Using error decay prediction to overcome practical issues of deep active learning for named entity recognition
MACHINE LEARNING(2020)
摘要
Existing deep active learning algorithms achieve impressive sampling efficiency on natural language processing tasks. However, they exhibit several weaknesses in practice, including (a) inability to use uncertainty sampling with black-box models, (b) lack of robustness to labeling noise, and (c) lack of transparency. In response, we propose a transparent batch active sampling framework by estimating the error decay curves of multiple feature-defined subsets of the data. Experiments on four named entity recognition (NER) tasks demonstrate that the proposed methods significantly outperform diversification-based methods for black-box NER taggers, and can make the sampling process more robust to labeling noise when combined with uncertainty-based methods. Furthermore, the analysis of experimental results sheds light on the weaknesses of different active sampling strategies, and when traditional uncertainty-based or diversification-based methods can be expected to work well.
更多查看译文
关键词
Active learning,Transparency,Robustness to labeling noise,Black-box models,Clustering,Named entity recognition
AI 理解论文
溯源树
样例
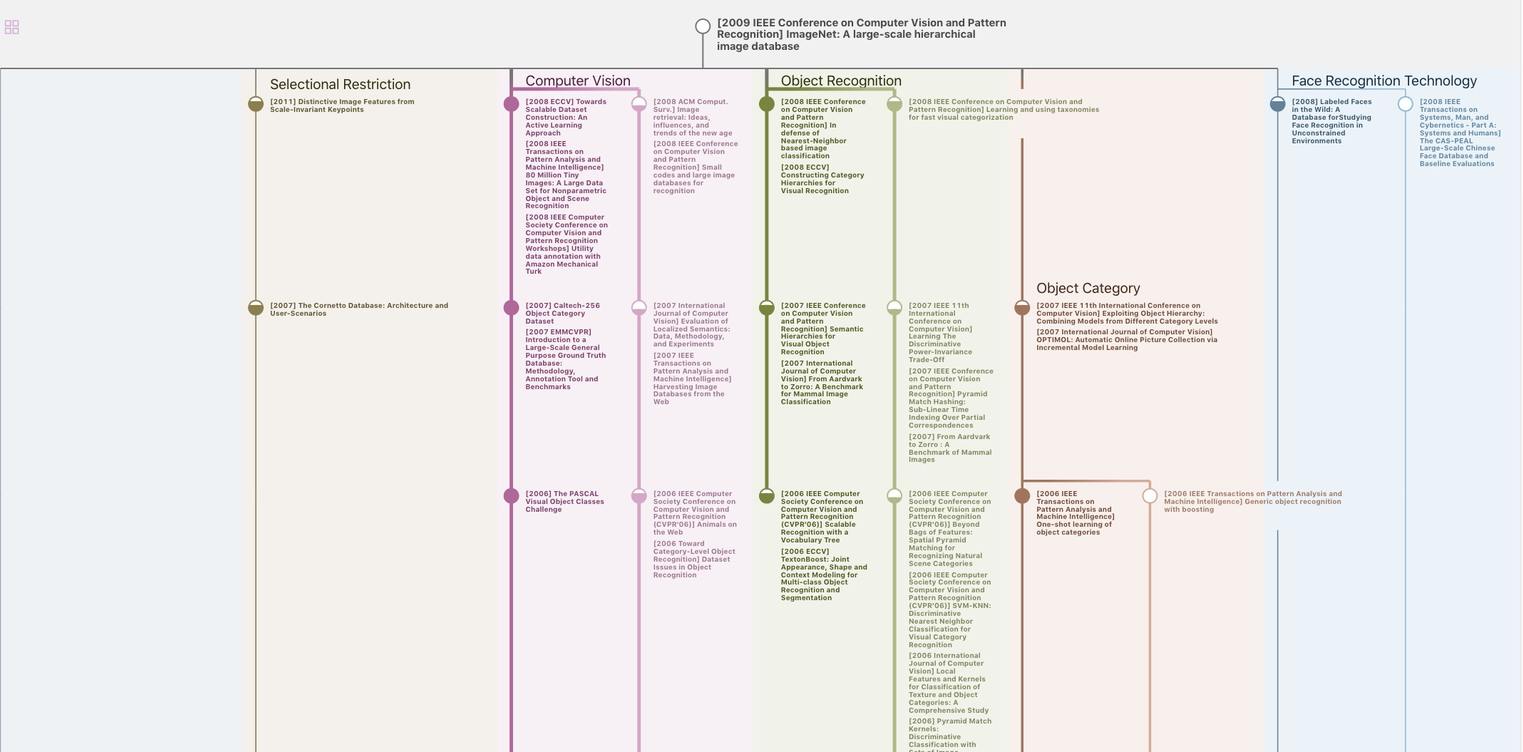
生成溯源树,研究论文发展脉络
Chat Paper
正在生成论文摘要