CentroidNetV2: A hybrid deep neural network for small-object segmentation and counting
Neurocomputing(2021)
摘要
This paper presents CentroidNetV2, a novel hybrid Convolutional Neural Network (CNN) that has been specifically designed to segment and count many small and connected object instances. This complete redesign of the original CentroidNet uses a CNN backbone to regress a field of centroid-voting vectors and border-voting vectors. The segmentation masks of the individual object instances are produced by decoding centroid votes and border votes. A loss function that combines cross-entropy loss and Euclidean-distance loss achieves high quality centroids and borders of object instances. Several backbones and loss functions are tested on three different datasets ranging from precision agriculture to microbiology and pathology. CentroidNetV2 is compared to the state-of-the art networks You Only Look Once Version 3 (YOLOv3) and Mask Recurrent Convolutional Neural Network (MRCNN). On two out of three datasets CentroidNetV2 achieves the highest F1 score and on all three datasets CentroidNetV2 achieves the highest recall. CentroidNetV2 demonstrates the best ability to detect small objects although the best segmentation masks for larger objects are produced by MRCNN.
更多查看译文
关键词
Deep Learning,Computer Vision,Convolutional Neural Networks,Object Detection,Instance Segmentation
AI 理解论文
溯源树
样例
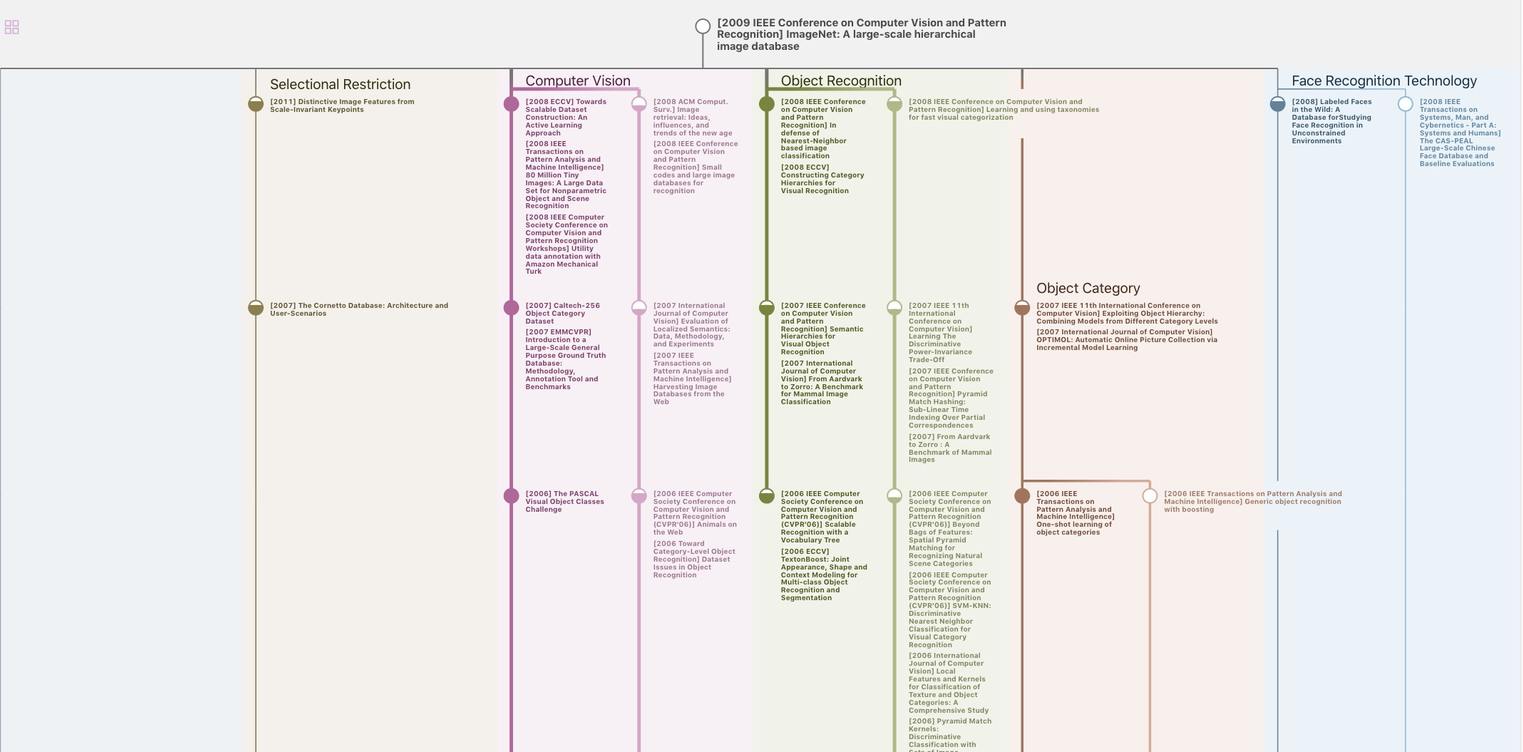
生成溯源树,研究论文发展脉络
Chat Paper
正在生成论文摘要