Multi-stage hybrid embedding fusion network for visual question answering
Neurocomputing(2021)
摘要
Multimodal fusion is a crucial component of Visual Question Answering (VQA), which involves joint understanding and semantic integration between visual and textual information. Existing VQA learning frameworks focus mainly on Latent Embedding Fusion (LEF) method, by projecting visual and textual features into a common latent space, and fusing them with element-wise multiplication. In this paper, we intend to achieve multiple and fine-grained multimodal interactions for enhancing fusion performance. To this end, we propose a Multi-stage Hybrid Embedding Fusion (MHEF) network to fulfill our improvements in two folds: First, we introduce a Dual Embedding Fusion (DEF) approach that transforms one modal input into the reciprocal embedding space before integration, and the DEF is further incorporated with the LEF to form a novel Hybrid Embedding Fusion (HEF). Second, we design a Multi-stage Fusion Structure (MFS) for the HEF to form the MHEF network, so as to obtain diverse and better fusion features for answer prediction. By jointly training the multi-stage framework, we can not only improve the performance in each single stage, but also obtain additional accuracy improvements by integrating all prediction results from each stage. Extensive experiments verify both our proposed HEF and MFS are beneficial to multi-modal fusion. The full MHEF model outperforms the baseline LEF model with 2% accuracy improvements, and achieves promising performance on the VQA-v1 and VQA-v2 datasets.
更多查看译文
关键词
Visual Question Answering,Multimodel Embedding,Multi-Stage Fusion
AI 理解论文
溯源树
样例
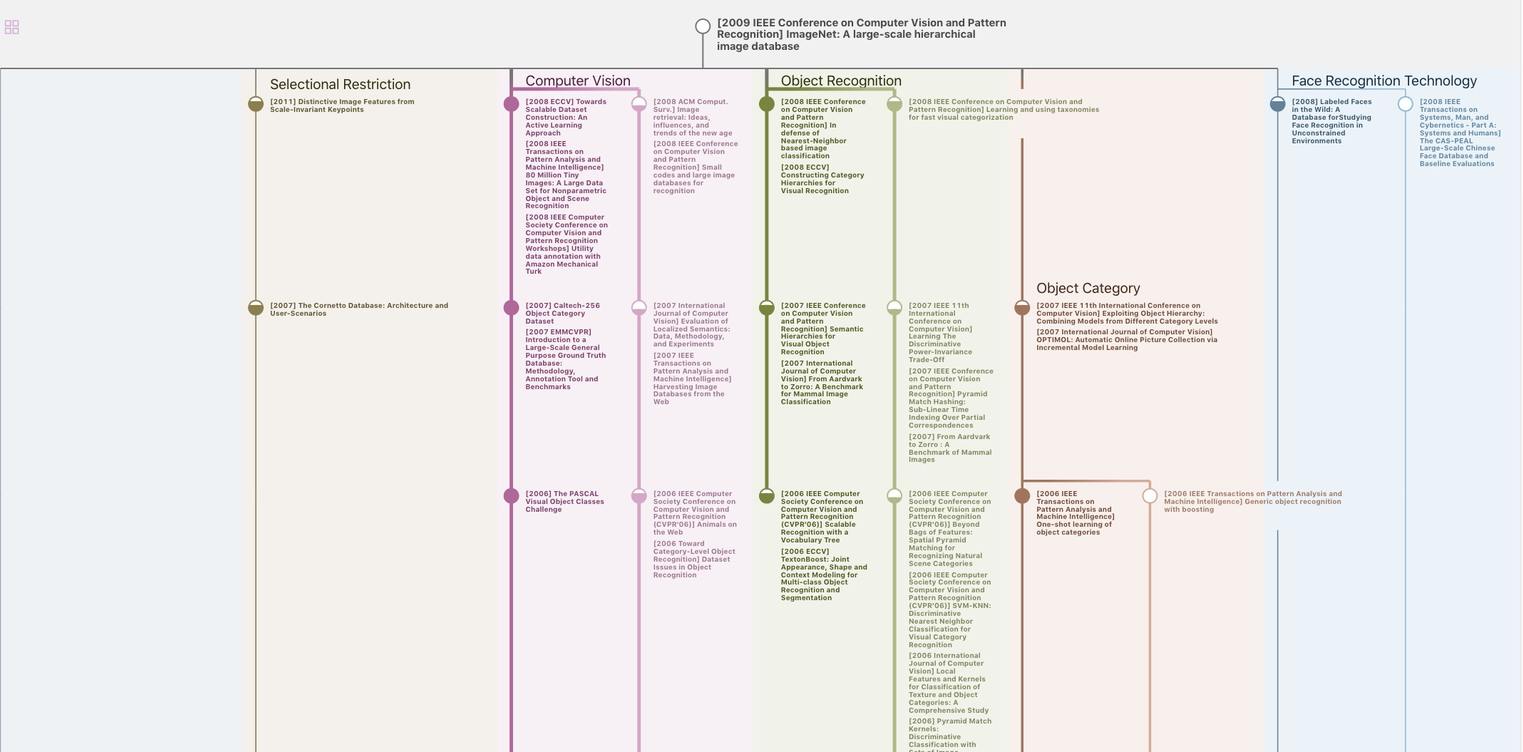
生成溯源树,研究论文发展脉络
Chat Paper
正在生成论文摘要