Near-Optimal A-B Testing.
MANAGEMENT SCIENCE(2020)
摘要
We consider the problem of A-B testing when the impact of the treatment is marred by a large number of covariates. Randomization can be highly inefficient in such settings, and thus we consider the problem of optimally allocating test subjects to either treatment with a view to maximizing the precision of our estimate of the treatment effect. Our main contribution is a tractable algorithm for this problem in the online setting, where subjects arrive, and must be assigned, sequentially, with covariates drawn from an elliptical distribution with finite second moment. We further characterize the gain in precision afforded by optimized allocations relative to randomized allocations, and show that this gain grows large as the number of covariates grows. Our dynamic optimization framework admits several generalizations that incorporate important operational constraints such as the consideration of selection bias, budgets on allocations, and endogenous stopping times. In a set of numerical experiments, we demonstrate that our method simultaneously offers better statistical efficiency and less selection bias than state-of-the-art competing biased coin designs.
更多查看译文
关键词
decision analysis: inference,dynamic programming,Markov,infinite state,statistics: design of experiments
AI 理解论文
溯源树
样例
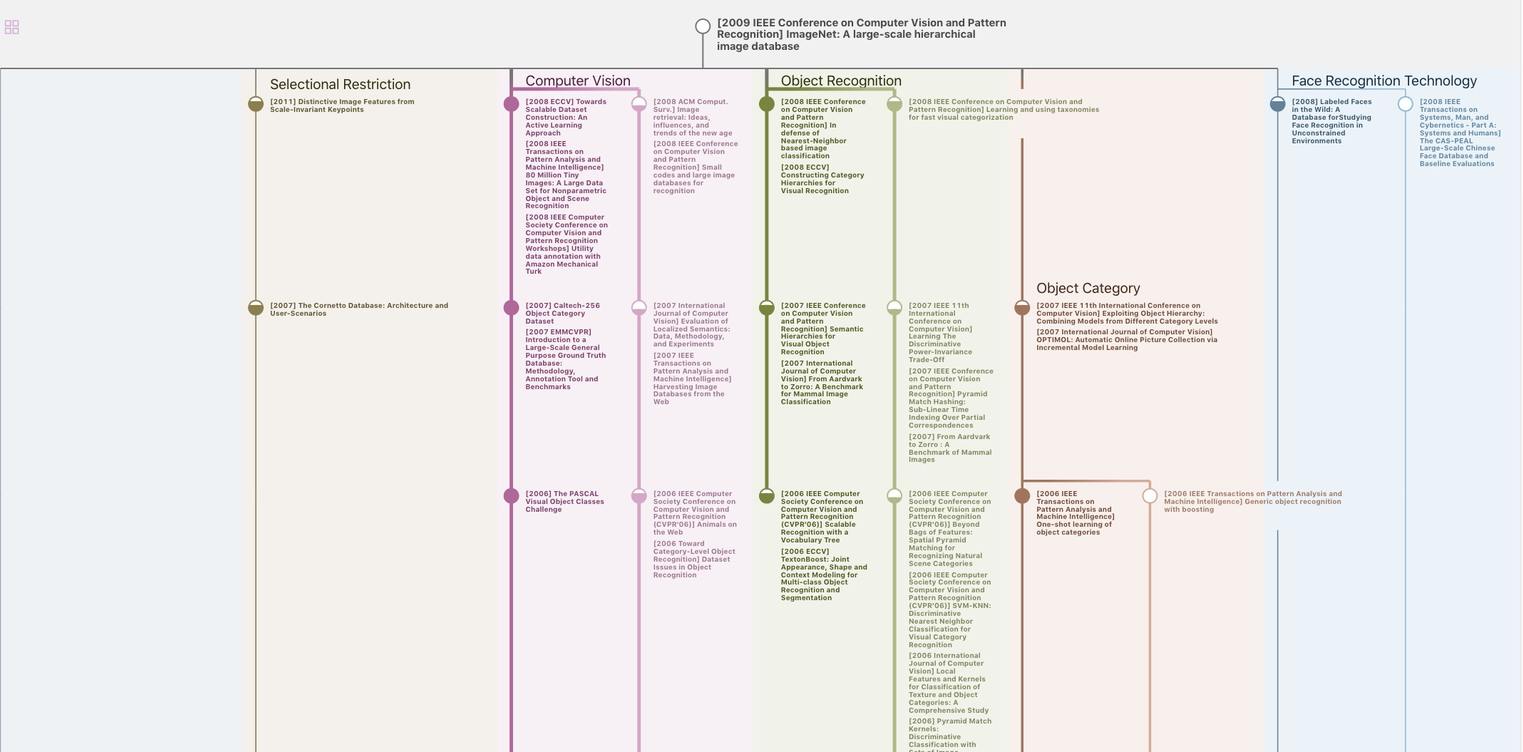
生成溯源树,研究论文发展脉络
Chat Paper
正在生成论文摘要