成溯源树,研究论文发展脉络
Chat Paper
正在生成论文摘要